With the increasing use of unmanned aerial systems (UASs) in the agricultural domain, ensuring the consistency and completeness of aerial surveys is critical in order to establish repeatability and consistency in data collection activities. While the operational use of UASs has been simplified due to advances in user-friendly technologies in the past few years, operational issues concerning data collection and/or data delivery protocols remain unclear. Ensuring consistency in the process of aerial data collection and processing guarantees that studies conducted using aerial data continue to be relevant and repeatable in the future. This document is intended to help with setting basic protocols for collecting UAS data critical to time-sensitive observations.
This document covers five main steps to ensure that aerial data collections are repeatable and consistent among missions: 1) establishing survey areas and demarcating focus zones, 2) setting geo-reference points in and around the sampling area, 3) flight planning and conducting the actual survey, 4) image stitching and orthorectification, and 5) post-processing imagery to ensure consistent alignment. This publication is one of a three-part series focusing on the applications, configuration, and best practices for using UASs in agricultural operations management.
Introduction
With the advent of newer optical, thermal, and laser sensors, the use of small UASs has grown exponentially in the past few years (van der Wal et al. 2013; Laliberte, Winters, and Rango 2011; Verger et al. 2014; Rasmussen et al. 2013). Applications of UAS-borne sensors include crop acreage estimation (Atkins 2014), crop progress assessment (Geipel, Link, and Claupein 2014), evaluation of efficiencies of fertilizer and/or pesticide applications (Ladd and Bland 2009), disease detection (Mahlein 2016; Kerkech, Hafiane, and Canals 2020), and cultivar evaluations (Gracia-Romero et al. 2019).
Single-date (or single-pass) surveys are useful for establishing baseline conditions in farms (e.g., estimating acreage, developing digital surface models [terrain models], or obtaining snapshot views of crop condition). The most powerful applications of aerial imaging techniques are for monitoring crop progress over time. When conducted consistently, UAS-based surveys allow the observation of crop progress, phenology, and impacts of fertilization or pesticides. These surveys also help map and assess spatial patterns of yield variability by observing combinations of crop health indicators over time. For repetitive surveys to be effective, however, they should be conducted with established protocols that maintain data consistency across space and time.
This document discusses a few best management practices to help practitioners ensure that aerial surveys are repeatable and replicable when consistent monitoring is required. The publication outlines typical sequences of steps to follow when designing and executing aerial surveys for agricultural operations.
Establish the survey area and demarcate study zones.
- Establish the boundaries of the survey site in consultation with the stakeholder or property owner. Make sure to consult with a person who holds a current Remote Pilot License to ensure UAS flight permissions have been secured. See the EDIS document by Kakarla and Ampatzidis (2018) (https://edis.ifas.ufl.edu/ae527) for an overview of the licensing and preflight check processes.
- Have the area physically surveyed and the survey map converted to a standard geospatial format usable in a GIS framework (typically as a "shapefile"). The survey should preferably be conducted using differential GPS survey instrumentation to preserve the accuracy of the area being mapped.
- Depending on the context of the survey, divide the survey areas into treatment or cropping zones per the study design or farm management or planting plan (Figure 1). These zones may denote different crops, cultivars, nutrient treatment or irrigation zones, or other demarcations as applicable in the context. It is important that the subdivisions be as fine-grained as possible. While data can be averaged across treatments or blocks after the survey, the data cannot be disaggregated within zones on a later date. Assign a unique ID to each of the zones in the shapefile and store in a separate filing system for future reference.
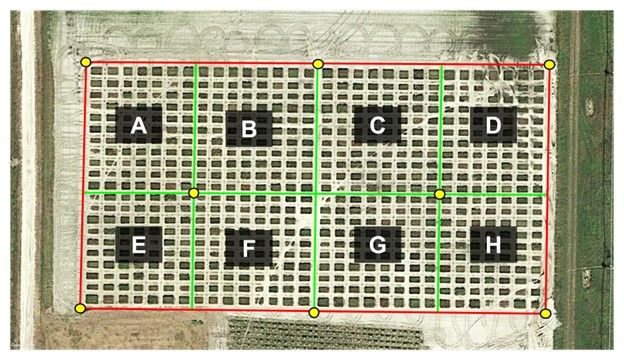
Credit: Google EarthTM
Establish geo-reference points in and around the sampling area.
- The GPS units on commercial off-the-shelf (COTS) UASs have a positional accuracy of approximately 3–5 m. Imagery collected? using these GPS units will typically display slight offsets after image stitching and orthorectification. These offsets may be of concern when repeat flights are required to track the progress of the crop or to detect changes in crop health after treatments. This is because regions mapped in successive flights may not overlap spatially if the GPS does not support sub-meter accuracies that are available from advanced Real-Time Kinematic (RTK)- or Post-Processing Kinematic (PPK)-based surveys. RTK- or PPK-based systems use two GPS units in tandem: a "base" GPS unit in a fixed location (e.g., at the UAS operator's location), and a "rover" unit mounted on the UAS that communicates with the base unit as the UAS flies and supplies real-time data that are used to refine location information. Using RTK/PPK technologies can allow the geolocation error to be reduced to less than 5 cm. When RTK/PPK-based systems are not available, a network of locations that can be used to correct the geolocation of imagery post-mission is required.
- Find a minimum of 10–20 locations across that field that are either invariant (do not change or move over time, i.e., corners of built surfaces such as parking lots or road intersections), or can be marked semi-permanently using a metal stake or PVC pipe without causing interference to farm operations (see Figure 1 for an example with eight locations). Note that more locations may be required if the shape of the plot is complex or if the site is topographically uneven. Conversely, if the area is small (e.g., less than 5 acres) and relatively flat and has several strong markers as described above, as few as five locations (one at each corner and one in the center) may suffice.
- Mark all locations established in the previous step by either driving a metal stake (e.g., rebar) or a PVC pipe into the ground. Record and file the location of these markers using a differential GPS unit. Once locations have been established, fashion a circular target (PVC, metal, or plywood painted black and white) with prominent markings (Figure 2) that can be placed at each location before commencing flight operations. This way, the geolocation targets will be imaged consistently for every mission and will allow the spatial adjustment of subsequent images to base location in a consistent manner. If needed, remove the markers at the end of each campaign, and replace before every flight.
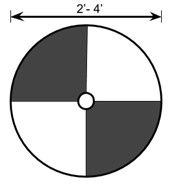
Credit: Aditya Singh, UF/IFAS
Flight Planning and Conducting the Actual Survey
Flight planning can be considered as an extension of efforts to keep data collection as consistent as possible between missions. Follow the steps below to increase the efficiency of aerial data collection efforts.
- Flight Planning: Plan flights using an automated software or app. Many freely available or commercial apps, such as DroneDeploy®, DJI Go®, MissionPlanner®, and U-GCS®, allow the user to store flight plans before flying. When conducting multiple aerial surveys, the same flight plan should be used as often as possible unless the ground situation or survey area changes. If using standard flight planning apps, design the flight paths to align with the longest edge of the field when possible (Figure 3). While diagonal and short edge-aligned flight paths are possible alternatives, they generally force slowing the UAS around the ends and cause battery drain.
- Flight Timing: Flights should be conducted as close to the solar noon (i.e., the time the sun is directly overhead) as possible to avoid large variations in solar angles during the mission. Having the sun directly above the aircraft minimizes shadowing artifacts and helps minimize brightness differences between missions. Ideally, missions should be flown when the sky is completely overcast, and the effects of canopy self-shadowing are minimized. However, these conditions can rarely be expected on a consistent basis. The most suitable alternative is to fly during sunny days with little or no cloud cover that may cast shadows during the mission. Note that these are general recommendations. Certain applications may require flights to be conducted under specific conditions. For example, thermal imagery for estimating plant water use is best collected in sunny conditions when plants are actively transpiring. Conversely, optical imagery over complex canopies is best collected in overcast conditions to avoid canopy-shadowing artifacts.
- Cloud Shadows: It is often assumed that cloud shadows can be ignored when the intention of the aerial survey is to obtain image indices synthesized from image bands (such as the often-used NDVI) (Rouse et al. 1974). The assumption that image indices are invariant to effects of terrain and/or cloud shadowing may be invalid in many circumstances. This is because varying light wavelengths are scattered differently depending on the proportional mix of direct and diffuse incident radiation at the time of sampling. Shaded regions may produce erroneous estimates of image indices and end up confounding findings. Newer vegetation indices such as the shadow-eliminated vegetation index (SEVI) (Jiang et al. 2019) may be a useful alternative if pre-planning activities cannot eliminate shadows.
- This is not critical, but if the temporal frequency or observation schedule allows, it would be advantageous to follow the ephemeris of satellite overpasses. This allows aerial data to be validated against satellite imagery when cloud conditions permit. The satellite overpass predictor provided by NASA (https://cloudsway2.larc.nasa.gov/cgi-bin/predict/predict.cgi) can be used to generate observation schedules.
Image Stitching and Orthorectification
The image stitching and orthorectification processes are, in general, software specific. However, the process is consistent across all image-stitching programs. All software programs need images to be organized in folders for processing. Typically, the software reads image metadata on location, timing, and relative orientations, then aligns the pictures in Cartesian space according to approximate locations. The building of depth maps from features detected among overlapping pictures occurs next, followed by the generation of a point cloud. A digital surface model is then created from the point cloud, and finally, the feature map is stitched into an orthorectified image. Kakarla and Ampatzidis (2019) detail steps involved in this process when using the Pix4D® system (https://edis.ifas.ufl.edu/ae533).
Post-Processing Imagery to Ensure Consistent Alignment
Once all imagery has been stitched and orthorectified, it should be further geo-rectified to either a base image (e.g., digital orthophoto quadrangle, DOQ, or digital topographical map), or when a base image is not available, relatively geo-rectified using one of the orthorectified images as a "base" reference. In general, the process involves image-to-image registration and can be achieved using the open-source GIS platform QGIS (https://www.qgistutorials.com/en/docs/3/advanced_georeferencing.html) or commercial platforms such as ArcGIS™. The basic process involves picking common reference points between a base image (see the example in the QGIS tutorial) and the target image (the aerial image). The user selects a series of locations on the base image and matches them with corresponding locations on the target image. The software then uses these pairs of locations to adjust the target image to fit as closely to the base image as possible. Eventually, this newly geo-registered image can be used as the base image to georeference all images acquired over this site.
Conclusion
This document serves as a blueprint for designing and conducting UAS surveys specifically for agricultural applications. While a single-date aerial survey may suffice for cartographic or acreage estimation purposes, the power of UAS imagery for agricultural operations management generally lies in observing how plants develop over time, and how they respond to irrigation, fertilization, or pesticide applications. This document helps to set the basic protocols for collecting data that might be critical to time-sensitive observations. Note that certain modifications may be necessary to address specific application needs. For example, the time of aerial data collection may be a critical factor if one intends to use thermography data to estimate plant water use because plants utilize water differently throughout the course of a day. Readers may contact the authors or other UF/IFAS remote sensing faculty to request help with planning efficient aerial surveys.
References
Atkins, E. 2014. "Autonomy as an Enabler of Economically-Viable, beyond-Line-of-Sight, Low-Altitude UAS Applications with Acceptable Risk." AUVSI Unmanned Systems. https://www.academia.edu/14400490/AUTONOMY_AS_AN_ENABLER_OF_ECONOMICALLY_VIABLE_BEYOND_LINE_OF_SIGHT_LOW_ALTITUDE_UAS_APPLICATIONS_WITH_ACCEPTABLE_RISK
Geipel, J., J. Link, and W. Claupein. 2014. "Combined Spectral and Spatial Modeling of Corn Yield Based on Aerial Images and Crop Surface Models Acquired with an Unmanned Aircraft System." Remote Sensing 6 (11): 10335–55. https://doi.org/10.3390/rs61110335
Gracia-Romero, A., S. C. Kefauver, J. A. Fernandez-Gallego, O. Vergara-Díaz, M. T. Nieto-Taladriz, and J. L. Araus. 2019. "UAV and Ground Image-Based Phenotyping: A Proof of Concept with Durum Wheat." Remote Sensing 11 (10): 1244.
Jiang, H., S. Wang, X. Cao, C. Yang, Z. Zhang, and X. Wang. 2019. "A Shadow-Eliminated Vegetation Index (SEVI) for Removal of Self and Cast Shadow Effects on Vegetation in Rugged Terrains." International Journal of Digital Earth 12 (9): 1013–29. https://doi.org/10.1080/17538947.2018.1495770
Kakarla, S. C., and Y. Ampatzidis. 2018. Instructions on the Use of Unmanned Aerial Vehicles (UAVs). AE527. Gainesville: University of Florida Institute of Food and Agricultural Sciences. https://edis.ifas.ufl.edu/ae527
Kakarla, S. C., and Y. Ampatzidis. 2019. Postflight Data Processing Instructions on the Use of Unmanned Aerial Vehicles (UAVs) for Agricultural Applications. AE533. Gainesville: University of Florida Institute of Food and Agricultural Sciences. https://edis.ifas.ufl.edu/ae533
Kerkech, M., A. Hafiane, and R. Canals. 2020. "Vine Disease Detection in UAV Multispectral Images Using Optimized Image Registration and Deep Learning Segmentation Approach." Computers and Electronics in Agriculture 174 (July): 105446.
Ladd, G., and G. Bland. 2009. "Non-Military Applications for Small UAS Platforms." In AIAA Infotech@Aerospace Conference. Infotech@Aerospace Conferences. American Institute of Aeronautics and Astronautics. https://doi.org/10.2514/6.2009-2046
Laliberte, A. S., C. Winters, and A. Rango. 2011. "UAS Remote Sensing Missions for Rangeland Applications." Geocarto International 26 (2): 141–56. https://doi.org/10.1080/10106049.2010.534557
Mahlein, A.-K. 2016. "Plant Disease Detection by Imaging Sensors—Parallels and Specific Demands for Precision Agriculture and Plant Phenotyping." Plant Disease 100 (2): 241–51.
Rasmussen, J., J. Nielsen, F. Garcia-Ruiz, S. Christensen, and J. C. Streibig. 2013. "Potential Uses of Small Unmanned Aircraft Systems (UAS) in Weed Research." Weed Research 53 (4): 242–48. https://doi.org/10.1111/wre.12026
Rouse, J. W., Jr., R. H. Haas, J. A. Schell, and D. W. Deering. 1974. "Monitoring Vegetation Systems in the Great Plains with ERTS." https://ntrs.nasa.gov/search.jsp?R=19740022614
van der Wal, T., B. Abma, A. Viguria, E. Prévinaire, P. J. Zarco-Tejada, P. Serruys, E. van Valkengoed, and P. van der Voet. 2013. "Fieldcopter: Unmanned Aerial Systems for Crop Monitoring Services." In Precision Agriculture '13. 169–75. Wageningen Academic Publishers. https://doi.org/10.3920/978-90-8686-778-3_19. https://link.springer.com/chapter/10.3920/978-90-8686-778-3_19
Verger, A., N. Vigneau, C. Chéron, J.-M. Gilliot, A. Comar, and F. Baret. 2014. "Green Area Index from an Unmanned Aerial System over Wheat and Rapeseed Crops." Remote Sensing of Environment 152 (September): 654–64. https://doi.org/10.1016/j.rse.2014.06.006