Introduction
The main aim of this publication is to discuss the concept of variable rate technology (VRT), and its components associated with variable rate application of water, fertilizer, and other agricultural inputs. This publication also provides an example of the control system for variable rate application of agricultural inputs in row and tree crops. The document provides useful information on VRT to students, research scientists, Extension agents, growers, agricultural consultants, and state agency personnel.
Variable Rate Technology
In traditional agriculture, the same amount of agricultural input is applied across the field regardless of within-field variability, such as topography, variation in soil type, texture, or organic matter content, etc. This “one size fits all” approach to applying inputs may lead to either under- or over-applications of inputs, and consequently, variations in yield across the field, but it further impacts environmental sustainability and farm economics (Lark and Stafford 1997).
In the last two decades, successful advancements in precision agriculture technologies such as VRT have enabled the site-specific (i.e., individual plant or management unit) application of water, fertilizer, and other inputs such as herbicides or pesticides to manage spatial and temporal variabilities in yields within agricultural fields. According to the USDA Agricultural Resource Management Survey, the current adoption rate of VRT in the United States has increased by 69% across major commodity crops (corn: 71%, soybean: 76%, cotton: 74%, winter wheat: 68%, and sorghum: 57%) (USDA 2023). Survey results from 2016–2019 also indicated the increase in the use of VRT from 3.9% to 8.6% of crop planted acres in pesticide application, 9% to 25.3% of crop planted acres in seeding rate, and 8% to 28.2% of planted acres in fertilizers/lime application. Recent developments in artificial intelligence (AI) and sensor technologies have boosted adoption of VRT in the U.S. and worldwide.
The VRT enables growers and their crop advisors to precisely apply the agricultural inputs (such as water, nutrients, chemicals, etc.) at variable rates in response to the spatial variability within the field. This can be achieved by integrating VRT into different farming practices like seeding, irrigation, fertilization, and pesticide/fungicide application. VRT can be adopted into farming practices in two methods: (1) map-based (Figures 1, 2, and 3), and (2) sensor-based (Figure 4). When used appropriately, both techniques assist growers and other agricultural players to optimize crop yields, reduce input and labor costs, increase farm net revenue, and minimize environmental degradation (Grisso et al. 2011; Campos et al. 2020).
Map-Based VRT
Map-based VRT requires spatial data collection, and processing aimed at generating prescription maps using data from sensors (such as soil moisture sensors, soil electrical conductivity [EC] sensors, or drone/satellite sensors) as proxies of spatial variability of the parameter of interest. The prescription maps are then converted into a set of instructions that control the actual variable rate application system. The basic steps involved in the map-based VRT include:
- A process of investigating the field conditions — soil topography, soil types, soil properties, soil moisture variation, existing crop data, yield, biomass variation using soil sampling, remotely sensed images, or historical yield/biomass data.
- Generating the site-specific maps for the property of interest.
- Using a computer algorithm to develop the site-specific application map also known as a prescription map.
- Uploading the prescription map to the variable rate applicator to control the variable rate application of inputs.
Growers and their crop advisors can adopt different techniques, such as simple use of soil maps from Web Soil Survey (https://websoilsurvey.nrcs.usda.gov/app/), grid soil sampling, EC mapping (Veris Technologies), or remote sensing, to assess the field variability. Each of these methods has advantages and disadvantages and varies in cost. In grid soil sampling, the field is divided into different grids, and soil samples are collected from each grid and analyzed for desired soil property. For detailed information on grid soil sampling, refer to Ask IFAS publication SL 190, “UF/IFAS Nutrient Management Series: Soil Sampling Strategies for Precision Agriculture.” The Veris EC mapping can be used to understand a field’s soil texture and organic matter variability. However, these datasets are often collected at the point scale. For simplicity, constant values of the measured property (such as soil EC) are assigned to each sampling area or grid for variable rate application of agricultural inputs (Figure 1).
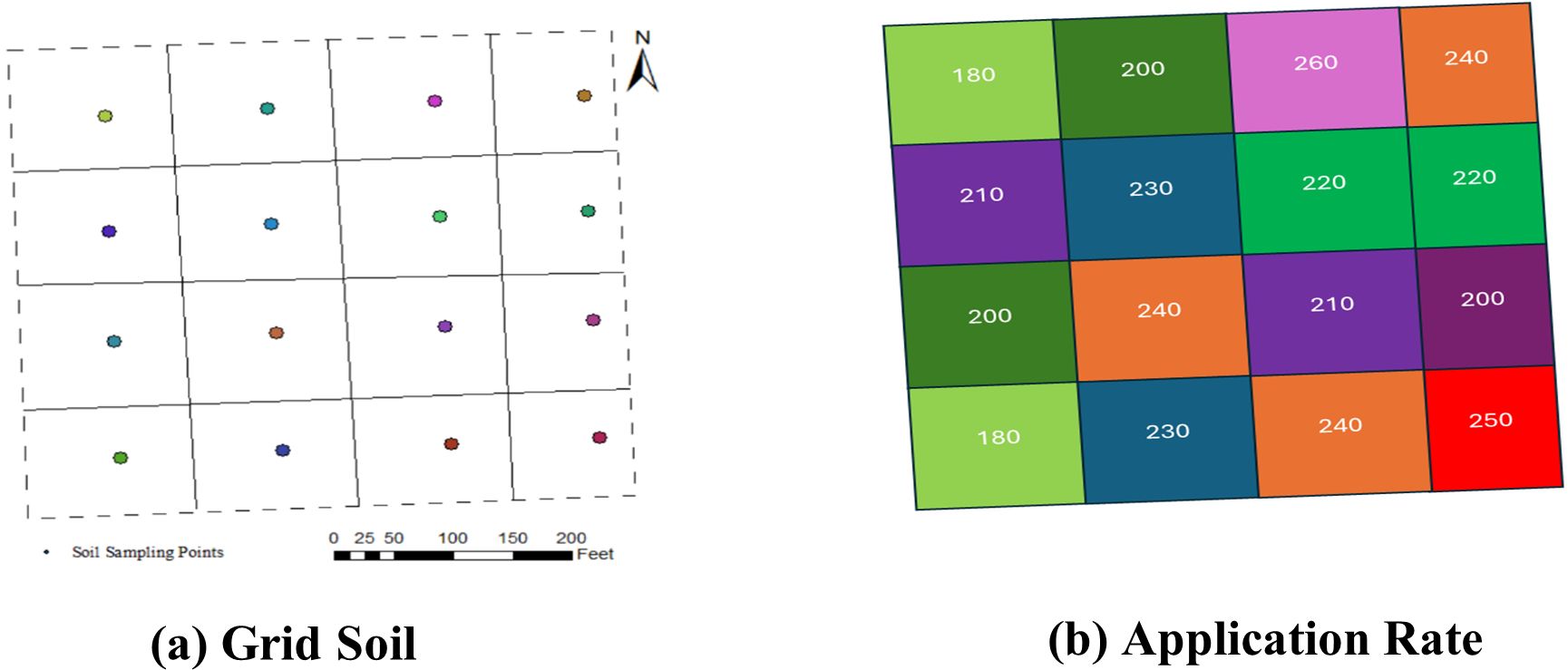
Credit: Vivek Sharma, UF/IFAS
Precise application of agricultural inputs requires a continuous, varying map at high resolution. Geographic Information System (GIS) interpolation techniques (Sharma et al. 2013) can be used to develop the continuous variability map (Figure 2). However, proper training is necessary to develop these maps through such techniques. Alternatively, multispectral images collected using drones or satellites can be used as proxies of parameters of interest (e.g., normalized difference vegetation index [NDVI] and other vegetation indices [VI]) to capture the within-field spatial variability (Figure 3). For more information on drone images, refer to Ask IFAS publication AE565, “Types of Unmanned Aerial Vehicles (UAVs), Sensing Technologies, and Software for Agricultural Applications.”
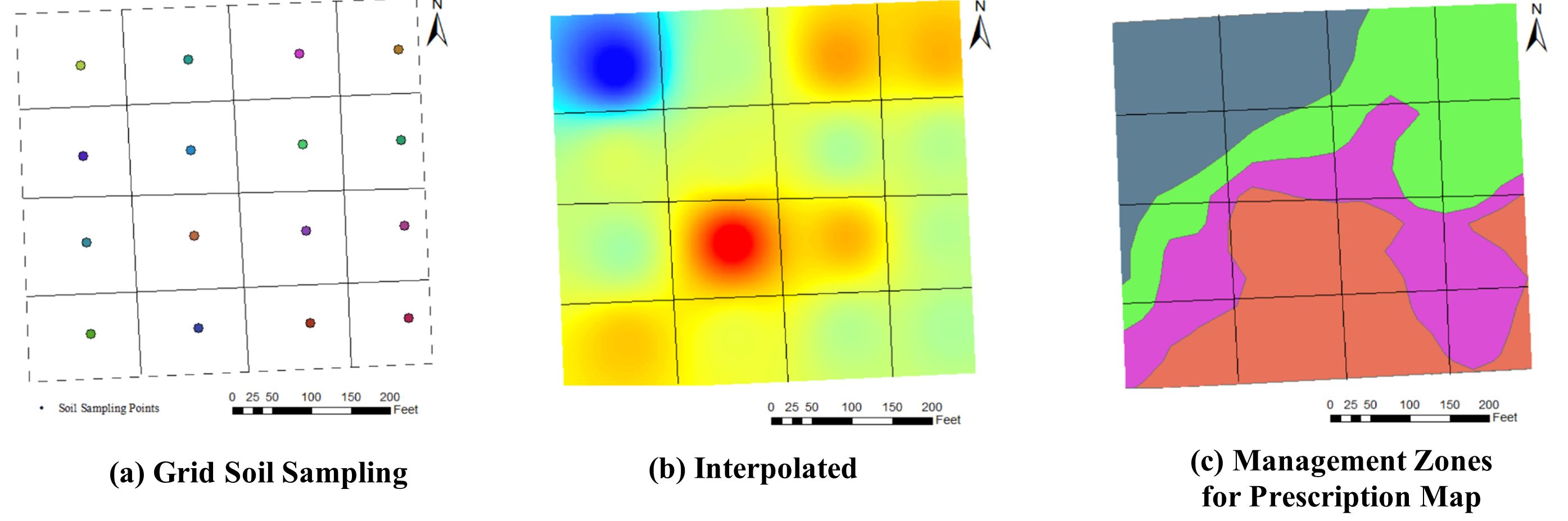
Credit: Vivek Sharma, UF/IFAS
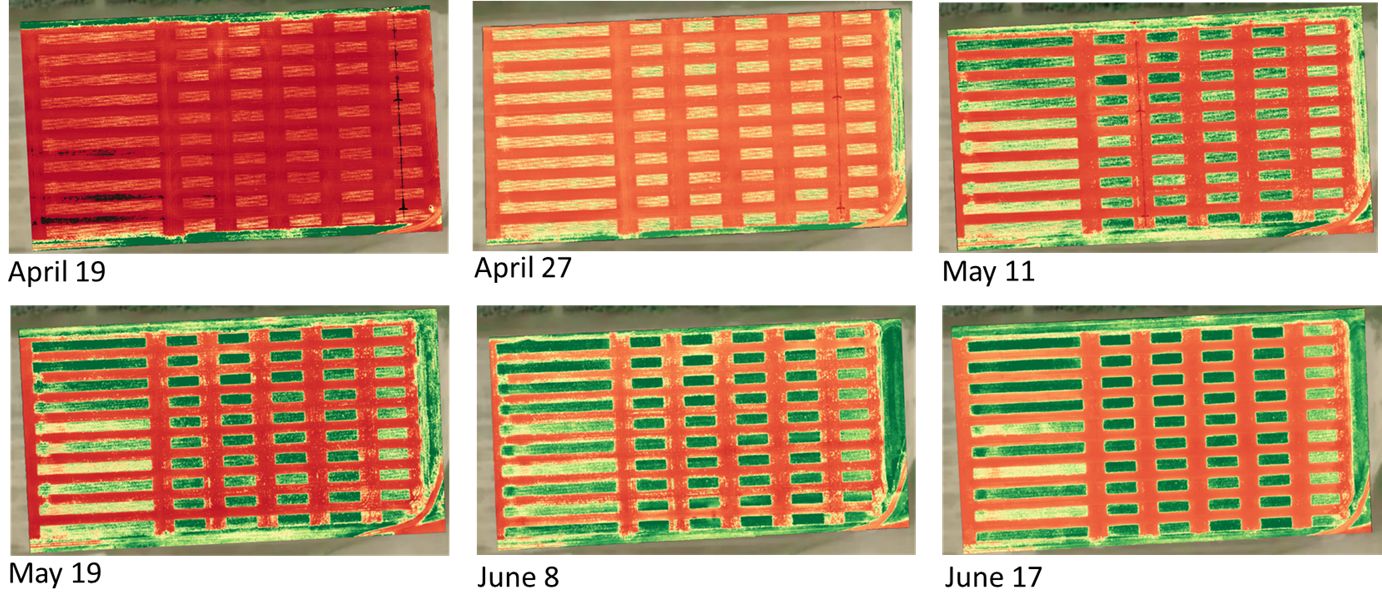
Credit: Vivek Sharma, UF/IFAS
Once maps of in-field variability have been generated, they are converted to prescription maps that will be read by variable rate applicators using different algorithms. These algorithms are based on standard irrigation and fertilizer recommendation formulas, such as water balance approach, canopy temperature-based irrigation scheduling, soil electrical conductivity (EC), and NDVI-based nitrogen management. These prescription maps generally contain the application rate based on the spatial variability in the field. Lastly, these prescription maps are transferred to the variable rate control panel system to deliver the proper rate of agricultural inputs at different locations in the field. In this whole process, a positioning system (e.g., Differential Global Positioning System [DGPS], which provides latitude and longitude information) is used continuously to identify the vehicle’s locations in the field.
Two types of prescription maps are generally used in the VRT: Static Prescription Maps (SPM) and Dynamic Prescription Maps (DPM) (O’Shaughnessy et al. 2013). As the name suggests, the SPM remains static throughout the growing season. These maps are generally based on parameters such as historical yield maps, soil texture, soil electrical conductivity, etc., and are often applied at the beginning of the crop season. However, many variables, such as irrigation and fertilizer requirements, are greatly affected by in-situ temperature and moisture conditions and fluctuate considerably within the growing season and between years. The DPM resulting from continuous monitoring and assessment of within-season field variability can be used for the dynamic prescription. Figure 3 represents the within-field and within-season variation of NDVI. These different maps can be used to develop DPM in conjunction with VRT to apply different amounts of agricultural inputs at different times within the growing season. Among the existing techniques, variable rate or site-specific irrigation (VRI) technology on a mechanical sprinkler irrigation system is an advanced irrigation management tool that uses map-based VRT and has the potential to optimize water use efficiency. VRI is a process in which VRT gets integrated with the pivot or lateral move irrigation system which lets farmers irrigate based on the variability of field conditions. Commercially, companies including Valley Irrigation (Valmont Industries, Inc., Omaha, NE), Zimmatic (Lindsay Corporation, Omaha, NE), and Reinke (Reinke Manufacturing Co., Inc., Deshler, NE) provide VRI technologies for both center pivot and lateral move irrigation systems. This technology enables farmers to change the irrigation rates in a zone or for individual sprinklers with respect to the field conditions. Developing the prescription map is one of the most efficient ways to adopt the VRT for irrigation.
Sensor-Based VRT
Another name for sensor-based VRT is on-the-go VRT. In contrast to map-based technology, sensor-based technology does not require prior collection of field conditions; rather, as the system advances, sensors built into it decide how much input to apply in the field. At the same time, sensor-based VRT enables a high density of sampling (e.g., at a high resolution of plant scale) which can help to precisely quantify the within-field variation. In sensor-based VRT, real-time measurements of soil properties and crop characteristics are made, processed, and used as a source of input in the variable rate applicator in real time. This method is mostly used for herbicides, pesticides, fungicides, and fertilizer applications. Table 1 provides a summary of the map-based and sensor-based VRT. The next section discusses the real-time application of sensor-based VRT in row crops and tree crops. In addition, a brief description is provided on the use of AI techniques in VRT.
Summary of map-based and sensor-based variable rate application:
Map-Based Variable Rate Application
- Most of the current site-specific variable rate technologies in different cropping systems utilize map-based VRT.
- Pre-data analysis by an expert user, including grid soil sampling, lab analysis, and generation of the prescription map, is required.
- Multispectral remote sensing images provide an alternative for the development of prescription maps.
- Differential Global Positioning System (DGPS) is used continuously to identify the different locations in the field.
- Technical and operational skills, including knowledge of software such as GIS, are required to generate prescription maps.
- The sampling size is based on the zones within the field.
- Travel speed needs to be altered during the application.
- Prior knowledge of agricultural inputs for the variable application is needed.
- There is a high cost associated with soil sampling and analysis.
Sensor-Based Variable Rate Application
- The adoption rate is increasing. More accurate in-situ sensing mechanisms are required to differentiate soil-plant-weed within the field.
- Pre-data analysis is not required. Field information is generally collected in real time for variable application of agricultural inputs.
- Multispectral remote sensing images are not required.
- DGPS is not required.
- Lab analysis of soil/plant samples is not required.
- Initial investment is high.
- The sampling size is the individual plant.
Application of Sensor-Driven Decision Support System
Over the years, many technological solutions and sensing mechanisms have been developed to synchronize the sensor's measurement with the desired application rate on-the-go. In general, the system consists of a sensing mechanism, a control interface, a display and control module, a GPS unit, and a variable rate applicator unit. The sensing mechanism is typically mounted in front of the application unit to provide the variable rate applicator enough time to adjust the rate before the applicator units pass the sensed location. A sensing mechanism must be installed directly over the target (crop, soil, weeds, etc.) facing downward. For effective data collection, it is advisable to mount the sensing mechanism 2.5 ft to 3.5 ft above the target.
Currently, most commercially available on-the-go sensing mechanisms are based on active light sensors. The sensors emit their own light on the target and measure the light reflected by the target. In most cases, these in-house light sources are unaffected by clouds, shadows, sunlight, and other interfering light sources. They enable use of these sensors in varying solar conditions, even in the night. This reflected signal is used to calculate certain properties of the target, which is further used in conjunction with an algorithm and variable rate unit to apply agricultural inputs variably.
Sensor-Based Fertilizer Applicator for Row Crops
Sensor-based variable rate fertilizer applicators have also been developed for row crops. One example of an active sensor that emits red and infrared light is the GreenSeeker sensor. It calculates the NDVI values based on the light the plant reflects. The sensor continues to sample the scanned area and provide an average NDVI reading (ranging from 0.00 to 0.99). The NDVI is based on the reflectance from plants in near-infrared (NIR) and red regions as follows:
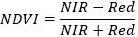
The NDVI generated by the sensor provides an input data source for the electronic circuit, which consists of a microcontroller system and transfers the data based on the sensor algorithm to a proportional solenoid valve. A proportional solenoid valve changes the liquid flow rate by varying the voltage provided for the valve by the microcontroller. A nozzle is attached to one end of the solenoid valve to dispense foliar spray. The complete system is mounted and operated by a tractor (Figure 4).
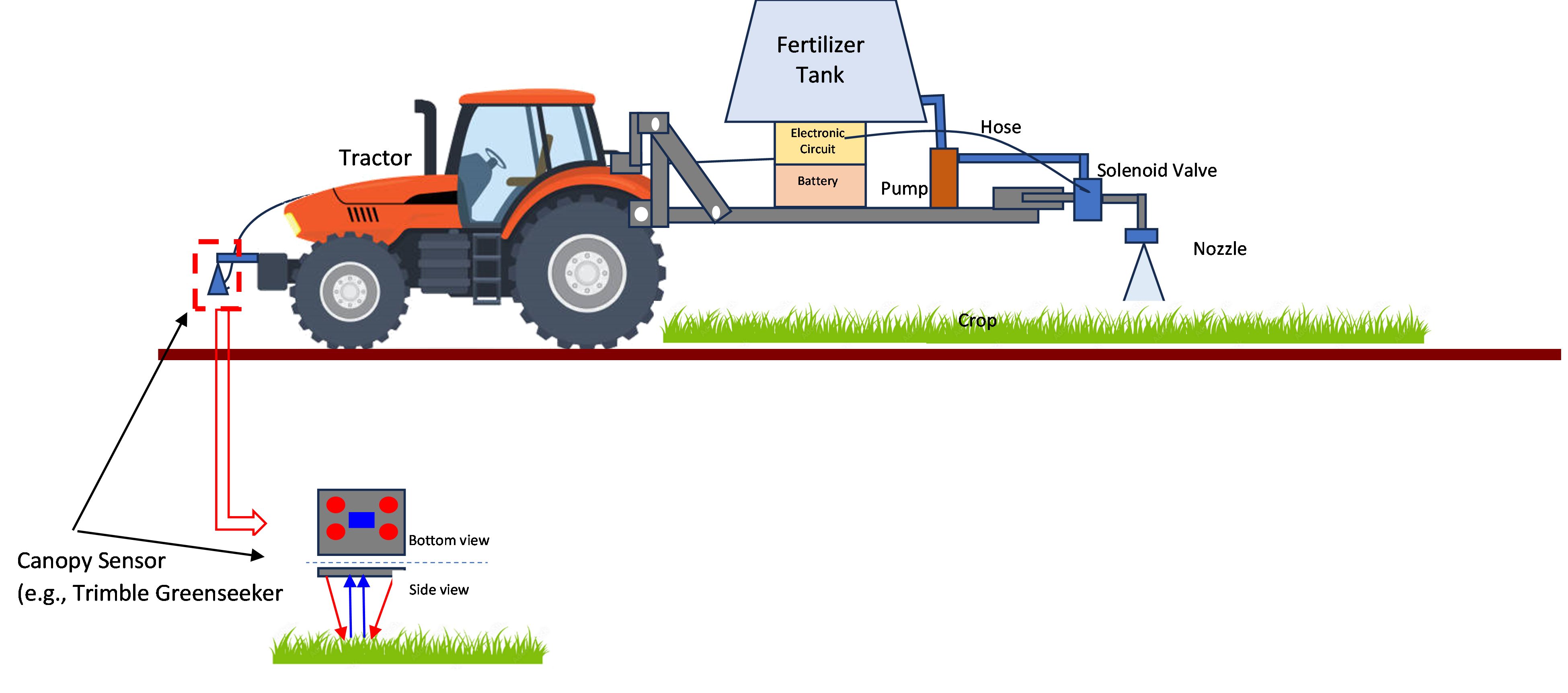
Credit: Vivek Sharma, UF/IFAS
In general, the algorithm is a set of equations that will convert sensor readings into an application rate. Although some custom functions are available for different crops, it is advisable to develop a site-specific algorithm for the relevant crop and region. To develop an algorithm, the first step is to develop a relationship between the sensor measurements (e.g., NDVI) and the crop properties of concern (e.g., yield, leaf nitrogen content, chlorophyll content, etc.). Once the relationship (equation) is developed, the next step is to determine the application rate as a function of sensor reading. Figure 4 represents the schematic of the sensor-based variable rate fertilizer application system.
Often, developing this relationship and determining the variable application rate require research-based data and vary considerably based on management practices, etc. An alternative approach is the use of nitrogen-rich strips or ramped calibration strips. The idea is to compare the spatial variability of the crop growth within the field to crop growth from the nitrogen-rich strip, where nitrogen is not a yield-limiting factor. Consequently, as the variable rate applicator covers the field, it compares the sensors-measured NDVI values with the NDVI values of the nitrogen-rich strip and adjusts the nitrogen application rate accordingly. For example, if the NDVI value of the nitrogen-rich strip was 0.6, and the NDVI value at the particular location in the field was 0.7, no nitrogen would be applied because sufficient nitrogen was available. However, if the NDVI value sensed by the sensor was below 0.6 (e.g., 0.4) at another location, then nitrogen would be applied at that location. Compared to a nitrogen-rich strip, the ramped calibration strip is used to create a continuous gradient (e.g., low to high nitrogen levels) so that sensors or measuring devices can be calibrated to detect and measure variations in the environment across a range of conditions (Raun et al. 2008).
Sensor-Based Sprayers for Tree Crops
Sensor-based variable rate sprayers utilize sensing systems to detect the presence of an object (e.g., a tree) and its height. Based on that information, they control individual spraying nozzles or zones of nozzles. In one example, Chemical Containers Inc. (Lake Wales, FL) developed the CC-Eye 8000 Tree Sense Control System, which utilizes GPS, six infrared “eye” sensors, and a system able to control 6 or 8 spraying zones. When a tree (object) is detected by the eye sensor in a particular zone, the Tree Sensing System (software) sends a signal to turn on the respective “spraying” zone(s) at the correct time to ensure accurate delivery of the application to the target tree. If a tree (or any other object) is not detected, then the software shuts off all nozzles to reduce waste of chemicals, environmental pollution, etc. This control system can also be used for fertilizer spreaders. Another sensor-based and smart tree crop sprayer was developed at UF/IFAS. It utilizes sensor fusion (i.e., two RGB cameras, a Lidar, and a GPS) and AI to detect trees, estimate tree height and canopy leaf density, and apply the right amount of chemicals at a tree level, minimizing waste of chemicals and spray drift (Partel et al. 2021). This AI-enabled sensing system can distinguish a tree from other objects (e.g., poles, pumps, etc.) and only spray on trees based on their canopy height and leaf density. At the same time, it can detect and count fruits (video demo: https://www.youtube.com/watch?v=qRd4g44b2lk) for yield prediction purposes (Vijayakumar et al. 2023), and develop spray and fruit heat maps (Costa and Ampatzidis 2022).
AI-Based Smart Sprayers for Precision Weed Management
Traditional sprayers apply herbicides uniformly, even though the distribution of weeds is typically patchy. Uniform applications of herbicides could increase costs, risk of crop damage, environmental pollution, and contamination of edible products. To address this issue, several AI-enabled, target-based technologies have been developed for the precision management of weeds in row and specialty crops (Vijayakumar et al. 2023). These technologies utilize machine vision and AI to distinguish weeds from crops and only target individual weeds. Examples of these technologies include the “see and spray” technology developed by Blue River Technology and John Deere, which utilizes herbicides to target individual weeds, and the Carbon Robotics LaserWeeder, which uses lasers to kill weeds. UF/IFAS developed another AI-enhanced technology for precision weed management, which can detect and classify weeds (i.e., grass, broadleaf, and other), and if needed, spray only a specific type of weeds (Partel et al. 2019; Ampatzidis 2018).
Summary
The VRT is one of the most powerful tools in precision agriculture that can be adopted in various farming practices to achieve high resource efficiency and maximum yields. VRT can create uniform conditions across the field by applying variable rates of inputs depending on the field variability. This technology can be adopted in both map-based and sensor-based methods. Map-based VRT requires lots of data to develop a prescription map. On the other hand, sensor-based technology uses sensors to determine the application rate. The cost associated with map- and sensor-based VRT is largely dependent on the field variability. For a uniform field, the value of VRT would be minimal; however, farms with significant variability may greatly benefit from VRT.
References
Ampatzidis, Y. 2018. “Applications of Artificial Intelligence for Precision Agriculture: AE529, 12/2018.” EDIS 2018 (6). https://doi.org/10.32473/edis-ae529-2018
Campos, J., M. Gallart, J. Llop, P. Ortega, R. Salcedo, and E. Gil. 2020. “On-Farm Evaluation of Prescription Map-Based Variable Rate Application of Pesticides in Vineyards.” Agronomy 10 (1): 102. https://doi.org/10.3390/agronomy10010102
Costa, L., S. Kunwar, Y. Ampatzidis, and U. Albrecht. 2022. “Determining Leaf Nutrient Concentrations in Citrus Trees Using UAV Imagery and Machine Learning.” Precision Agriculture:1–22. https://doi.org/10.1007/s11119-021-09864-1
Costa, L., J. McBreen, Y. Ampatzidis, J. Guo, M. R. Gahrooei, and M. A. Babar. 2022. “Using UAV-Based Hyperspectral Imaging and Functional Regression to Assist in Predicting Grain Yield and Related Traits in Wheat Under Heat-Related Stress Environments for the Purpose of Stable Yielding Genotypes.” Precision Agriculture 23 (2): 622–642. https://doi.org/10.1007/s11119-021-09852-5
Grisso, R. D., M. M. Alley, W. E. Thomason, D. L. Holshouser, and G. T. Roberson. 2011. “Precision Farming Tools: Variable-Rate Application.” Virginia Cooperative Extension. https://www.researchgate.net/publication/309121121_Precision_farming_tools_Variable-rate_application
Kakarla, S. C., and Y. Ampatzidis. 2021. “Types of Unmanned Aerial Vehicles (UAVs), Sensing Technologies, and Software for Agricultural Applications: AE565, 10/2021.” EDIS 2021 (5). https://doi.org/10.32473/edis-ae565-2021
Lark, R. M., and J. V. Stafford. 1997. “Classification as a First Step in the Interpretation of Temporal and Spatial Variation of Crop Yield.” Annals of Applied Biology 130 (1): 111–121. https://doi.org/10.1111/j.1744-7348.1997.tb05787.x
Mylavarapu, R. S., and W. S. D. Lee. 2020. “UF/IFAS Nutrient Management Series: Soil Sampling Strategies for Precision Agriculture: SL 190, 02/2020.” EDIS. https://edis.ifas.ufl.edu/ss402
O’Shaughnessy, S. A., S. R. Evett, P. D. Colaizzi, M. A. Andrade, T. H. Marek, D. M. Heeren, F. R. Lamm, and J. L. LaRue. 2019. “Identifying Advantages and Disadvantages of Variable Rate Irrigation: An Updated Review.” Applied Engineering in Agriculture 35 (6): 837–852. https://doi.org/10.13031/aea.13128
Partel, V., L. Costa, and Y. Ampatzidis. 2021. “Smart Tree Crop Sprayer Utilizing Sensor Fusion and Artificial Intelligence.” Computers and Electronics in Agriculture 191:106556. https://doi.org/10.1016/j.compag.2021.106556
Partel, V., S. C. Kakarla, and Y. Ampatzidis. 2019. “Development and Evaluation of a Low-Cost and Smart Technology for Precision Weed Management Utilizing Artificial Intelligence.” Computers and Electronics in Agriculture 157:339–350. https://doi.org/10.1016/j.compag.2018.12.048
Raun, W. R., J. B. Solie, R. K. Taylor, D. B. Arnall, C. J. Mack, and D. E. Edmonds. 2008. “Ramp Calibration Strip Technology for Determining Midseason Nitrogen Rates in Corn and Wheat.” Agronomy Journal 100 (4): 1088–1093. https://doi.org/10.2134/agronj2007.0288N
Sharma, V., D. R. Rudnick, and S. Irmak. 2013. “Development and Evaluation of Ordinary Least Squares Regression Models for Predicting Irrigated and Rainfed Maize and Soybean Yields.” Transactions of the ASABE 56 (4): 1361–1378. https://doi.org/10.13031/trans.56.9973
United States Department of Agriculture (USDA). 2023. “Precision Agriculture in the Digital Era: Recent Adoption on U.S. Farms.” Economic Information Bulletin No. (EIB-248). https://ers.usda.gov/publications/pub-details/?pubid=105893
Vijayakumar, V., Y. Ampatzidis, and L. Costa. 2023. “Tree-Level Citrus Yield Prediction Utilizing Ground and Aerial Machine Vision and Machine Learning.” Smart Agricultural Technology 3:100077. https://doi.org/10.1016/j.atech.2022.100077