Introduction
The concentration of carbon dioxide (CO2) in the atmosphere has been steadily increasing over the past several decades, contributing significantly to the greenhouse effect and climate change. Therefore, to mitigate climate change strategies such as carbon (C) sequestration (the capture and storage of atmospheric CO2) and reductions in CO2 emissions are essential to address these challenges effectively. Soils have the capacity to function as a sink of atmospheric CO2 (i.e., sequestration) and are crucial for climate regulation. Globally, the soil stores around 1.5 to 2.4 trillion metric tons of C annually (Ciais et al. 2014) and can store three times more C than the atmosphere and four times more than plants (Griggs and Noguer 2002). These massive C sinks have the potential to reverse soil degradation, mitigate climate change, and enhance food security (Lal 2019) by increasing soil health and fertility, which in turn can lead to increased crop yields and quality. It is therefore essential to monitor the global C cycle by accurately measuring the amount of soil C in various ecosystems, including highly managed agricultural fields. For accurate soil C stock (or pool) calculations, common standards and procedures for different ecosystems worldwide are required. One of the most difficult aspects of estimating C is obtaining an accurate picture of the soil C stock. Because C stocks can vary greatly even within a single field, it is difficult to obtain a representative sample of soil for testing. Additionally, the selection of sampling methods, sampling design, baseline selections, depth of soil, and use of proper analytical methods are prerequisites for accurately estimating the soil C stocks and getting an overall picture of the area’s C levels at different scales.
This article summarizes current and emerging analytical methods for measuring C on different spatial scales, providing valuable information to extension agents, researchers, and other professionals. Other end-users in sectors such as forestry, ranch and cattle management, and ecosystem services such as wetlands, may also benefit from the information provided here. For more information on the role of growers in the C economy, including topics such as C credits and agriculture practices for C sequestration, please refer to EDIS publications #AE573 and #AE582.
Protocols for Quantifying Carbon
Measuring soil C is crucial for understanding soil health, carbon sequestration potential, and overall ecosystem functioning. Various methods (Figure 1) have been developed to quantify soil C at different scales, ranging from laboratory analyses to remote sensing techniques. These methods provide valuable insights into the spatial and temporal distribution of soil C, enabling scientists, researchers, and land managers to make informed decisions regarding sustainable land management practices and climate change mitigation strategies. Below, we delve into a comprehensive discussion of the prevalent methods used to measure soil C, exploring their principles, methodologies, and applications.
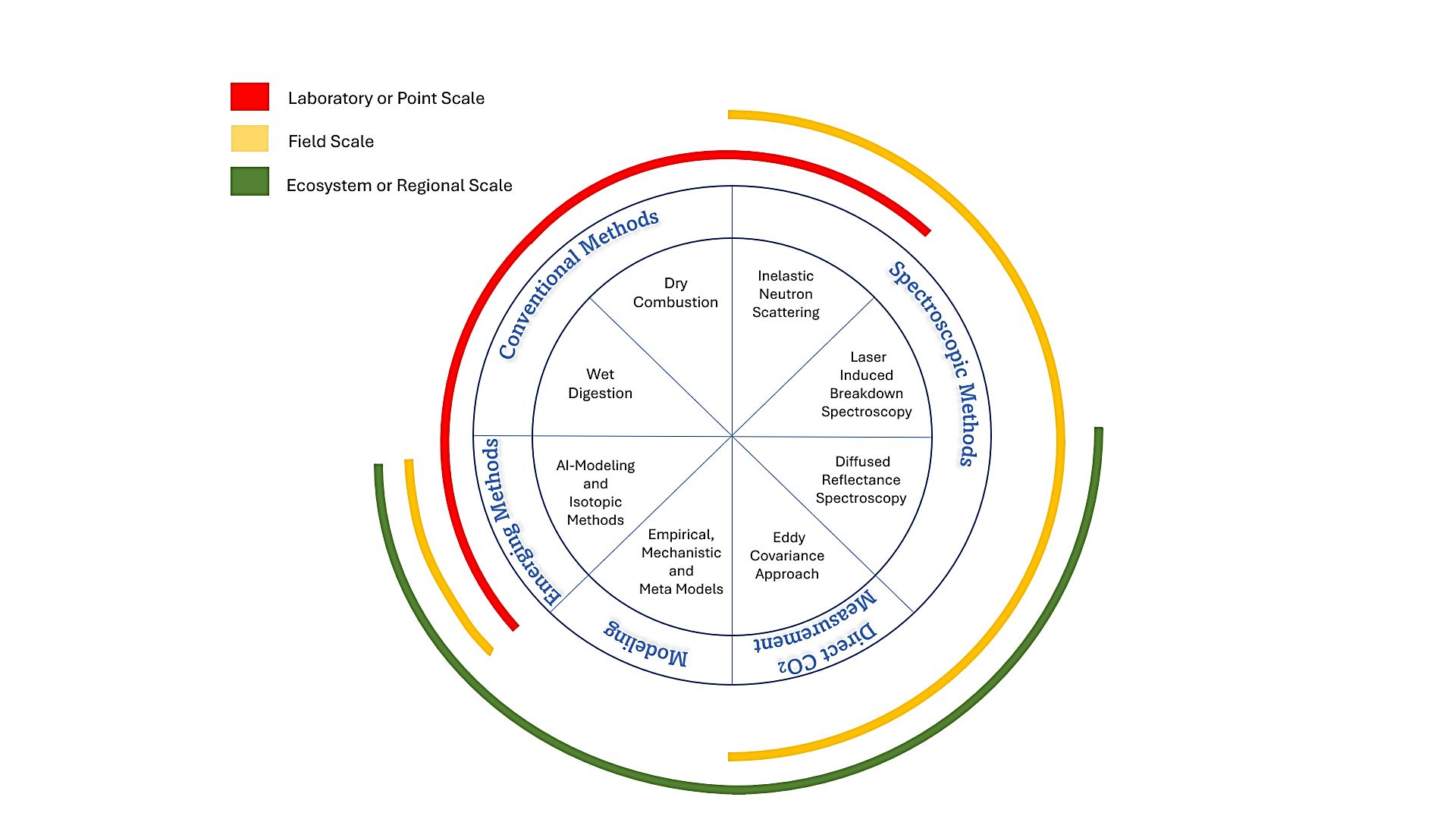
Credit: Suraj Melkani and Jehangir H. Bhadha, UF/IFAS
Conventional Methods
Wet digestion and dry combustion are conventional methods, widely used for regular laboratory analysis of C. These methods are the standard procedures available for measuring soil C. These procedures involve analyzing soil samples in a controlled environment to accurately determine the amount of C present. Typically, soil samples are taken using soil augers, which are cylindrical tools used to collect soil samples from a specific depth. The samples are then taken back to the laboratory for analysis. Soil C is measured at the point scale because it involves measurements representative of fields at specific locations and times.
Wet Digestion
The Walkley-Black dichromate oxidation method is a widely used laboratory method for determining C (Walkley and Black 1934), first by oxidizing C in soil with potassium dichromate and sulfuric acid. After the oxidation, the remaining unreacted potassium dichromate is titrated or reacted with a reducing agent like Ferrous Ammonium Sulfate along with an indicator like Diphenyl Amine. This titration process helps determine how much of the potassium dichromate was consumed during the oxidation process. The change in color of the solution, typically from orange to green, due to the reduction of potassium dichromate to chromium ions, plays a crucial role in determining the amount of unreacted potassium dichromate. This change in color is easily observable and serves as an endpoint indicator. The amount of unreacted potassium dichromate can then be related to the amount of carbon present in the original soil sample. This technique is extensively used worldwide because it is easy and rapid, while involving minimum apparatus. However, the wet digestion method may partially oxidize labile soil C, resulting in the loss of some labile carbon compounds during the digestion process. This is a concern because labile carbon is a major portion of soil C. To address these concerns, there is a need for correction factors when interpreting the results obtained through wet digestion methods. These correction factors are designed to account for the potential loss of labile carbon during digestion, ensuring that the estimated carbon content in the soil reflects the true carbon content, including both stable and labile carbon fractions (Neal and Younglove 1993). Labile C refers to the fraction of soil C that is more easily decomposable and can change rapidly in response to environmental factors. Despite being widely used to measure C concentration around the world, the wet digestion method has limitations, like the effects on labile C, because of variable C recovery percentage. However, the accuracy of C measurement using the wet digestion method can be improved through the use of exogenic heat during digestion and the development of site-specific correction factors.
Dry Combustion
The dry combustion method is a standard and precise laboratory technique for analyzing soil C concentration, which involves incineration of the soil C at a high temperature and measurement of the resulting CO2 using one of two methods:
- Loss-on-Ignition Method (LOI) (Matus et al. 1997) involves measuring the difference in mass of pre- and post-incinerated dry soils after achieving dry combustion in a high-temperature incinerator (Figure 2A). The variable sample size can be a source of error, and content of C measured with LOI reportedly decreases significantly with an increase in sample weight (Schulte et al. 1991).
- Dry Combustion with Elemental Analyzer (EA) (Atkins and Jones 1991) is the procedure for measuring C using EA, based on the collection of emitted CO2 after dry oxidation of C (Figure 2B). Dry oxidation involves heating the sample in a combustion chamber containing pure oxygen gas, which oxidizes the C in the sample to CO2 gas. The resulting CO2 gas is then collected and measured to determine the amount of C in the sample. However, it should be noted that carbonates do not completely oxidize in both LOI and EA methods, which can introduce variation when measuring C.
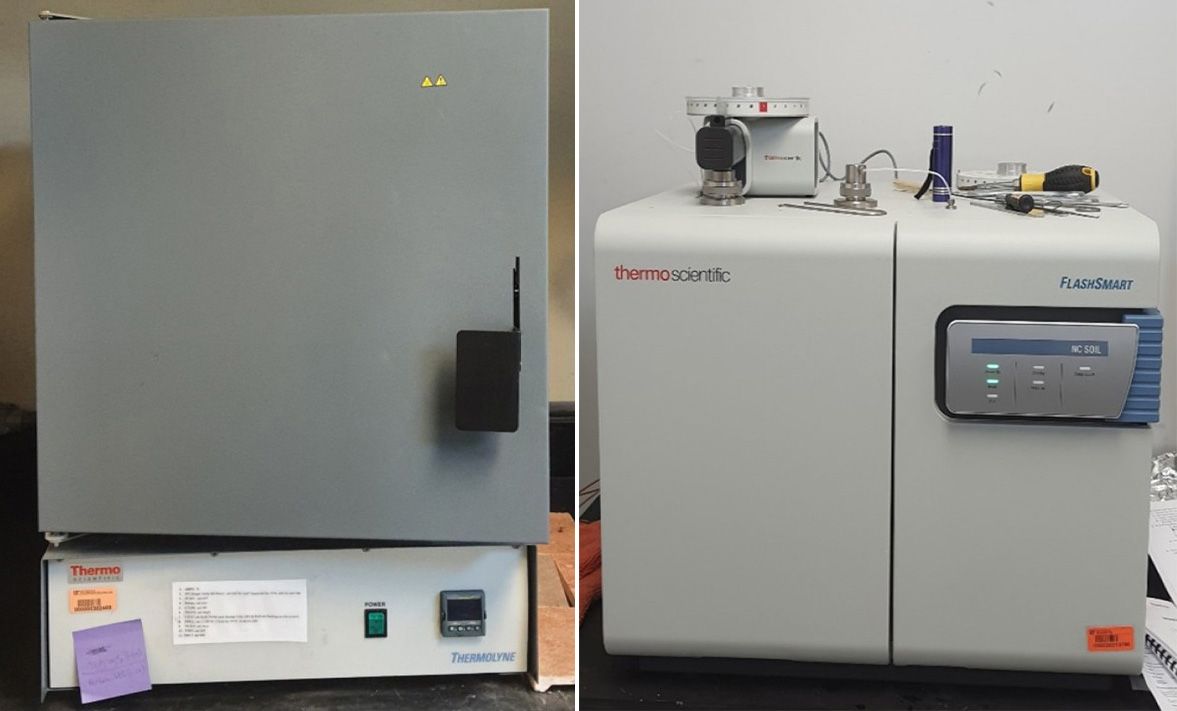
Credit: Suraj Melkani and Jehangir H. Bhadha, UF/IFAS
The wet digestion and dry combustion methods for measuring soil C are accurate but require laboratory facilities, making them time-consuming and expensive. Therefore, there is a need for portable, rapid, accurate, and cost-effective methods to measure soil C, which would benefit researchers who investigate C cycling and global change processes. As a response to these needs, recent developments have led to the use of spectroscopy methods to improve the efficiency of soil C analysis and stock estimation.
Spectroscopic Methods
The spectroscopic methods can be quick and efficient ways to measure and monitor C. These methods can be used for laboratory as well as in-situ measurements via platforms such as aircraft, satellites, and unmanned aerial vehicles. Spectroscopic methods include methods like diffused reflectance spectroscopy, laser-induced breakdown spectroscopy (LIBS), and inelastic neutron scattering (INS).
Diffused Reflectance Spectroscopy Techniques, or Infrared Spectroscopy
This technique works on the diffuse reflectance property of the soil, which depends on the composition of the soil, particle size distribution, organic matter, and soluble salts within the soil (Ben-Dor et. al. 1999). When infrared radiation falls on a sample, it causes electronic transitions in atoms along with vibrational stretching and bending of atomic bonds in crystals (Wetterlind et al. 2010). The amount of C present in the soil can be determined by comparing the sample spectrum to an existing library of spectra (Figure 3).
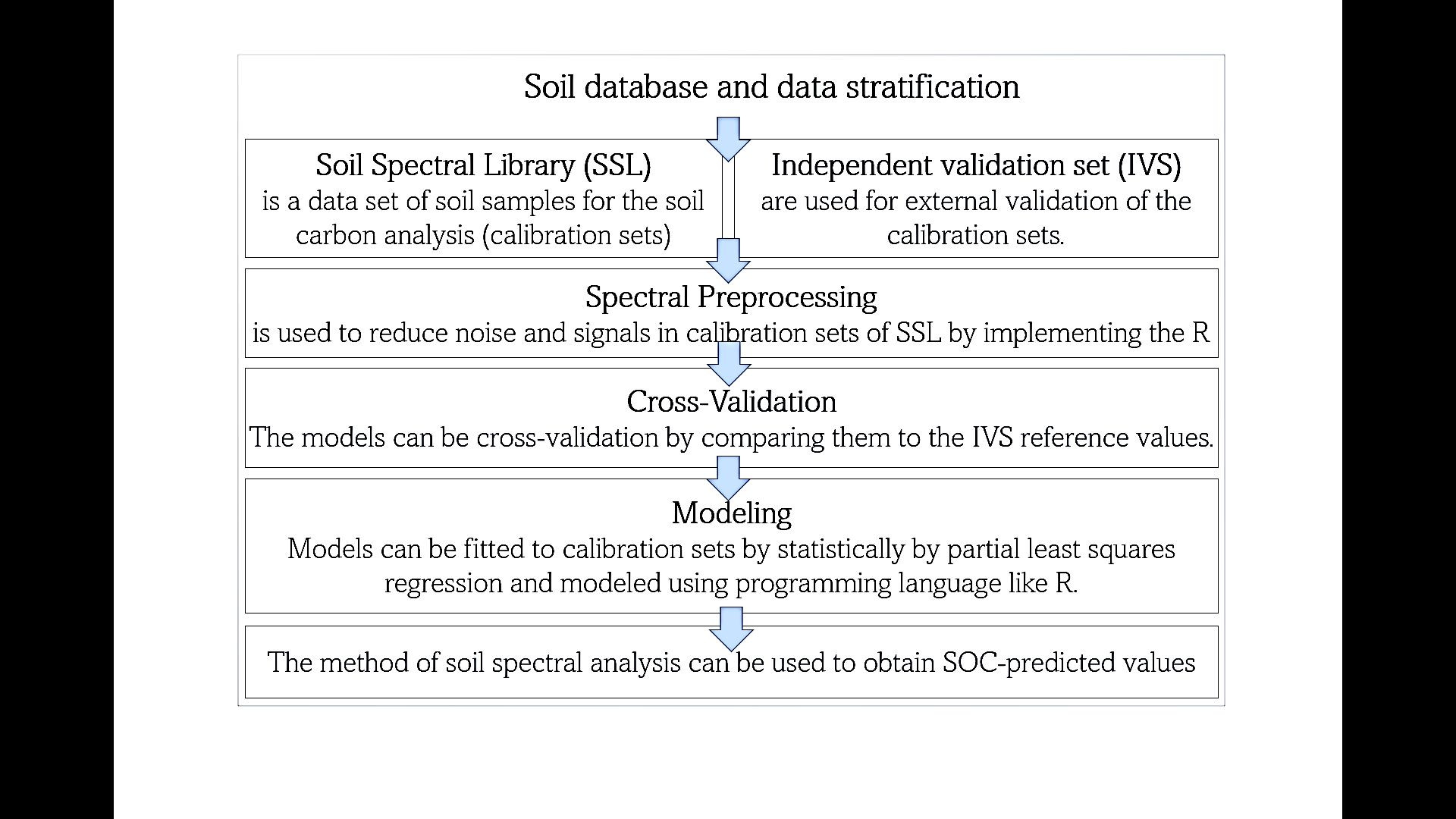
Credit: Suraj Melkani and Jehangir H. Bhadha, UF/IFAS
Depending on the wavelength range of infrared radiation used in diffused reflectance spectroscopy, different structural C properties like C activity, functional groups, aromaticity, arrangement of molecules, etc. can be determined.
Laser-induced Breakdown Spectroscopy (LIBS)
LIBS (Figure 3) is an atomic emission spectroscopy method which uses a high energy laser pulse focused on a soil sample. This causes ionization (i.e., the process of forming ions), excitation (i.e., the process of raising atoms to higher energy levels), evaporation, and atomization (i.e., particle division) of atoms and results in the formation of a high-temperature plasma (Hahn and Omenetto 2010). After cooling, the excited ionic, atomic, and molecular fragments generated within the plasma emit radiations indicative of the elemental composition of the volatilized matter (i.e., matter that has been converted into a vapor or gas state due to high temperature). The individual peaks in the spectrum (Figure 4A) correspond to the elements found in the sample. For C analysis, the strong emission line at the peaks were chosen for calibrating and testing LIBS data with those obtained by laboratory analysis (Figure 4B and 4C). However, there are some known problems with plasma formation as well as unknown problems with how soil properties, such as soil structure and composition, affect LIBS accuracy in estimating C (Senesi and Senesi 2016).
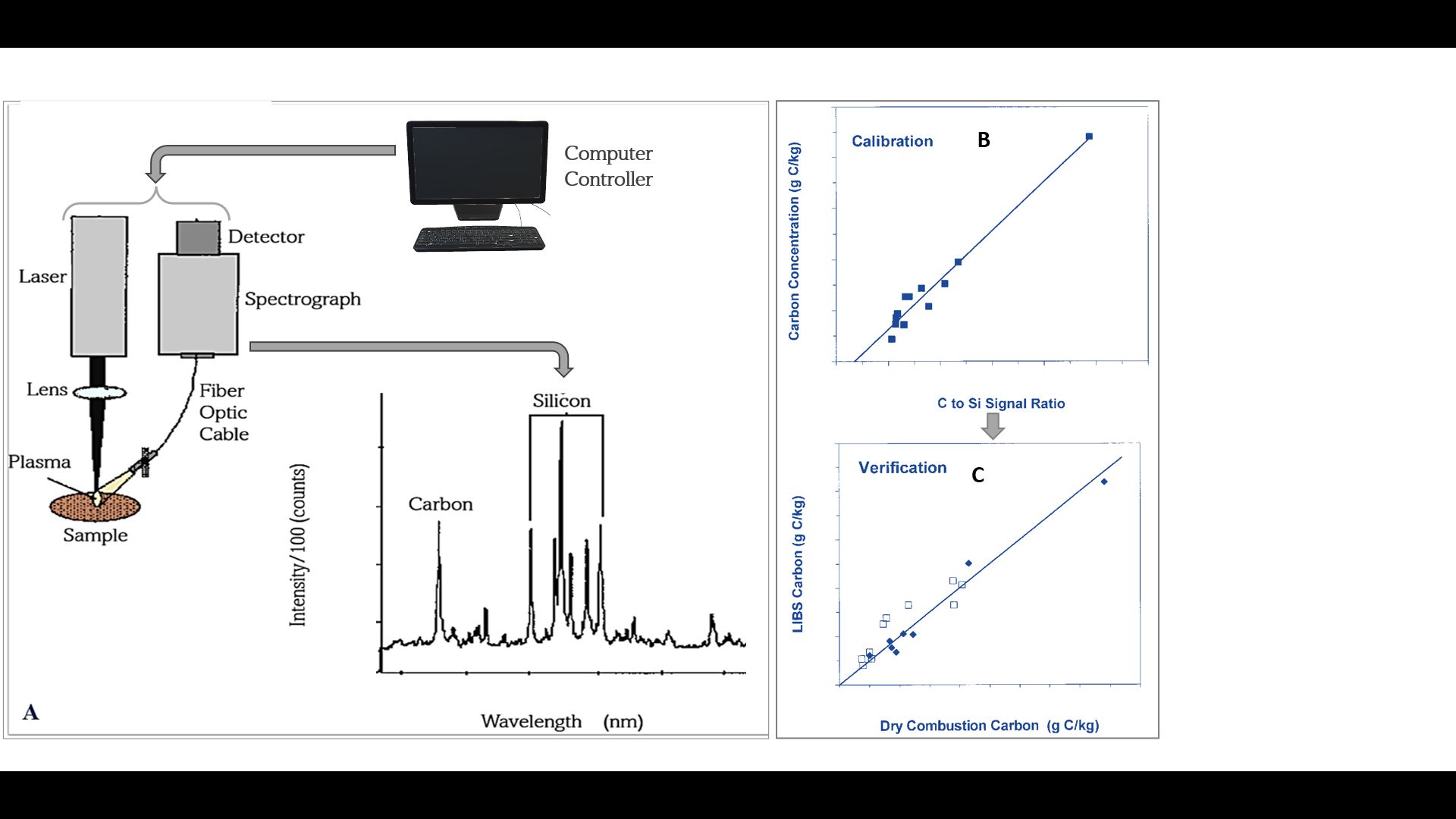
Credit: (A) Adapted from Chatterjee et al. (2009); (B) and (C) adapted from Chatterjee et al. (2009)
Inelastic Neutron Scattering (INS)
Inelastic Neutron Scattering (INS) is a method (Figure 5) that uses a low energy neutron beam to measure C atoms in the soil (Wielopolski and Carayannis 2011). This method can detect both inorganic and organic C by measuring the total number of C atoms present in the scanned area. However, INS faces some challenges, including high costs, lack of optimization and calibration, and the absence of field models ready for research and testing (Nayak et al. 2019). The INS requires further development, such as calibration in larger areas and different soil types, system optimization, and more efficient models to reduce measurement errors.
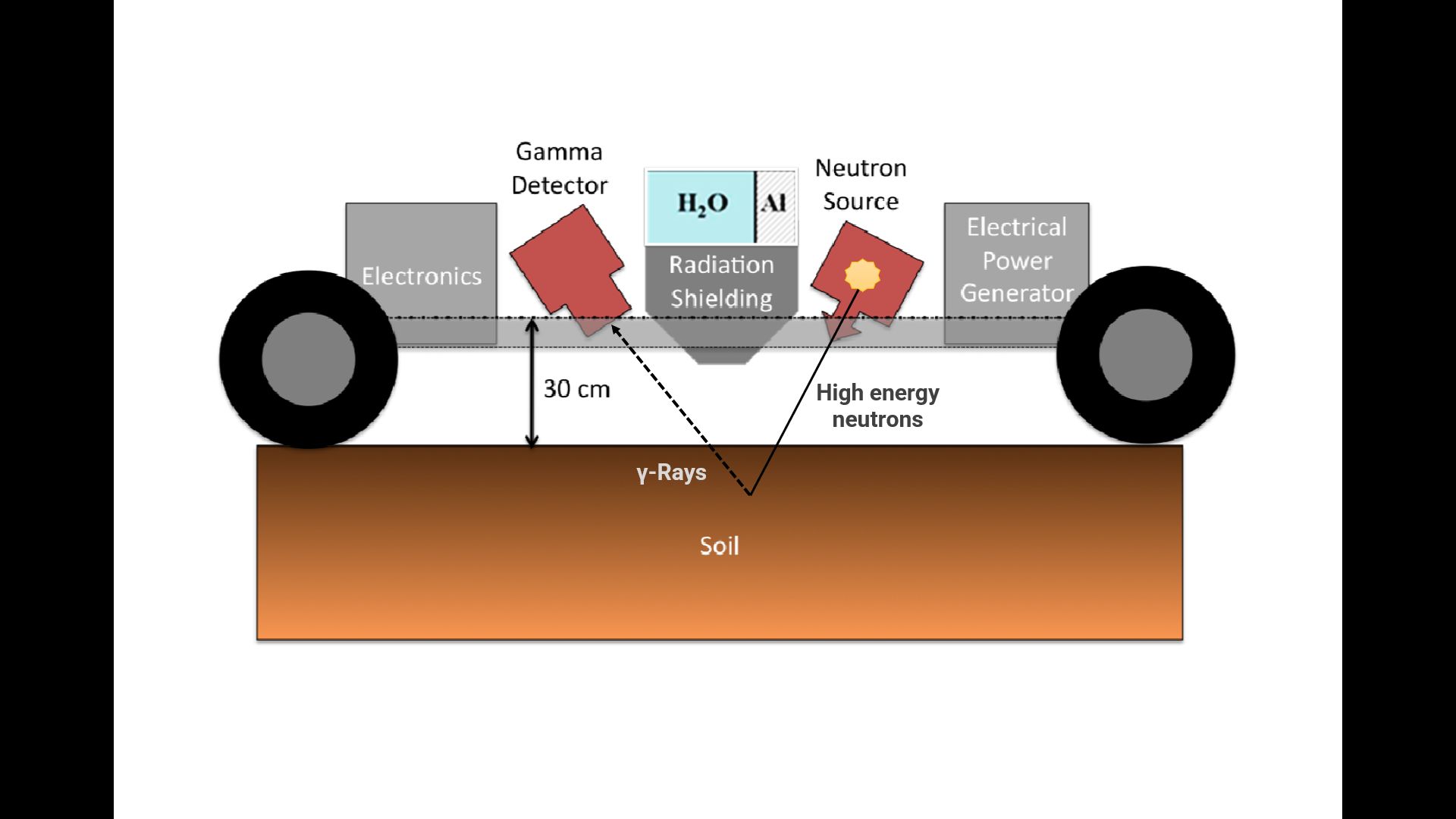
Credit: Adapted from Wielopolski et al. (2008)
Spectroscopic methods can be used for measuring and monitoring C on a field as well as on a regional scale. But, since they are indirect methods for measuring soil C, their current precision is low. Soil C can also be measured on a large scale by directly measuring the ecosystem C exchange.
Direct CO2 Exchange Measurement: Eddy Covariance Approach
An alternative to measuring changes in soil C stocks is to measure all the inputs and output of C at a large geographical scale. Ecosystem CO2 exchange is dominated by photosynthesis and respiration. All these CO2 exchange components can be measured directly with the Eddy covariance flux approach (Figure 6B). This method can effectively measure C and direct, greenhouse gas emissions (Burba et al., 2013).
Eddy covariance measurements of CO2 exchange include that of vertical wind velocity using a sonic anemometer, in addition to measuring fluctuations in CO2 mixing ratio using an open- or closed-path gas analyzer. C flux is commonly used to track long-term changes in land C as well as substantial CO2 fluxes, which refer to the exchange of CO2 between the atmosphere and the earth's surface. They occur due to natural processes and human activities such as burning fossil fuels and deforestation (Nayak et al. 2019).
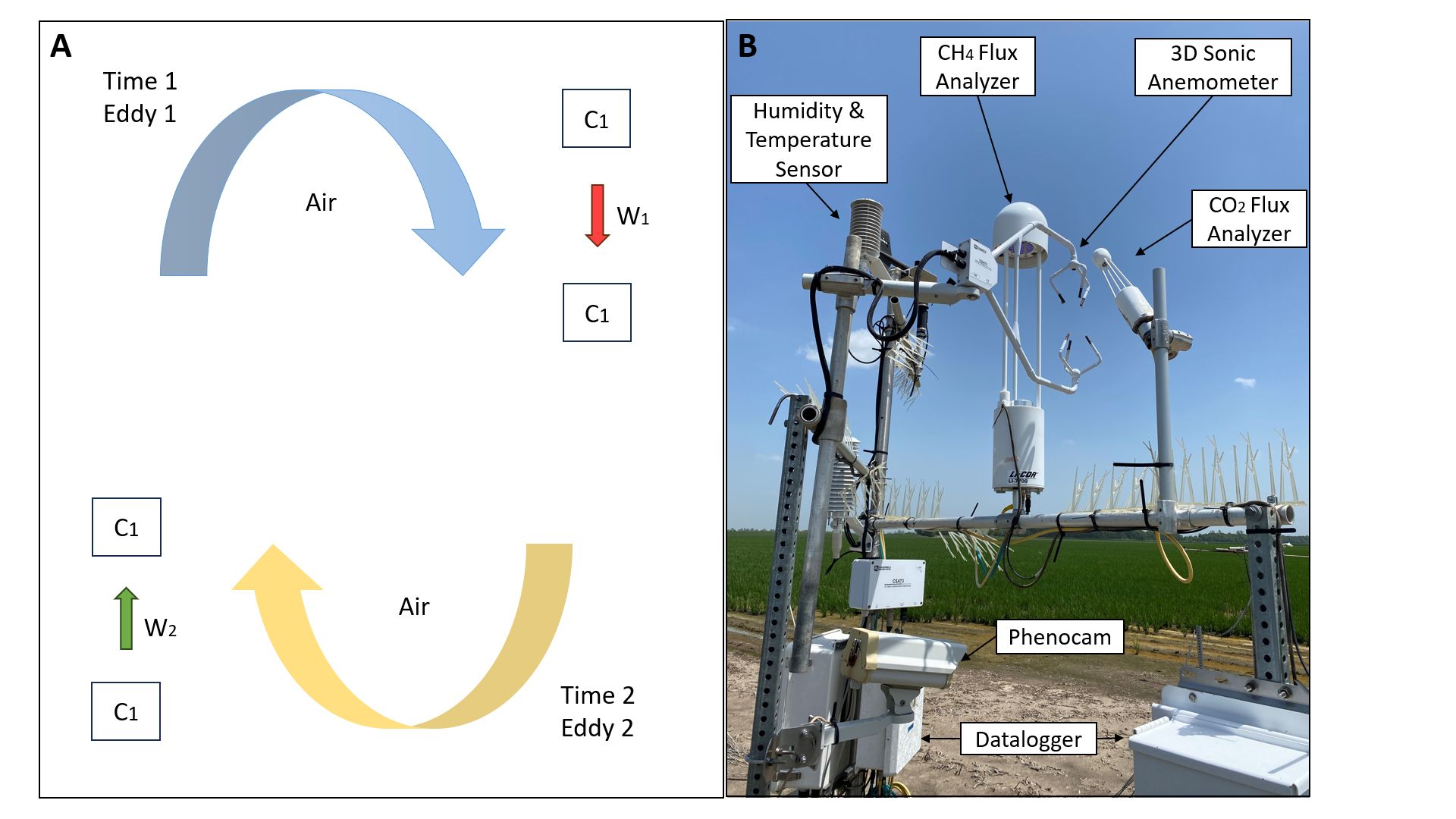
Credit: Suraj Melkani and Jehangir H. Bhadha, UF/IFAS
Challenges with this method of measuring C include inherent uncertainties with the flux estimation and biomass sampling, as well as the proper development of hardware that can rapidly and accurately analyze turbulent flux data (Rinne and Ammann 2012). Eddy covariance approaches also require long-term studies and reduction of uncertainties associated with CO2 flux estimation. On a regional or global scale, C can also be predicted with the use of C models.
Soil C Modelling Approaches
Soil C models can be used to simulate C and estimate soil C budget components such as: (a) CO2 emissions from soil caused by the decomposition of organic C compounds in soil; (b) the C pool soil; and (c) changes in the C pool of soil over time. Soil C models can be classified as empirical models, mechanistic- or process-based models, or meta (fusion) models.
Empirical Models
Empirical models use linked mathematical equations to express conceptual understanding of ecosystem processes.
Mechanistic- or Process-based Models
Process-based models have been established and utilized to simulate biogeochemical processes in the plant-soil-ecosystem (Wang et al. 2017). Table 1 provides information about some process-based models that can effectively simulate soil C.
Table 1. Process-based Models for Soil C Simulation: A Review of Popular Tools.
Meta Models (Fusion Models)
These models combine the features of both empirical and process-based models to achieve greater accuracy. These models are also less complex than process-based models.
The modelling approach requires less time and saves resources used in destructive methods. However, accounting for soil C change using C models needs reliable databases from different agriculture ecosystems. One of the other limitations of process-based models is that they are available for only a few crops and sometimes do not respond to all environmental and management factors. To counter some of the limitations, new techniques for studying C dynamics are emerging.
Emerging Methods
Using C Isotopes to Determine C Decomposition and Turnover Rate
C isotopes can be particularly useful in determining the organic matter decomposition rate and dynamics, rather than actual soil C values in real time. Several methods involve the use of C-13 and C-14 isotopes to determine and measure the decomposition or turnover of C (Balesdent, Wagner, and Mariotti 1988; Paul et al. 1997). The turnover rate of an element in a pool is defined as the balance of the element's inputs (I) and outputs (O) to and from the pool (Six and Jastrow 2002). The obtained decomposition, or turnover value, can be used to calculate soil C:
Soil C = C input – C loss (calculated from turnover rate)
Using Machine Learning for Modeling Soil C Change
Soil C modeling using machine learning is becoming a powerful tool for understanding soil C dynamics. When compared to other modeling approaches, these artificial intelligence generated soil C models perform better in predicting soil C components and other global C cycle properties (Grunwald 2022).
Soil C artificial intelligence models use environmental covariates as inputs, representing different parameters associated with soils, topography, ecology, parent material or lithology, climate, hydrology, organisms, and human activities (Grunwald, Thompson, and Boettinger 2011; Thompson et al. 2012). Table 2 presents commonly applied artificial intelligence algorithms to model soil C.
Table 2. Artificial Intelligence Algorithms for Soil C Modeling.
Artificial intelligence modeling offers sophisticated abilities to estimate C stocks. These artificial intelligence models have potential for avoiding human error at all scales, even though they are currently data-restricted in their ability to explain the spatial variability of soil C storage within landscapes.
Conclusion
Soils have a great capacity to sequester C. However, data on their C storage is limited. Accurate soil C measurements are therefore critical for quantifying soil C pools and inventories, as well as for monitoring the inherent spatial heterogeneity of soil C content and changes that may occur because of various sustainable management practices. Therefore, accurate assessment of soil C quantity would make a significant contribution to assessing disturbance impacts, global climate change, and land use change.
Regardless of the underlying principles, each method has its distinct advantages and limitations. All these methods are effective, but they need to be more accurate at all geographical scales. Therefore, for more efficient quantification of soil C, further research on the existing and emerging methods is required.
References
Angelopoulou, T., N. Tziolas, A. Balafoutis, G. Zalidis, and D. Bochtis. 2019. "Remote Sensing Techniques for Soil Organic Carbon Estimation: A Review.” Remote Sensing. 11(6): 676. https://doi.org/10.3390/rs11060676
Apesteguia, M., A. F. Plante, and I. Virto. 2018. “Methods Assessment for Organic Assessment for Organic and Inorganic Carbon Quantification in Calcareous Soils of the Mediterranean Region.” Geoderma Regional. 12: 39-48. https://doi.org/10.1016/j.geodrs.2017.12.001
Atkins, P.W., and L. Jones. 1991. Principles of Elemental Chemistry. Oxford: Oxford University Press.
Balesdent, J., G. H. Wagner, and A. Mariotti. 1988. “Soil Organic Matter Turnover in Long-term Field Experiments as Revealed by Carbon-13 Natural Abundance.” Soil Science Society of America Journal. 52(1): 118-124. https://doi.org/10.2136/sssaj1988.03615995005200010021x
Ben-Dor, E., J. Irons, and G. Epema. 1999. "Soil Reflectance." In Remote Sensing for the Earth Sciences: Manual of Remote Sensing, edited by N. Rencz. 3: 111-188. New York: Wiley & Sons Inc.
Burba, G., R. Madsen, and K. Feese. 2013. “Eddy Covariance Method for CO2 Emission Measurements in CCUS Application: Principles, Instrumentation, and Software.” Energy Procedia. 40: 329-336. https://doi.org/10.1016/j.egypro.2013.08.038
Chabrillat, S., E. Ben-Dor, J. Cierniewski, C. Gomez, T. Schmid, and B. van Wesemael. 2019. “Imaging Spectroscopy for Soil Mapping and Monitoring.” Surveys in Geophysics. 40(3): 361-399. https://doi.org/10.1007/s10712-019-09524-0
Chatterjee, A., R. Lal., L. Wielopolski, M. Z. Martin, and M. H. Ebinger. 2009. "Evaluation of Different Soil Carbon Determination Methods.” Critical Reviews in Plant Science. 28(3): 164-178. https://doi.org/10.1080/07352680902776556
Ciais, P., C. Sabine, G. Bala, L. Bopp, V. Brovkin, J. Canadell, A. Chhabra, et al. 2014. “Carbon and other Biogeochemical Cycles.” In Climate Change 2013: The Physical Science Basis. Contribution of Working Group I to the Fifth Assessment Report of the Intergovernmental Panel on Climate Change. 465-570. Cambridge University Press. https://doi.org/10.1017/CBO9781107415324.015
Ghani, A., M. Dexter, R. A. Carran, and P. W. Theobald. 2007. “Dissolved Organic Nitrogen and Carbon in Pastoral Soils: The New Zealand Experience.” European Journal of Soil Science. 58(3): 832-843. https://doi.org/10.1111/j.1365-2389.2006.00873.x
Griggs, D. J., and M. Noguer. 2002. “Climate Change 2001: The Scientific Basis. Contribution of Working Group I to the Third Assessment Report of the Intergovernmental Panel on Climate Change.” Weather. 57(8): 267-269. https://doi.org/10.1256/004316502320517344
Grinand, C., B. G. Barthès, D. Brunet, E. Kouakoua, D. Arrouays, C. Jolivet, G. Caria, and M. Bernoux. 2012. “Prediction of Soil Organic and Inorganic Carbon Contents at a National Scale (France) Using Mid-Infrared Reflectance Spectroscopy (MIRS).” European Journal of Soil Science. 63(2): 141-151. https://doi.org/10.1111/j.1365-2389.2012.01429.x
Grunwald, S. 2022. “Artificial Intelligence and Soil Carbon Modeling Demystified: Power, Potentials, and Perils.” Carbon Footprints. 1(1): 5. https://doi.org/10.20517/cf.2022.03
Grunwald, S., J. A. Thompson, and J. L. Boettinger. 2011. “Digital Soil Mapping and Modeling at Continental Scales: Finding Solutions for Global Issues.” Soil Science Society of America Journal. 75(4): 1201-1213. https://doi.org/10.2136/sssaj2011.0025
Hahn, D. W., and N. Omenetto. 2010. “Laser-Induced Breakdown Spectroscopy (LIBS), Part I: Review of Basic Diagnostics and Plasma–Particle Interactions: Still-Challenging Issues within the Analytical Plasma Community”. Applied Spectroscopy, 64(12), 335A-366A. https://doi.org/10.1366/000370210793561691
Harmon, R. S., and G. S. Senesi. 2021. “Laser-Induced Breakdown Spectroscopy–A Geochemical Tool for the 21st Century.” Applied Geochemistry. 128: 104929. https://doi.org/10.1016/j.apgeochem.2021.104929
Lal, R. 2019. “Conceptual Basis of Managing Soil Carbon: Inspired by Nature and Driven by Science.” Journal of Soil and Water Conservation. 74(2): 29a-34a. https://doi.org/10.2489/jswc.74.2.29A
Matus, F. B., V. A. Hermosilla, C. G. Maire, and S. F. Ortega. 1997. “Comparison in the Detemination of Soil Organic Matter by Partial and Complete Oxidation in Different Soils of the VII Region.” Agricultura Technica. 57: 195-199.
Milori, D. M. B. P., H. V. A. Galeti, L. Martin-Neto, J. Dieckow, M. González-Pérez, C. Bayer, and J. Salton. 2006. "Organic Matter Study of Whole Soil Samples Using Laser-Induced Fluorescence Spectroscopy.” Soil Science Society of America Journal. 70(1): 57-63. https://doi.org/10.2136/sssaj2004.0270
Vasques, G. M., S. Grunwald, and D. B. Myers. 2012. “Associations Between Soil Carbon and Ecological Landscape Variables at Escalating Spatial Scales in Florida, USA.” Landscape Ecology. 27: 355-367. https://doi.org/10.1007/s10980-011-9702-3
Nayak, A. K., M. M. Rahman, R. Naidu, B. Dhal, C. Swain, A. Nayak, R. Tripathi, M. Shahid, M. R. Islam, and H. Pathak. 2019. "Current and Emerging Methodologies for Estimating Carbon in Agricultural Soils: A Review.” Science of the Total Environment. 665: 890-912. https://doi.org/10.1016/j.scitotenv.2019.02.125
Neal, R. H., and T. Younglove. 1993. “The Use of a Dry Combustion Infrared Instrumental Technique to Determine Total and Organic Carbon in California Soils.” Communications in Soil Science and Plant Analysis. 24(19-20): 2733-2746. https://doi.org/10.1080/00103629309368992
Nelson, D. W., and L. E. Sommers. 1996. “Total Carbon, Organic Carbon, and Organic Matter.” In Methods of Soil Analysis: Part 3 Chemical Methods 5.3, edited by D. L. Sparks, et al. 961-1010. Soil Science Society of America. https://doi.org/10.2136/sssabookser5.3.c34
Olson, J. S. 1963. “Energy Storage and the Balance of Producers and Decomposers in Ecological Systems.” Ecology. 44(2): 322-331. https://doi.org/10.2307/1932179
Paul, E. A., R. F. Follett, S. W. Leavitt, A. Halvorson, G. A. Peterson, and D. J. Lyon. 1997. “Radiocarbon Dating for Determination of Soil Organic Matter Pool Sizes and Dynamics.” Soil Science Society of America Journal. 61(4): 1058-1067. https://doi.org/10.2136/sssaj1997.03615995006100040011x
Rinne, J., and C. Ammann. 2012. “Disjunct Eddy Covariance Method.” In Eddy Covariance, edited by M. Aubinet, T. Vesala, D. Papale. 291-307. Springer Atmospheric Sciences. Springer, Dordrecht. https://doi.org/10.1007/978-94-007-2351-1_10
Schulte, E. E., C. Kaufmann, and J. B. Peter. 1991. “The Influence of Sample Size and Heating Time on Soil Weight Loss-on-Ignition.” Communications in Soil Science and Plant Analysis. 22(1-2): 159-168. https://doi.org/10.1080/00103629109368402
Senesi, G. S., and N. Senesi. 2016. “Laser-Induced Breakdown Spectroscopy (LIBS) to Measure Quantitatively Soil Carbon with Emphasis on Soil Organic Carbon. A Review.” Analytica Chimica Acta. 938: 7-17. https://doi.org/10.1016/j.aca.2016.07.039
Six, J., & Jastrow, J. D. (2002). “Organic Matter Turnover.” In Encyclopedia of Soil Science, 2nd ed., edited by R. Lal. 1210-1215.
Thompson, J. A., S. Roecker, S. Grunwald, and P. R. Owens. 2012. “Chapter 21 - Digital Soil Mapping: Interactions with and Applications for Hydropedology.” In Hydropedology, edited by H. Lin. 665-709. Academic Press. https://doi.org/10.1016/B978-0-12-386941-8.00021-6
Walkley, A., and I. A. Black. 1934. “An Examination of the Degtjareff Method for Determining Soil Organic Matter, and a Proposed Modification of the Chromic Acid Titration Method.” Soil Science. 37(1): 29-38. https://doi.org/10.1097/00010694-193401000-00003
Wang, G., W. Zhang, W. Sun, T. Li, and P. Han. 2017. “Modeling Soil Organic Carbon Dynamics and Their Driving Factors in the Main Global Cereal Cropping Systems.” Atmospheric Chemistry and Physics. 17(19): 11849-11859. https://doi.org/10.5194/acp-17-11849-2017
Wang, S., K. Guan, C. Zhang, D. Lee, A. J. Margenot, Y. Ge, J. Peng, W. Zhou, Q. Zhou, & Y. Huang. 2022. “Using Soil Library Hyperspectral Reflectance and Machine Learning to Predict Soil Organic Carbon: Assessing Potential of Airborne and Spaceborne Optical Soil Sensing.” Remote Sensing of Environment. 271: 112914. https://doi.org/10.1016/j.rse.2022.112914
Wetterlind, J., B. Stenberg, and M. Söderström. 2010. “Increased Sample Point Density in Farm Soil Mapping by Local Calibration of Visible and Near Infrared Predication Models.” Geoderma. 156(3-4): 152-160. https://doi.org/10.1016/j.geoderma.2010.02.012
Wielopolski, L., and E. Carayannis. 2011. “Nuclear Methodology for Non-Destructive Multi-Elemental Analysis of Large Volumes of Soil.” In Planet Earth, edited by E. G. Carayannis. 467-492. https://doi.org/10.5772/23230
Wielopolski, L., G. Hendrey, K. H. Johnsen, S. Mitra, S. A. Prior, H. H. Rogers, and H. A. Torbert. 2008. “Nondestructive System for Analyzing Carbon in the Soil.” Soil Science Society of America Journal. 72(5): 1269-1277. https://doi.org/10.2136/sssaj2007.0177
Wielopolski, L., S. Mitra, and O. Doron. 2008. “Non-Carbon-Based Compact Shadow Shielding for 14 MeV Neutrons.” Journal of Radioanalytical and Nuclear Chemistry. 276(1): 179-182. https://doi.org/10.1007/s10967-007-0429-1
Zhang, Z., and J. C. Moore. (2014). Mathematical and Physical Fundamentals of Climate Change. Elsevier.