Who Owns and Controls Farming Data?
Introduction
The future of agriculture depends on the adoption of new technologies that gather, transfer, manage, and analyze data. New terms such as “smart farming” and “prescriptive agriculture” generally refer to the application of data-driven management in agriculture. Supported by sensors, the Internet of Things (IoT), and physical devices (e.g., smartphones) that collect and share data through the Internet, modern data collection has been relatively easier for farmers. In turn, data-driven methods, such as artificial intelligence (AI), have been gradually but significantly changing the decision-making of farming operations in terms of improved efficiency, efficacy, and profitability (Pham and Stack 2018; Khanna and Kaur 2019). However, there are many concerns that still have to be addressed before further advances and wider applications can be made. Farmers’ primary reservations about embracing data-driven technologies stem from the concern that farmers may lose ownership and control of their data from which Agriculture Technology Providers (ATPs) derive high-value products. ATPs are mainly the partners who develop or introduce the advanced technologies to help farmers better manage their farming operations. For example, a farmer may employ an ATP to quickly scout his 100-acre-farm by flying a drone over and taking RGB or multispectral images.
We intend to clarify the nature of farmers’ concerns in collaborating with ATPs in two parts: 1) the components of farming data, and 2) the principles and guidelines to follow when handling data ownership concerns in a smart farming partnership with ATPs.
The target audience of these publications includes Extension educators, farmers, smart farming entrepreneurs, and ATPs. Our primary goal is to clarify the concerns regarding data ownership and explain the responsibilities of that ownership, sharing, and benefits in a collaborative smart farming application.
What is farming data?
Farming data can be generated and collected through various processes and devices during food production. Farming data can be categorized by its objectives, including environment, agriculture operation, and system management. These objectives will be used to identify and further categorize the processes and devices through which the data was collected, because these are the most important features related to ownership of the data. Specifically, data is attributed to the land where it was collected, the device by which it was measured, the farmer who arranged the operation rate and sequence, the cloud service where it was stored, etc. All entities related to these attributes may claim the ownership of data in contracts. The farming data with ownership concerns is mainly raw data that feeds calculations, or decision-making. Therefore, in the ownership discussion, sophisticated measures or aggregations derived from raw data are not part of the scope. For example, the soil moisture data from a farm is considered raw information, while its average daily or weekly soil moisture is one of many aggregations. In this case, sophisticated measures may include the linkage of a certain soil moisture level with crop leaf color during a certain period analyzed by a software or algorithm.
Environmental Facts
Food production is highly subject to environmental features, such as soil, weather, and water resource conditions, which is why observations on such systems are widely applied in agriculture. Environmental observations are mostly objective facts, describing nature responses determined by physics, biology, chemistry, etc. With different collection methods and devices, these facts can be stored in various formats. Table 1 shows the representative features and associated examples.
Table 1. Environmental data.
The environment described here specifically refers to the open-field farming systems interacting with nature. For indoor agriculture, aquaculture, or other farming systems with intensive artificial environment controls, their environments are part of the operations decided by human or controlling programs.
Agriculture Operation Data
The management of agricultural operations is the primary focus of farmers’ daily work. For crops or livestock, various types of data are produced to describe the human activities and associated responses from the products. In turn, analysis, knowledge, and models can be conducted, produced, and developed by human or digital assistance (e.g., AI) to direct the operation executions, increase growth, and improve product yield, quality, and profit. In other words, this data is relevant to every aspect of operation of agricultural production cycles. People choose the timing, location, and operations to be undertaken. Nevertheless, detailed information about how operations were conducted, by which machine, at which rate at different locations, and based on what rules is also valuable. Therefore, many types of data are included in this category such as operation records, planting and yields, machine operation logs, etc. Table 2 shows the representative data types in this category.
Table 2. Agriculture operation data.
Business Data
Modern agriculture has transformed to be more like a business and is usually the top of a supply chain. It needs to fine-tune the usage and maintenance of its numerous facilities and devices to control cost while improving the yield and profit affected by various uncertainties during long growth cycles. Thus, farmers should endorse better analytical techniques to upgrade the efficiency and performance of farming operations. The representative data features in this category are shown in Table 3.
Table 3. Business data.
Experts estimated that the IoT would consist of almost 50 billion objects by 2020. With the expansion of the IoT used in agriculture for observation, measurement, and control, data related to devices may cover an even broader range of sensors (or “Things”).
Flow and Integration of Farming Data
Nevertheless, the three types of farming data previously mentioned will be always structured in a pyramid form (Figure 1). The data in the higher level is refined from the lower one. While business insights can be drawn from business data of power and water usage, yield, and quality, the improvement of the efficacy of operations is reflected by agriculture operation data which, in most cases, is the result of data-driven applications. For example, a smart irrigation schedule may be determined by estimating soil moisture and weather forecasts, estimating evapotranspiration, and simulating the photosynthesis of plants. These two types of farming data, business data and agricultural operation data, are to some extent generated by human creations that can be considered as intellectual property (IP). However, the concern of ownership is mainly for environmental facts, the bottom level with the largest size. To correctly and accurately depict the physical concepts or uncertainties on which agriculture operation decisions can be made, one must work with objective environmental facts. The environmental facts lack the innovation or creation by human mind to be considered as IP; nevertheless, such underlying concepts or uncertainties are the gold that AI and other data-driven applications are intended to mine from the environmental facts.
With the rapid development of AI, farming data, especially environmental facts, may potentially be of greater economic value than revenue from farming yields. Therefore, if the ownership of farming data is not well regulated, the related benefits and profit of farmers, who are the originators of this data, can be significantly impaired.
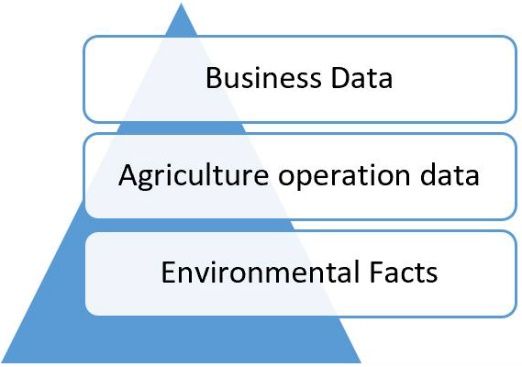
Credit: Ziwen Yu, UF/IFAS
Ownership and Control of Farming Data
Farmers’ Concerns
The results of a survey released by the American Farm Bureau Federation (AFBF) in 2014 (Figure 2) indicate that farmers and ranchers want to control the information collected from their fields and livestock. Most (81%) think they own their data and 77% of them are worried about data security. Ownership and control of farming data is a significant concern for farmers if others could use their information for commodity market speculation without their consent. A similar study in Australia by Wiseman et al. (2019) shows the majority (74%) of respondents did not know much about the conditions and terms in the contracts they signed. There is a similar lack of awareness surrounding data sharing with a third party, which usually requires the farmer’s approval.
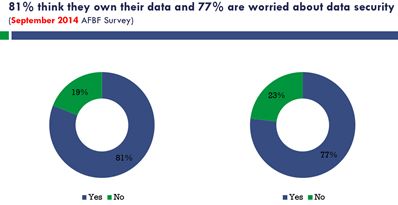
Credit: American Farm Bureau Federation. Used with permission.
While some ATPs offer to subsidize the service fee for owning on-farm data, competitors or downstream companies may take advantage and use the data to leverage more significant contractual terms against growers. Not surprisingly, these scenarios lead to concerns about how the data was collected from the fields and who owns and has access to such data. Regulation of such data is still nascent and court cases about OAU are sparse, adding to legal uncertainty and making business planning for growers more difficult because of information asymmetries regarding data-related risks, especially with the development of IoT in smart devices, such as tractors, drones, and smartphones.
Ownership and Control
Farming data produced by monitoring systems may involve many parties including producers, landlords, and different ATPs. Proportionally, everyone contributes to the processes in producing the physical agricultural commodities whose ownership is similar to owning a house, land, or a car. However, farming data generated during this process is a virtual product that cannot be easily considered as a common property. Instead, US law classifies data, including farming data, as “facts.” As the basic fact underlying certain agriculture commodities, farming data lacks a creative element which can be defined as an intellectual property (IP) whose ownership could be protected by copyright laws (17 U.S.C. § 102(a)), such as published books or commercial programs. Even if the data collection or the fact discovery has taken years in a research project, for example, the factual data is still not protected. Therefore, legally speaking, farmers do not own the “raw” data generated from their land.
However, creativity can be part of the arrangement, management, and selection of data that can be defined as IP. For example, a database can be protected under copyright laws if it compiles selective datasets collected from a certain field in 2020 for strawberry phenotyping. In other words, if a farmer stores and manages the data generated from their land and relates it to other elements, then their ownership of such a database can be copyrighted.
Unfortunately, most farmers lack such expertise or resources and have to purchase services from ATPs. This is the exact source of the farmers’ concerns about losing their ownership of data. The IP is owned by ATPs who made creations in collecting, managing, and analyzing data while farmers are the providers of the factual information. How can farmers’ benefits be protected in collaborations with ATPs?
This flaw has been handled by copyright law in which contracts to override ownership provisions are allowed. While the use of databases (e.g., managed datasets) is governed by copyrights, contract law can help regulate factual data. It is important for farmers to look more closely at the contracts through which both the data contributors (e.g., farmers) and the service providers (e.g., ATPs) bilaterally negotiate an agreement. These contracts are the primary legal documents that determine how agricultural data is owned, controlled, and shared.
Policies and Principles
Knowing farming data ownership is primarily governed by contract, farmers may still face unbalanced negotiations because many ATPs are large multinational corporations with powerful legal teams (Jakku et al. 2019). Nevertheless, negotiating asymmetries may be mitigated. This could be one of the motivations for the American Farm Bureau Federation to develop their Privacy and Security Principles in 2014 (American Farm Bureau Federation 2016) as a way to help farmers. A hypothetical smart farming case (Figure 3) will be used to explain the following principles. In Figure 3, the farmer has committed into a smart irrigation partnership with ATP1, ATP2, and ATP3. The arrows indicate the flow of information and activities. ATP1 provides the smart irrigation service to the farm with package (PK) 1 and 2. IoT smart sensors are provided by ATP1 to collect data (e.g., soil moisture) from the farm. Depending on the PK the farmer selects, the data will either be sent to the irrigation controller for simple judgment (PK1) or to other ATPs for sophisticated estimation (PK2). In PK2, data is first sent to ATP2, which provides cloud service to store and manage data. ATP3 will access the data in ATP2 to determine the irrigation schedule for the farm based on other information such as weather forecast and send the control signal to the irrigation system on the farm. ATP4 is an AI startup trying to develop new products in agriculture. This farm is also collaborating with ATP5, a smart fertilization company.
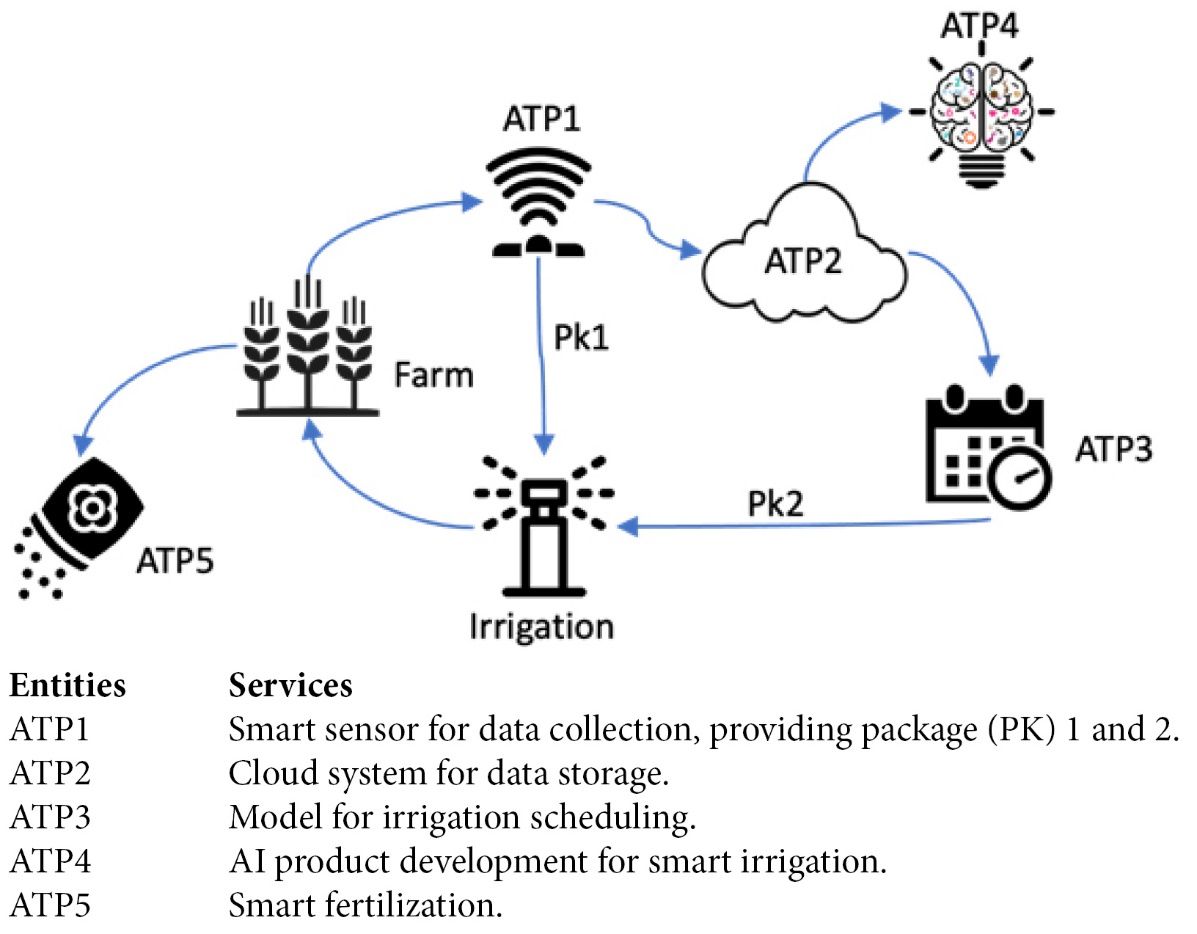
Credit: Ziwen Yu, UF/IFAS
- Education: Resources, courses, and Extension workshops need to be provided to help farmers learn more about the data collection processes on their farms. For the case in Figure 3, farmers need to know the flow of data directed by the arrows and the data-related activities each entity will conduct.
- Ownership: It is agreed that farmers retain ownership of the information generated on their farms. However, when uploading data to ATP portals, it is the farmers’ responsibility to determine whether the ATP owns this data or has permission to access. In the hypothetical case, farmers need to confirm that the permission to access data has been granted to ATP1, ATP2 and ATP3, which are involved in the smart irrigation partnership.
- Collection, Access, and Control: These are the fundamental actions surrounding data, the rights of which should be explicitly described in the contracts between farmers and ATPs. For example, in Figure 3, ATP1 collects data, ATP2 stores data, and ATP3 accesses data. However, the data flowing to ATP4 should be controlled by the owner of the data because ATP4 is not in the partnership.
- Notice: The contract for the partnership should clearly state who will collect, control, or access the data, as well as why, how, and when.
- Transparency and Consistency: Contracts should be written in a clear, concise, and consistent manner to ensure full comprehension. This could be an extension to the above principle (“Notice”) describing the reasons for the data collection and use in detail and explicitly stating the operators.
- Choice: The explanations of farmers’ choices in purchasing different kinds of services, usually additional packages, offered by ATPs are important. In Figure 3, ATP1 needs to clarify the difference between PK1 and PK2, especially the data flow.
- Portability: Farmers should have the right and ability to use their data across different applications for more opportunities and improvement of data values. In Figure 3, farmers can access the data in ATP2 and provide it to ATP5 for fertilization decision-making.
- Terms and Definitions: Farmers and ATPs should clearly understand the definitions of all the terms of their contracts.
- Disclosure, Use, and Sale Limitation: Farmers’ agreement is required for the sharing or sale of the original farming data to a third party. In Figure 3, ATP4 is trying to purchase the data from ATP2, including the data from the specific farm. Because ATP4 is not involved in the partnership, this disclosure and transaction cannot proceed without the agreement of the farmer.
- Data Retention and Availability: The length of the existence of farming data in an ATP’s system needs to be defined clearly, as well as how the data will be treated afterward (i.e., removed, returned, or destroyed). For example, in Figure 3, famers can have contractual terms specifying that ATP2 will only store data from the most recent two months and completely remove the old data beyond this period.
- Contract Termination: The contract should state what will happen to the data if an agreement is canceled.
- Unlawful or Anti-Competitive Activities: A contract should state that the ATP cannot use the data for unlawful or anti-competitive activities. For example, if ATP3 uses the irrigation data of the region where the farm is located to determine a fixed price of water with a utility company, this is an anti-competitive activity that should be prohibited in the contract.
- Liability and Security Safeguards: If an ATP stores farming data, it should have the proper protocols to protect this data from security breaches. ATP data security responsibilities should be clearly stated in the contract.
Conclusion
Farmers who have been using or are looking forward to applying smart farming technologies should fully understand the principles listed above when working with ATPs. It is also important for every farmer to do the following.
- Realize that smart farming applications are not only tools to help farming operations, but also an industry node to which farmers contribute their farming data. In the Figure 3 example, when disclosing data to ATP4, farmers can request a share of the profit of the ATP’s future products that will be developed using the data provided by farmers.
- Be knowledgeable about the farming data flow and the relationship between farmers and ATPs.
- Know the terms and associated meanings so that they can completely understand the contract and the explicitly defined ownership, access, and control.
- Resolve confusion before committing to any data sharing or service.
- Learn basic techniques about data management and keep a personal copy of the farming data from their farms.
- Apply smart farming applications using farming data to improve the efficiency of farming operations.
References
American Farm Bureau Federation. 2016. "Privacy and Security Principles for Farm Data." Retrieved July 9, 2021. https://www.agdatatransparent.com/principles
Jakku, E., B. Taylor, A. Fleming, C. Mason, S. Fielke, C. Sounness, and P. Thorburn. 2019. "'If they don’t tell us what they do with it, why would we trust them?' Trust, Transparency and Benefit-Sharing in Smart Farming." NJAS—Wageningen Journal of Life Sciences 90-91:100285.
Khanna, A., and S. Kaur. 2019. "Evolution of Internet of Things (IoT) and Its Significant Impact in the Field of Precision Agriculture." Computers and Electronics in Agriculture 157:218–231.
Pham, X., and M. Stack. 2018. "How Data Analytics Is Transforming Agriculture." Business Horizons 61(1): 125–133.
Wiseman, L., J. Sanderson, A. Zhang, and E. Jakku. 2019. "Farmers and Their Data: An Examination of Farmers’ Reluctance to Share Their Data through the Lens of the Laws Impacting Smart Farming." NJAS—Wageningen Journal of Life Sciences 90-91:100301.