Unmanned aerial systems (UASs, UAVs, or drones) have emerged as one of the most promising technologies for agricultural operation management in recent decades. Simplifications in UAS operation and development of cloud-based image processing pipelines have increased the accessibility of these technologies to a wide variety of users. From the standpoint of potential users, these developments have raised the possibility of the use of UASs for day-to-day farm management. This EDIS publication provides an overview of the broad areas where UASs can be utilized for monitoring and managing farm operations. It is the first in a series of three publications that covers: 1) an overview of the potential applications of unmanned aerial systems (UASs) for agricultural operations management in Florida (this paper), 2) an overview of UAS platforms and payloads relevant to UAS applications in agriculture, and 3) an overview of best practices for efficient UAS-based aerial surveying.
These publications will set the groundwork for subsequent publications on UAS applications in agricultural management detailing: 1) the computation, interpretation, and use of image products such as NDVI from UAS imagery; 2) the use of emerging technologies such as spectroscopy for crop water and nutrient management; and 3) advanced applications of LiDAR and hyperspectral imaging for soil moisture and plant health assessments at field scales. This series is a compendium on sensors and upcoming technologies that are relevant to practitioners and Extension agents as practical examples of applications of UASs for monitoring nutrients, water stress, diseases, weeds, and eventually, yields for field and tree crops in Florida.
Introduction
Advances in the automation of agricultural operations have provided a boost to reducing farmers' logistics and labor costs while simultaneously increasing agricultural production efficiencies, and in many cases, possibly improving worker safety outcomes (Bac et al. 2014; Bergerman et al. 2012; Edan et al. 2009; Fathallah 2010; Grift et al. 2008). Newer technologies are also helping agricultural operations in managing environmental and economic risks that may have important sustainability and financial implications (Stubbs 2016; World Bank 2005). This is especially true for Florida, considering ongoing changes in the rules and regulations that govern the use of natural resources. Over the past 80 years, the population of Florida has quadrupled from approximately 5 million to more than 20 million people. By 2070, Florida's population is estimated to grow by another 15 million people (Water 2070 Summary Report 2016). Based on current and projected scenarios, it is important to critically evaluate newer technologies and educate producers on appropriate uses for increasing efficiencies in farm operations management. Unmanned aerial systems (UASs, UAVs, or drones) are among the many promising technologies that can help achieve these goals.
Remote sensing technologies have a long history of use in mapping and monitoring natural and human-managed landscapes. While satellite remote sensing has enabled the management of large landscapes by helping generalize point-based observations to entire regions, processing these data into operationally useful products has remained largely within the domain of trained professionals. Recent developments in sensor miniaturization and out-of-the-box usability of UASs have enhanced the accessibility of these technologies to a far larger cohort of users. UASs can now be used to collect data over field-scale operations as frequently as needed at high spatial resolutions and to help simplify a wide variety of applications that might otherwise be logistically difficult, resource-intensive, or prohibitive in time and labor.
The use of UASs has expanded in recent times due to increased equipment affordability and reliability as well as availability of processing software developed specifically to process UAS data. Industry sectors are consequently developing innovative ways to use UASs in agriculture by leveraging the ability to fly on-demand missions at low altitudes, allowing for frequent monitoring of crops (Gago et al. 2015). UASs have been used to provide useful details about plant health, agricultural water management (quality and quantity), weed management, and growth characteristics (Herwitz et al. 2004; Berni et al. 2009; Huang et al. 2013), to detect diseases (Abdulridha et al. 2019a; Abdulridha et al. 2019b; Harihara et al. 2019), to evaluate rootstock (Ampatzidis et al. 2019a), and to perform crop phenotyping (Ampatzidis et al. 2019b). UASs equipped with thermal sensors can also help identify areas of potential under- and over-irrigation in real time for managing and optimizing irrigation water use (Gago et al. 2015; Zarco-Tejada, González-Dugo, and Berni 2012; Baluja et al. 2012). While many technological advances have been made in increasing usability of UASs, algorithms still need to be customized to specific local contexts to be operationally feasible for irrigation management, plant nutrient demand, and other areas of direct applicability to agriculture.
In general, data obtained from UAS sensors are used to develop baseline maps to identify issues related to crop production by looking at the measures of light reflected off plant canopies. Each plant canopy has its own characteristic way of reflecting certain wavelengths of light that change with variations in the plant's structural or biochemical attributes. A plethora of image indices (i.e., ratios or combination of wavelengths; e.g., Normalized Difference Vegetation Index, NDVI = [Near infrared – Red]/[Near infrared + Red]) can be used to derive indicators of plant health, canopy coverage, and/or water status of canopies. Henrich et al. (2009) have compiled a comprehensive list of image and spectral indices that are often utilized in remote sensing studies. A follow-up publication in this series will describe a subset of these indices that can specifically be derived using aerial imagery from UASs.
Requirements to Use UASs
Before planning any activity involving UASs, the user must have a comprehensive understanding of the legal and regulatory requirements of using UASs from the Federal Aviation Administration (FAA). In August 2016, FAA released new rules about the registration of UASs for commercial use. These rules apply to UASs weighing more than 0.55 lb and less than 55 lb. Requirements include, among other things, passing an exam to obtain a remote pilot airman certificate. There are many resources available to help obtain this certification, starting with the FAA website (https://www.faa.gov/uas/getting_started/). For a primer on legal and operational issues in the use and operation of UASs, see EDIS publications by Kakarla and Ampatzidis (https://edis.ifas.ufl.edu/ae527; https://edis.ifas.ufl.edu/ae535).
Airframes and Sensors
There are many different types of UASs that can be selected for agricultural operations. These include multirotor (quadcopters, hexacopters), fixed-wing aircraft, and/or hybrid vertical takeoff and landing (VTOL) airframes. There are substantial tradeoffs to consider when selecting the appropriate airframe, depending on flight requirements (i.e., flight time, maximum/minimum altitude, stability, takeoff/landing characteristics) and/or sensor payload requirements. Regardless of the airframe, one of the most critical components in any UAS is the choice of the sensor payload, which could include imaging sensors, laser scanners, or thermal cameras. An imaging sensor is much like a camera on a typical cell phone and collects reflected light in three broad spectral regions: red, green, and blue (RGB: Red: 600nm, Green: 500nm, and Blue: 400nm). Multispectral sensors extend the spectral sampling to wavelength ranges beyond the visible spectrum (e.g., near-infrared NIR: 700–1000nm), and hyperspectral sensors extend the spectral sampling to multiple contiguous wavelength intervals (e.g., 270 wavebands in the 400–700nm range using the Headwall Nano® imaging sensor). For agricultural use, multispectral sensors generally span the RGB visible wavelength regions, and extend the sampling to the NIR band. Improvements in manufacturing technologies in imaging sensors spanning the Visible-NIR (VNIR) spectrum can now support very high-resolution images ranging from HD (1080 pixels across the diagonal), 4K (or 4096 × 2160 pixels) to even 20MP for digital single-lens reflex (DSLR) cameras outfitted with bandpass filters. Multispectral sensors can produce images used to quantify vegetation vigor by contrasting the reflectance in the red wavelengths with that of NIR wavelengths (Figure 1). Variations in patterns observed from these contrasts can reveal multiple facets of vegetation condition.
![Figure 1. Patterns of NDVI (contrast between red and NIR wavebands calculated as [NIR - Red]/[NIR + Red]) scale the image between -1.0 (no vegetation) to +1.0 (full canopy with around three leaf layers). This image from a turfgrass operation in Hastings, FL shows variations of canopy closure in an early stage of development. Transitional regions between deep blue or red indicate variations between no vegetation (blue) to full canopy (red). Note artifacts in coloration around the truck parked at the northwestern corner of the image.](/image/AE541/15539494/9648634/9648634-2048.webp)
Credit: Aditya Singh, UF/IFAS
Although thermal imaging sensors do not provide high-resolution imagery, they can be used to detect plant water stress and irrigation management by contrasting the temperature of a canopy with the ambient temperature and relative humidity (Gago et al. 2015; Baluja et al. 2012). Recording electromagnetic radiation between the 7–13.5 µm range of the electromagnetic spectrum (i.e., heat), thermal imagery converts longwave infrared radiation (LWIR) radiated off crops into a spatial map of canopy skin temperature (Figure 2).
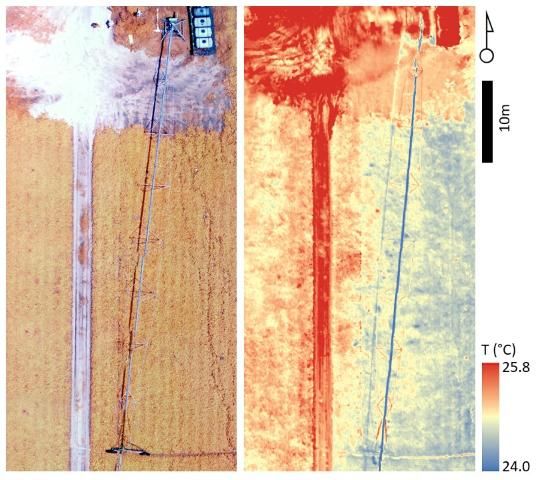
Credit: Aditya Singh, UF/IFAS
Plant canopy temperature profiles generally correlate well with water stress, mostly resulting from reductions in evapotranspirative cooling of the plant canopy (Kustas et al. 2003; Allen, Tasumi, and Trezza 2007). Research has shown that spectral reflectance data from UAS imagery can be used as a tool to monitor plant nutrition and health, specifically nitrogen (Caturegli et al. 2016). UAS thermal imagery has served as a tool to determine water stress in a variety of tree and agronomic crops (Sullivan, Fulton, and Shaw 2007; Gonzalez-Dugo, Zarco-Tejada, and Nicolás 2013; Gómez-Candón et al. 2016). The second publication in this series provides a selection guide for platforms and sensors.
Image Data Acquisition and Post-processing
There have also been many advances in both commercial and open-source software platforms for planning and executing aerial surveys, image stitching, and post-processing of aerial imagery. Mobile applications are available to create flight plans which allow for autopilot of the UASs (e.g., DroneDeploy®, DJI Go®), and software is available for postflight image processing (e.g., Agisoft Metashape®, Pix4D®) and for stitching individual images into maps. Image post-processing software programs also allow the creation of standard vegetative index maps such as NDVI. While the operational details differ considerably, the ease of use of these platforms has been increasing each year. See examples for a specific software implementation by Kakarla and Ampatzidis in https://edis.ifas.ufl.edu/ae533. The third publication in this series helps frame data collection protocols for efficient aerial campaigns.
Operational Limitations of UASs
There are several limitations to using UASs and various sensors, depending upon the application context, and sometimes upon economic constraints. Most commercial off-the-shelf (COTS) UASs have limited flight duration ability. The average flight time for a small quadcopter with a standard battery pack is around 20 minutes. While battery modules can be added to extend flight time, this comes at a cost of payload capacity, thus limiting UAS applications in larger fields. Fixed-wing aircraft have longer flight times (up to 5 hours), but lack some of the maneuverability of multirotors and have additional safety concerns during takeoff or landing. Although flight programs allow the UASs to return for battery replacement at a certain level and to continue the flight plan, the process itself can be time-consuming and may add extra costs for charging and maintaining multiple batteries at optimal charge.
One of the most important limitations of the use of UASs in agricultural applications is the quality and reliability of the data collected by the sensors. Specific recommendations for nutrient and water applications are generally not possible without careful calibration and validation of sensor data. Current work at UF/IFAS involves the development of algorithms for both multispectral and thermal imaging in an effort to quantify results and make recommendations for both fertilizer and water applications. These issues are even more critical for applications involving thermal sensors because these need additional steps for validation from ground-based sensors that might not be readily available to the general user. Thermal applications may be limited by any combination of issues, such as the sensitivity of observations, behavior of surfaces related to time of day, leaf location, and angle of the sun (Jones et al. 2009). In general, the use of a single sensor provides limited information. When combined with multispectral and/or thermal sensors, it can assist in diagnosis of both primary (water deficit) and biotic (pest and disease) stresses (Leinonen and Jones 2004; Chaerle et al. 2007).
Conclusion
The wide commercial availability and affordability of UASs have raised the potential of their use by agricultural producers for day-to-day farm management operations. However, it should be noted that integrated systems that allow for real-time irrigation, nutrient, and/or disease management have not yet been implemented in most operational settings. According to Wright and Small (2016), the key to the successful use of precision agricultural technology will be the availability of algorithms that translate data, such as weather data, UAS imagery, and yield maps, into actionable information that can be used by growers to improve efficiency and profitability of their operations. These EDIS publications are intended to chart the way forward and help growers and Extension agents make informed decisions in this new and emerging field.
References
Abdulridha, J., Y. Ampatzidis, S. C. Kakarla, and P. Roberts. 2019a. "Detection of Target Spot and Bacterial Spot Diseases in Tomato Using UAV-based and Benchtop-based Hyperspectral Imaging Techniques." Precision Agriculture (November): 1–24.
Abdulridha, J., R. Ehsani, A. Abd-Elrahman, and Y. Ampatzidis. 2019b. "A Remote Sensing Technique for Detecting Laurel Wilt Disease in Avocado in Presence of Other Biotic and Abiotic Stresses." Computers and Electronics in Agriculture 156 (January 2019): 549–557.
Allen, R. G., M. Tasumi, and R. Trezza. 2007. "Satellite-Based Energy Balance for Mapping Evapotranspiration with Internalized Calibration (METRIC)—Model." Journal of Irrigation and Drainage Engineering 133(4): 380–394. https://doi.org/10.1061/(ASCE)0733-9437(2007)133:4(380)
Ampatzidis, Y., V. Partel, B. Meyering, and U. Albrecht. 2019a. "Citrus Rootstock Evaluation Utilizing UAV-based Remote Sensing and Artificial Intelligence." Computers and Electronics in Agriculture 164: 104900. doi.org/10.1016/j.compag.2019.104900
Ampatzidis, Y., and V. Partel. 2019b. "UAV-based High Throughput Phenotyping in Citrus Utilizing Multispectral Imaging and Artificial Intelligence." Remote Sensing 11(4): 410. doi: 10.3390/rs11040410.
Bac, C. W., E. J. van Henten, J. Hemming, and Y. Edan. 2014. "Harvesting Robots for High-Value Crops: State-of-the-Art Review and Challenges Ahead." Journal of Field Robotics 31(6): 888–911. https://onlinelibrary.wiley.com/doi/abs/10.1002/rob.21525
Baluja, J., M. P. Diago, P. Balda, R. Zorer, F. Meggio, F. Morales, and J. Tardaguila. 2012. "Assessment of Vineyard Water Status Variability by Thermal and Multispectral Imagery Using an Unmanned Aerial Vehicle (UAV)." Irrigation Science 30(6): 511–522. https://doi.org/10.1007/s00271-012-0382-9
Bergerman, M., S. Singh, and B. Hamner. 2012. "Results with Autonomous Vehicles Operating in Specialty Crops." In 2012 IEEE International Conference on Robotics and Automation. 1829–1835. https://doi.org/10.1109/ICRA.2012.6225150
Berni, J. A. J., P. J. Zarco-Tejada, L. Suarez, and E. Fereres. 2009. "Thermal and Narrowband Multispectral Remote Sensing for Vegetation Monitoring From an Unmanned Aerial Vehicle." IEEE Transactions on Geoscience and Remote Sensing: A Publication of the IEEE Geoscience and Remote Sensing Society 47(3): 722–738. https://doi.org/10.1109/TGRS.2008.2010457
Caturegli, L., M. Corniglia, M. Gaetani, N. Grossi, S. Magni, M. Migliazzi, L. Angelini, et al. 2016. "Unmanned Aerial Vehicle to Estimate Nitrogen Status of Turfgrasses." PloS One 11(6): e0158268. https://doi.org/10.1371/journal.pone.0158268
Chaerle, L., D. Hagenbeek, X. Vanrobaeys, and D. Van Der Straeten. 2007. "Early Detection of Nutrient and Biotic Stress in Phaseolus vulgaris." International Journal of Remote Sensing 28(16): 3479–3492. https://doi.org/10.1080/01431160601024259
Edan, Y., S. Han, and N. Kondo. 2009. "Automation in Agriculture." Springer Handbook of Automation. https://link.springer.com/chapter/10.1007/978-3-540-78831-7_63
Fathallah, F. A. 2010. "Musculoskeletal Disorders in Labor-Intensive Agriculture." Applied Ergonomics 41(6): 738–743. https://doi.org/10.1016/j.apergo.2010.03.003
Fletcher, J., and T. Borisova. 2017. Collaborative Planning for the Future of Water Resources in Central Florida: Central Florida Water Initiative. FE1012. Gainesville: University of Florida Institute of Food and Agricultural Sciences. https://edis.ifas.ufl.edu/fe1012
Gago, J., C. Douthe, R. E. Coopman, P. P. Gallego, M. Ribas-Carbo, J. Flexas, J. Escalona, and H. Medrano. 2015. "UAVs Challenge to Assess Water Stress for Sustainable Agriculture." Agricultural Water Management 153(May): 9–19. https://doi.org/10.1016/j.agwat.2015.01.020
Gómez-Candón, D., N. Virlet, S. Labbé, and A. Jolivot. 2016. "Field Phenotyping of Water Stress at Tree Scale by UAV-sensed Imagery: New Insights for Thermal Acquisition and Calibration." Precision. http://link.springer.com/article/10.1007/s11119-016-9449-6
Gonzalez-Dugo, V., P. Zarco-Tejada, and E. Nicolás. 2013. "Using High Resolution UAV Thermal Imagery to Assess the Variability in the Water Status of Five Fruit Tree Species within a Commercial Orchard." Precision. http://link.springer.com/article/10.1007/s11119-013-9322-9
Grift, T., Q. Zhang, N. Kondo, and K. C. Ting. 2008. "A Review of Automation and Robotics for the Bio-Industry." Journal of Biomechatronics Engineering 1(1): 37–54. http://journal.tibm.org.tw/wp-content/uploads/2013/06/2.-automation-and-robotics-for-the-bio-industry.pdf
Harihara, J., J. Fuller, Y. Ampatzidis, J. Abdulridha, and A. Lerwill. 2019. "Finite Difference Analysis and Bivariate Correlation of Hyperspectral Data for Detecting Laurel Wilt Disease and Nutritional Deficiency in Avocado." Remote Sensing 11(15): 1748. https://doi.org/10.3390/rs11151748
Henrich, V., E. Götze, A. Jung, C. Sandow, D. Thürkow, and C. Gläßer. 2009. "Development of an Online Indices-Database: Motivation, Concept and Implementation." EARSeL Proceedings. Tel Aviv: EARSeL.
Herwitz, S. R., L. F. Johnson, S. E. Dunagan, R. G. Higgins, D. V. Sullivan, J. Zheng, B. M. Lobitz, et al. 2004. "Imaging from an Unmanned Aerial Vehicle: Agricultural Surveillance and Decision Support." Computers and Electronics in Agriculture 44(1): 49–61. https://doi.org/10.1016/j.compag.2004.02.006
Huang, Y., S. J. Thomson, W. C. Hoffmann, Y. Lan, and B. K. Fritz. 2013. "Development and Prospect of Unmanned Aerial Vehicle Technologies for Agricultural Production Management." International Journal of Agricultural and Biological Engineering 6(3): 1–10. https://doi.org/10.3965/j.ijabe.20130603.001
Jones, H. G., R. Serraj, B. R. Loveys, L. Xiong, A. Wheaton, and A. H. Price. 2009. "Thermal Infrared Imaging of Crop Canopies for the Remote Diagnosis and Quantification of Plant Responses to Water and Stress in the Field." Functional Plant Biology 36: 978–989.
Kustas, W. P., J. M. Norman, M. C. Anderson, and A. N. French. 2003. "Estimating Subpixel Surface Temperatures and Energy Fluxes from the Vegetation Index–Radiometric Temperature Relationship." Remote Sensing of Environment 85(4): 429–440. https://doi.org/10.1016/S0034-4257(03)00036-1
Leinonen, I., and H. G. Jones. 2004. "Combining Thermal and Visible Imagery for Estimating Canopy Temperature and Identifying Plant Stress." Journal of Experimental Botany 55(401): 1423–1431. https://doi.org/10.1093/jxb/erh146
Rouse, J. W., R. H. Haas, J. A. Schell, and D. W. Deering. 1973. "Monitoring the Vernal Advancement and Retrogradation (Green Wave Effect) of Natural Vegetation." Vol. NTIS No. E73–106393. Prog. Rep. RSC 1978-1. College Station, TX: Remote Sensing Center, Texas A&M Univ.
Stubbs, M. 2016. Irrigation in US Agriculture: On-Farm Technologies and Best Management Practices. Washington, D.C.: Congressional Research Service. http://nationalaglawcenter.org/wp-content/uploads/assets/crs/R44158.pdf
Sullivan, D. G., J. P. Fulton, and J. N. Shaw. 2007. "Evaluating the Sensitivity of an Unmanned Thermal Infrared Aerial System to Detect Water Stress in a Cotton Canopy." Transactions of the American Society of Agricultural and Biological Engineers. https://elibrary.asabe.org/abstract.asp?aid=24091
Water 2070 Summary Report. 2016. "Mapping Florida's Future—Alternative Patterns of Water Use in 2070." Accessed on June 1, 2020. http://1000friendsofflorida.org/water2070/wp-content/uploads/2016/11/water2070summaryreportfinal.pdf
World Bank. 2005. Shaping the Future of Water for Agriculture: A Sourcebook for Investment in Agricultural Water Management. World Bank Publications. https://play.google.com/store/books/details?id=groiOguWVlgC
Zarco-Tejada, P. J., V. González-Dugo, and J. A. J. Berni. 2012. "Fluorescence, Temperature and Narrow-Band Indices Acquired from a UAV Platform for Water Stress Detection Using a Micro-Hyperspectral Imager and a Thermal Camera." Remote Sensing of Environment 117(February): 322–337. https://doi.org/10.1016/j.rse.2011.10.007