Precision Farming Adoption by Florida Citrus Producers: Probit Model Analysis
Introduction
Production practices in agriculture are constantly changing and being modified. The introduction of site-specific crop management (SSCM), also known as precision farming, can be considered the newest advance in production agriculture and mechanization. The use of multiple technologies and common production practices have opened a new era of "high-tech" farming. The use of soil sampling; yield monitoring; remote sensing; and variable-rate applications of herbicide, pesticide, and fertilizer, as well as the Global Positioning System (GPS) and a geographic information system (GIS) can be considered precision agriculture.
The purpose of precision agriculture is multifold. First, growers are always seeking ways to increase profits by maximizing yield while simultaneously decreasing production costs by managing input applications. Second, producers are becoming more environmentally aware and, as a result of managing inputs, are implementing more environmentally friendly practices (Sevier and Le, 2003). Potentially, growers can realize economic benefits by reducing their overall cost of production; likewise the environment benefits and what appears to be a win-win situation is a result of simply being able to manage inputs to production. Simply stated, Morgan and Ess (2003) provided the following definition for precision agriculture: "managing each crop production input...on a site-specific basis to reduce waste, increase profits, and maintain the quality of the environment."
There is a large potential for the adoption of precision technologies in citrus production. Florida citrus production has experienced a rather volatile trend in cost of production per unit area. The premise behind SSCM technologies would lend itself perfectly to the production scenario in citrus. If growers were able to manage their input applications on a site-specific basis, then the cost of production could be maintained at an "acceptable" level. Several studies have shown that the adoption of precision agriculture technologies and practices would be biased towards crops or commodities that are input-intensive (Daberkow 1997); citrus may be a likely candidate as well.
Objectives
There are several items that need to be identified before additional research regarding precision agriculture in citrus production can proceed based on the current status of precision agriculture adoption in Florida citrus. This study will identify the current trend of adoption for citrus producers in the top 10 counties in Florida and will investigate the current attitudes of adopters versus non-adopters towards technology in general. We also want to determine what the decision criteria are for technology adoption and which, if any, are being used.
The enumerated goals for this research are as follow:
- Determine what the barriers for entry/adoption of precision farming are.
- Quantify the adoption rate based on responses to a survey questionnaire.
- Identify the demographic characteristics that may describe adopters and non-adopters.
- Determine if the adoption of precision agriculture technologies is based on "some" demographic factor.
In addition, we want to ascertain if technology adoption will follow similar trends to those seen in "traditional" commodities, and if we can categorize adopters and non-adopters into groups, such as:
- Early-adopters.
- Coat-tailors.
- Slow-to-adopt.
- Do it because it is there.
- Non-adopters.
In identifying a sample, we selected the top 10 citrus-producing counties in the state based on the area in citrus production. These counties and their respective percentage of total citrus production area in the state are itemized in Table 1. Although the 11th through 13th ranked counties are provided in Table 1, they were not sampled because the top 10 counties represented more than 80% of the production area in the state, which was sufficient for sampling purposes.
There were 2,391 growers identified in this 10-county sampling region. Of that group, 1,232 were randomly selected to receive the questionnaire. The questionnaire investigated the following technologies and their current use or planned use (the following references to companies and their products are not an endorsement by the authors or the University of Florida):
- Sensor-based variable rate applicators (e.g., "Tree See").
- Prescription map-based variable rate applicators (e.g., "Legacy 6000").
- Pest scouting and mapping (e.g., "EntoNet").
- Weed scouting and mapping.
- Remote sensing (e.g., aerial or satellite imagery).
- GPS receiver (e.g., boundary mapping).
- Soil variability mapping.
- Water table monitoring (e.g., automated irrigation scheduling).
- Harvesting logistics (e.g., mapping brix, acid, and sugar levels to determine peak harvest time).
- Yield monitoring (e.g., GOAT yield monitoring system).
A second matrix was used to determine the cause of negative responses to adoption. Respondents were asked to place a checkmark in the fields to identify their attitudes toward each of the respective technologies. The selections provided to the respondents for "NOT Adopting" or "NO PLAN TO Adopt" were:
- Not enough information.
- Not profitable.
- Lack of capital.
- Process/equipment not reliable.
- Process/equipment too complex for laborers.
- Satisfied with current practices.
- Other (please specify).
Additional information was collected for the purpose of establishing demographic profiles for adopters versus non-adopters. In addition, these questions will provide information pertaining to future research on the cost of production estimates for these growers in connection with the profile that is built. These questions included:
- Grower demographic information (age, highest education level achieved, and grove management experience).
- Personal willingness to adopt technology.
- Their ability to identify the current level of in-grove variability.
Survey Results and Discussion
To date, 304 questionnaires (24.7% of total mailed) have been received, with 211 of those returned completed. The completed responses accounted for 17.1% of the total mailed. The modified response rate accounting for the exclusions was calculated to be 18.5%, which was considered to be a sufficient percentage of completed questionnaires for analysis.
A response matrix was used in the questionnaires to identify which technologies were currently being used, as well as planned future usage. Currently, the most commonly used precision agriculture technologies are the sensor-based variable rate applicators (17.5% of the completed surveys indicated use), soil variability mapping (16.1%), and GPS boundary mapping (16.1%). The least commonly used technologies are currently remote sensing (e. g., aerial or satellite imagery) at 4.7% and "prescription map" variable rate controllers at 3.3%.
A second response matrix was used to determine reasons for "Not Adopting" precision farming technologies. By far, the most common response in this matrix was that producers were satisfied with their current production practices for all of the investigated technologies. The next most common responses were lack of information regarding the respective technologies and lack of capital to make investments in new technologies.
Respondents were also questioned on their "adoption attitude." This was their self-perceived willingness to adopt new technologies. The largest category, representing 62.0% of the respondents, indicated that, "I normally wait to see others' success with new technologies and production methods." This group of respondents would be categorized as "coat-tailors." Approximately 18% of the respondents were in the top two adoption attitude categories. They would be classified as "early-adopters." Roughly 13% of the respondents would be classified as "slow-to-adopt." Only 6.6% of the respondents omitted responses to this question.
The last section of the questionnaire was dedicated to the demographic profiles of the respondents. These questions investigated each respondent's age, years of experience in the citrus industry, and highest level of education achieved. Approximately 82% of the respondents reported having some college education, and 16% of the respondents reported a high school education or lower. Approximately 3% of the responses were unanswered for this question.
Experience in the citrus industry was a key variable we wanted to understand. Ultimately, is there a correlation with some demographic characteristic that can profile the adopters versus non-adopters? Sevier and Lee (2004) performing probit model analysis on this survey data concluded that there was a statistically significant negative correlation between age and precision farming technology adoption. There was, however, no correlation to experience. The complete results of the probit model analysis can be found in the next section. The resulting statistics for both age and years of experience are listed in Table 2.
Probit Model Theory
Linear regression assumes that the dependent variable being tested is both continuous and measured for all of the observations within the sample. In this survey, the dependent variable is not continuous; instead it is a dichotomous binary variable. The dependent variables were the 10 respective technologies, with each having two choices. The choices were designed to measure current adoption and planned adoption for each of the 10 technologies. Data were collected from surveys and recorded using a binary 0/1 response. Each respondent was scored a one (1) for a "yes" response to either "currently using" or "planning to use" a technology. Alternatively, a negative response was assigned a zero (0). Additionally, some survey respondents did not indicate a positive or negative response; hence an incomplete measurement. This statistical analysis has broken both initial assumptions of linear regression. This is the primary reason for using an alternative means of running a regression analysis on the survey data. The probit model analysis can appropriately handle the binary data that breaks the previous assumptions.
Equation 1 represents the final probit model used in this study. Definitions for variables are shown in Table 3. The dependent variable is USETECH, which represents the aggregation of all responses from the survey questioning current use of precision farming technology in Florida citrus production.
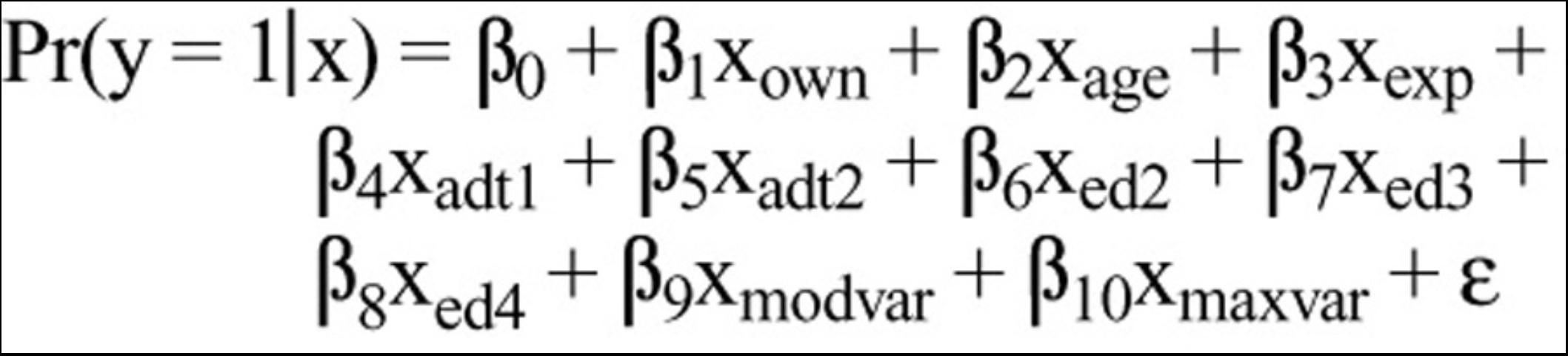
In Table 3, there are several multi-level variables that were present in the probit model. The variables for the respondents' self-perceived adoption attitude, their maximum education achieved, and the in-grove variability are each multi-level variables.
When multi-level variables are used as independent variables in a probit analysis, one level of the variable is excluded. Results are then interpreted by using the omitted level as the point of comparison for the other levels. The omitted variables are shown in Table 4. Also shown in Table 4 is a variable named DKVAR. This information was collected to allow respondents to indicate uncertainty of their in-grove variability. This variable was omitted entirely from the analysis since less than 1% (<1%) of the respondents chose this option. The probit model analysis was performed using a statistical software package named LIMDEP, version 7.0 (Greene, 1995). The significance level for this analysis was 90% (alpha = 0.9).
Probit Model Analysis Results and Discussion
The binomial probit model in Equation 1 was estimated using 135 observations. Recall, 1,232 surveys were distributed by mail. Respondents returned more than 300 surveys, of which 211 were considered to contain usable data. A probit model can only make estimates for complete responses in which every variable measured contains a response. This being the case, the probit model could only be used for 135 observations. Results indicated that three of the independent variables were statistically significant in influencing the decision to adopt precision farming technologies.
The variable for each grower's age was significant and negatively correlated to USETECH, indicating that as a grower's age increases, the likelihood of adopting precision farming technologies decreases.
The variables associated with in-grove variability resulted in two significant independent variables. The variables representing maximum variability and moderate variability were significant and positively related to the likelihood to adopt. The positive correlation indicates that either level of variability higher than minimum in-grove variability would influence the decision to adopt precision farming technologies. Marginal probabilities indicate that farmers with maximum and moderate variability are more likely to adopt the technology compared to those in the minimum variability group.
Table 5 illustrates predicted outcomes versus actual outcomes measured in the survey results. Recall, respondents were asked to identify from a list of 10 technologies if they were currently using or planning to use any of the technologies. For the sake of the probit model in this study, current usage was only taken into consideration (referred to as USETECH above). Those survey responses were measured against predicted outcomes of the binary probit model.
The benefit of the predicted outcomes matrix is in identifying the percentage of correct guesses versus naïve predictions by the probit model. In Table 5, you can tabulate that 99, or 73.3%, correct predictions were made (64 "no" responses and 35 "yes" responses). A correct prediction is when the model guesses "no" (0) and it actually was, and likewise when it predicts "yes" (1). If one were to make a naïve prediction, the correct prediction rate would be 76, or 56%. The naïve prediction is calculated by always guessing either "no" (0) or "yes" (1). In this case, we would always guess "no" (0), as we would be correct more frequently. Therefore, the probit model is better at predicting the dependent variable (73% correct prediction) compared to the naïve prediction (56%).
There are two types of incorrect predictions in a probit model: type I errors and type II errors. With a Type I error, the model incorrectly predicts "no" when it should have predicted "yes" (in our sample, this occurred 24 times). A Type II error occurs when the model predicts "yes" when it should predict "no" (in our sample, this occurred 12 times). A Type I error is not a major concern because if this model was established to assist growers in deciding whether to adopt based on their own demographic characteristics as inputs, an incorrect "no" prediction would simply result in growers not investing when they should have. The only measurable loss would be the opportunity cost of not adopting early to reap a major payback from investment in the technology. However, with a Type II error, the model would recommend that growers make rather large investments in technology, which would be inappropriate. The results from Table 5 indicate that there are only 12 Type II error predictions (approximately 8.9% of the sample) that would result in a mistaken investment.
Conclusion
This research set out initially to determine the current adoption rate of precision agriculture technologies by the citrus industry. We explored what attitudes were prevalent regarding the adoption of new technologies and production practices. The most commonly used technologies were sensor-based variable rate applicators and soil variability mapping. The least commonly used technology was remote sensing data which, as indicated in the open-ended responses, occurred as a result of the value of the information being far less than the cost to acquire the information.
The most prevalent reason for not adopting new technologies was quite simply that the respondents were satisfied with their current production practices - anecdotally, "why change it if it already works?" At best, the citrus industry is moving slowly with regard to adopting new technology and production practices.
The goal of this study was to identify certain key demographic characteristics of citrus growers that could influence the decision to adopt precision farming technologies. Results from a probit analysis with decision to adopt as the dependant variable indicated that variables associated with age and moderate and maximum spatial variability were significant influences on the decision to adopt. Age-influenced likelihood to adopt negatively influenced adoption, while both moderate and maximum in-grove spatial variability compared to minimum in-grove spatial variability were positive influences on the decision to adopt.
The probit model accurately predicted outcomes 73.3% of the time. In addition, Type II error predictions resulting in a mistaken decision to invest only occurred 8.9% of the time. Overall, the success of the probit model is average, at best. If this model was to be used as a grower decision tool, more data would need to be collected to validate the predictions. Although 8.9% is relatively low, it represents approximately 1 in 10 incorrect predictions about whether a grower should adopt precision farming technologies. That is an extremely expensive error when considering the cost of the technologies involved in precision farming in citrus.
References
Daberkow, S. 1997. Adoption Rates for Selected Crop Management Practices: Implications for Precision Farming. Choices (3d Quarter): 26–30.
Florida Agricultural Statistics Service. 2002. Commercial Citrus Inventory–2002. Orlando, FL: Florida Department of Agriculture and Consumer Affairs and USDA Agricultural Marketing Service and National Agricultural Statistics Service.
Greene, W.H. 1995. LIMDEP Version 7.0. Bellport, NY: Econometric Software.
Morgan, M. and D. Ess. 2003. The Precision-Farming Guide for Agriculturists, 2d Edition. Moline, IL: John Deere Publishing.
Sevier, B. J. and W. S. Lee. 2003. Adoption Trends and Attitudes Towards Precision Agriculture in Florida Citrus: Preliminary Results from a Citrus Producer Survey. ASAE Paper No. 031100, American Society of Agricultural Engineers, St. Joseph, MI.
Sevier, B. J. and W. S. Lee. 2004. Precision Agriculture in Citrus: A Probit Model Analysis for Technology Adoption. ASAE Paper No. 041092, American Society of Agricultural Engineers, St. Joseph, MI.
Top 14 citrus producing counties in Florida (Florida Agricultural Statistics Service 2002).