Lysimeters are often used in research concerning nutrient leachate all over the globe. However, due to factors such as Florida's high water table (a condition when the water level stays relatively close to the soil surface) in some production areas, the assessment and collection of leachates are not feasible. Another obstacle to the use of lysimeters in field research is the fact that several types of irrigation methods are used in Florida (e.g., subirrigation with seepage or drain-tile, overhead, micro sprinkler, and drip irrigation) requiring different strategies for leachate assessment. This publication focuses on the use of crop models as alternatives to estimate nitrate leaching from cropping systems at a field scale. This publication's target audience is growers, Extension agents, crop consultants, representatives of the fertilizer industry, state and local agencies, students, instructors, researchers, and interested Florida citizens.
Background
An agroecosystem consists of several interdependent components such as soil, weather, crops, cultivars, hybrids or varieties, pests, diseases, and weeds. Mathematical models may help to represent the many interactions inside such systems. The models include mathematical algorithms that gather quantitative data about the agronomic environment to explain and estimate plant growth and yield, as well as water and soil dynamics (Asseng et al. 2014). They can ultimately be used to guide producers in deciding the best management practices based on different options that consider different scenarios or climatic patterns (Tsuji, Hoogenboom, and Thornton 1998).
The high water table in Florida significantly impacts the state's agriculture industry, which annually generates billions of dollars in economic activity (USDA-NASS 2024). In 2021, the National Agricultural Statistics Service of the United States Department of Agriculture (USDA-NASS) predicted that the total value of Florida's agricultural commodities was approximately $7.64 billion. Farmers who rely on irrigation to grow their crops in Florida may have challenges due to the state's high water table. Depending on the location, the natural water table depth in Florida ranges from 5 to 20 feet below the surface (Ryan and Hazelbaker 2022). This might be troublesome for farmers who use seepage irrigation to irrigate their crops. In this method, groundwater is pumped and distributed on the field via furrows so that water seeps slowly into the ground. Keeping the water table level closer to the crop rootzone can lead to waterlogging of the field and other related issues (Ryan and Hazelbaker 2022). During periods of intense rainfall, which are necessary for leachate studies, the water table may rise close to the surface of the soil, potentially inducing groundwater to enter the lysimeters. Since it may be difficult to determine whether the leachate originated from the soil surface or groundwater, this is sometimes the sole piece of information provided in the data. The presence of a high water table in most cultivated areas limits the use of lysimeters to areas with well-drained soil profiles. In those areas, the placement of the lysimeter is crucial since it has the capacity to affect both the quality and quantity of the collected leachate. Furthermore, the positioning of the lysimeters in relation to the crop rows and irrigation systems provides accurate measurements of water, and nutrient movement through the soil can impact the accuracy of the measurements as well (Santos et al. 2023; Song et al. 2022). In addition, the cost of the lysimeter (~$5,000–$8,000 [Drain Gauge – G3 lysimeter]) and leachate analysis are expensive and time consuming. More recently, research funding organizations in Florida have required researchers and Extension agents to gather water quality data to evaluate the impact of agricultural best management practices. Thus, alternative methods to assess nutrient leaching from agricultural systems are needed.
What are the potential solutions to substitute lysimeters use?
Soil nutrient research must continue to innovate and adapt its techniques to suit the demands of this unique environment. At the same time, these techniques need to address the financial restrictions that might make implementation difficult. Crop models are a valuable tool that can be used to understand how management will impact the system without having to implement trials to account for every interaction. Crop models can be used to understand how crop management will impact the environment in several different ways. Following a thorough evaluation, crop models can be used to predict these interactions just by requiring a minimal data collection to run the model, as well as quality data to calibrate and verify the simulation of certain circumstances. The bare minimum dataset will include information regarding the soil properties (e.g., profile, texture, and chemical analysis), information regarding the weather (e.g., solar radiation, rainfall, maximum and minimum temperature), and information regarding crop management (e.g., cultivar or hybrid, irrigation, fertilizer, planting date, spacing, tillage operations, etc.) (Arnold et al. 2012; Holzworth et al. 2014; Hoogenboom et al. 2012).
The following information is required for most model evaluations and estimations of nutrient leaching.
- Crop measurements such as yield and biomass at regular time intervals.
- Crop phenology (e.g., emergence, flowering, physiological maturity, harvest maturity, first seed).
The information above could be used to test if the model can capture the effect of specific management on plant growth and production (Arnold et al. 2012; Holzworth et al. 2014; Hoogenboom et al. 2019). Considering that the crop model presents a good estimation of crop production, it can be used to simulate and test the effects of different management practices on a system to evaluate their impacts on crop growth, yield, environment, and economics considering, not just one season of weather data, but multiple years to account for the annual weather variability (Woli, Hoogenboom, and Alva 2016; Salmerón et al. 2014; Nijbroek, Hoogenboom, and Jones 2003; Tsuji, Hoogenboom, and Thornton 1998).
Several models are available and can be used for crop and soil dynamics simulation. Of the available computer software for crop simulation, the Decision Support System for Agrotechnology Transfer (DSSAT; www.dssat.net) has already been applied in Florida to combine different management options as input to simulate nitrate leaching responses.
Decision Support System for Agrotechnology Transfer (DSSAT)
The DSSAT is a software application program that comprises crop simulations for more than 42 crops and has a user-friendly computational interface with tools to facilitate the effective use and application of the models (Hoogenboom et al. 2021; Hoogenboom et al. 2019). The DSSAT software allows running single-season experiments, crop rotation systems, and spatial analysis to account for weather variation. The user can combine practices such as fertilizer inputs, irrigation methods, and rotation systems. Many different simulation outputs can be assessed to test the management's efficiency (Jones et al. 2003). The DSSAT is a powerful tool that integrates weather, soil, crop management, and crop yield data to simulate crop growth, estimate crop yield, and analyze the impact of different management practices on crop performance. To accurately predict crop performance, a minimum dataset is required to input into the DSSAT system (Hoogenboom et al. 2021; Hoogenboom et al. 2019).
- Weather data include daily maximum and minimum temperature, rainfall, and solar radiation. The weather data in Florida can be obtained from the Florida Automated Weather Network (www.fawn.ifas.ufl.edu).
- Soil surface and profile data, such as soil texture, bulk density, and soil organic matter content, are also required. If local soil information is not available, general soil information can be retrieved from Natural Resources Conservation Service (NRCS) – Web Soil Service (https://websoilsurvey.nrcs.usda.gov/app/).
- Crop management data include planting date, planting density, and irrigation and fertilizer application rates and dates.
Who to Contact to Learn about DSSAT
Dr. Gerrit Hoogenboom is the point of contact and development coordinator of the DSSAT, which is one of the most popular crop modeling systems worldwide. He is a preeminent scholar in the Global Food Systems Institute and a professor of Agricultural and Biological Engineering at the University of Florida Institute of Food and Agricultural Sciences. Prior to this institution, he was the director of the AgWeatherNet Program and professor of Agrometeorology at Washington State University. Dr. Hoogenboom has over 30 years of experience in the development of crop simulation models and decision support systems, and he frequently organizes and facilitates international training workshops on the topics.
Example of using DSSAT and Results
In Figure 1, DSSAT was used to estimate nitrate leaching for different levels of N fertilizer (low, medium, and high) and irrigation methods (calendar-based and soil moisture sensor-based) for a corn-peanut rotation in the Suwannee Valley, Florida. The results presented in this plot show which irrigation method or fertilizer application result in higher nitrate losses (e.g., nitrate moving below the crop rootzone), guiding the decision-making process of choosing the best management practice.
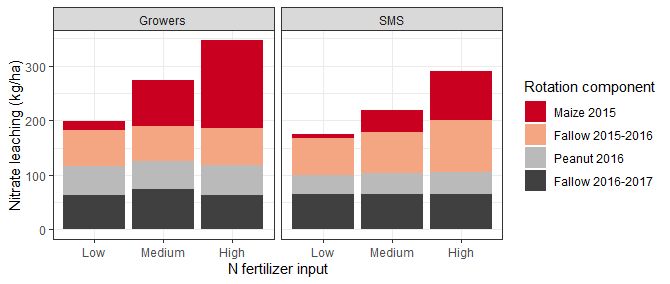
Credit: Adapted from Zamora-Re et al. (2020) with permission
In Figure 2, we used the SUBSTOR-Potato model of DSSAT to simulate the rainfall-induced uncertainty on the nitrate leaching from a 2011–2020 period with different timings of application and rates of N fertilizer (Low: 0, 56, and 56 kg/ha applied at planting (PL), emergence (EM), and tuber-initiation (TI) stages, respectively; Medium: 0, 112, and 56 kg/ha at PL, EM, and TI; High: 56, 112, and 56 kg/ha at PL, EM, and TI). Simulations were conducted for a sub-irrigated potato cropping system in Hastings, Florida. The results presented in Figure 2 show that rainfall conditions can greatly affect the N leaching even when lower N fertilizer rates are used. These examples of research combined with a crop modeling approach could provide guidance to growers in the decision-making process of choosing the appropriate N rates for a given rainfall condition without a reduction in tuber yield.
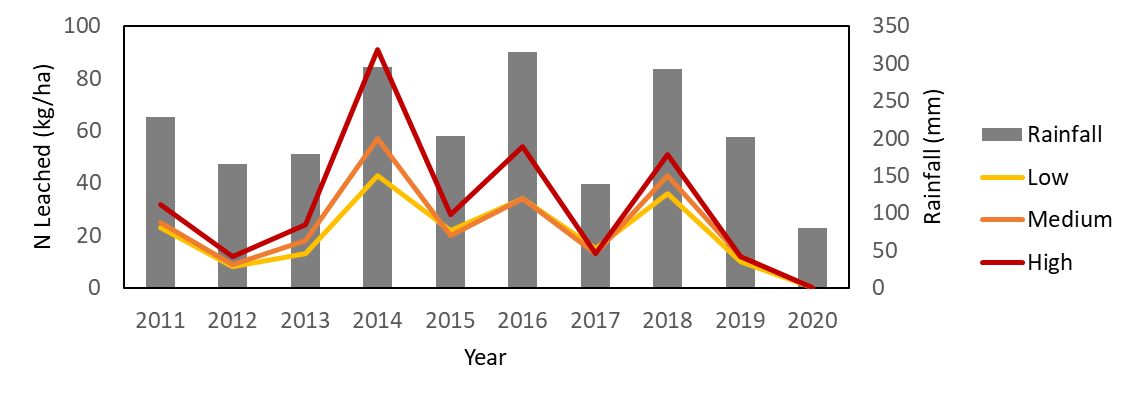
Credit: Adapted from Zamora-Re et al. (2020) with permission
Future Implications
The issues confronting agriculture in Florida and other parts of the globe are anticipated to continue changing in the future. Crop yield, environmental impact, and resource use are projected to be significantly affected by climate change, population increase, and shifting consumer needs. Consequently, the development of new tools and technologies, such as crop modeling, artificial intelligence/machine learning, and associated decision support systems, may increase our capacity to manage water resources and improve agricultural operations.
Moreover, the use of minimum datasets, mathematical modeling, and advanced analytical tools like DSSAT will continue to be essential for effective water resource management. These technologies can help ensure that agriculture can continue to be a viable industry while minimizing its environmental impact.
While the challenges facing agriculture in Florida and other regions may seem daunting, it is possible to overcome them by adopting innovative solutions that are built on data-driven approaches and show potential tradeoffs related to maximum economic returns, optimizing yield, and minimizing environmental impact. By doing so, we can ensure that agriculture can continue to thrive while preserving the environment for future generations.
Conclusion
Challenges, such as a high water table, seepage irrigation, nutrient leaching, and wide row plantations of tomatoes, potatoes, and other crops, require a proactive approach to managing water resources. Expensive water sampling and labor-intensive processes can be mitigated by employing new technologies and methodologies. Using crop modeling to estimate nitrate leaching through soil sampling, representing phenomena by mathematical equations, and adopting minimum datasets can help to improve the accuracy and efficiency of water resource management. Model evaluation and application, including tools such as DSSAT, can aid in identifying areas of improvement and optimize land use and management practices. With these solutions, it is possible to mitigate the impact of agriculture on the environment and ensure sustainable practices for future generations.
References
Arnold, J., D. Moriasi, P. Gassman, K. Abbaspour, M. White, R. Srinivasan, C. Santhi, et al. 2012. “SWAT: Model Use, Calibration, and Validation.” Transactions of the ASABE 55 (4): 1491–1508. https://doi.org/10.13031/2013.42256
Asseng, S., Y. Zhu, B. Basso, T. Wilson, and D. Cammarano. 2014. “Simulation Modeling: Applications in Cropping Systems.” In Encyclopedia of Agriculture and Food Systems, edited by N. K. Van Alfen, 102–112. Cambridge, Massachusetts: Academic Press. https://doi.org/doi.org/10.1016/B978-0-444-52512-3.00233-3
Holzworth, D. P., N. I. Huth, P. G. deVoil, E. J. Zurcher, N. I. Herrmann, G. McLean, K. Chenu, et al. 2014. “APSIM - Evolution towards a New Generation of Agricultural Systems Simulation.” Environmental Modelling and Software 62: 327–350. https://doi.org/10.1016/j.envsoft.2014.07.009
Hoogenboom, G., J. W. Jones, P. C. S. Traore, and K. J. Boote. 2012. “Experiments and Data for Model Evaluation and Application.” In Improving Soil Fertility Recommendations in Africa using the Decision Support Systems for Agrotechnology Transfers (DSSAT), edited by J. Kihara, D. Fatondji, J. W. Jones, G. Hoogenboom, R. Tabo, and A. Bationo, 9–18. Dordrecht, the Netherlands: Springer.
Hoogenboom, G., C. H. Porter, K. J. Boote, V. Shelia, P. W. Wilkens, U. Singh, J. W. White, et al. 2019. “The DSSAT Crop Modeling Eecosystem.” In Advances in Crop Modelling for a Sustainable Agriculture, edited by K. J. Boote, 173–216. Cambridge, UK: Burleigh Dodds Science Publishing. https://doi.org/10.19103/AS.2019.0061.10
Hoogenboom, G., C. Porter, V. Shelia, K. J. Boote, U. Singh, J. W. White, W. Pavan, et al. 2021. Decision Support System for Agrotechnology Transfer - v. 4.8. DSSAT Foundation. www.DSSAT.net
Jones, J. W., G. Hoogenboom, C. H. Porter, K. J. Boote, W. D. Batchelor, L. A. Hunt, P. W. Wilkens, U. Singh, A. J. Gijsman, and J. T. Ritchie. 2003. “The DSSAT Cropping System Model.” In European Journal of Agronomy 18 (3–4): 235–265. https://doi.org/10.1016/S1161-0301(02)00107-7
Ni, X., P. B. Parajuli, Y. Ouyang, P. Dash, and C. Siegert. 2021. “Assessing Land Use Change Impact on Stream Discharge and Stream Water Quality in an Agricultural Watershed.” CATENA 198: 105055. https://doi.org/10.1016/j.catena.2020.105055
Nijbroek, R., G. Hoogenboom, and J. W. Jones. 2003. “Optimal Irrigation Strategy for a Spatially Variable Soybean Field: A Modeling Approach.” Agricultural Systems 76 (1): 359–377. https://doi.org/10.1016/S0308-521X(02)00127-0
Ryan, P. J., & C. L. Hazelbaker. 2022. "Comparison of Computed Flow through Manually Operated Water Control Structures in Florida using Theoretical Versus Calibrated Coefficients, Open File Report 2022-1011. US Geological Survey." https://doi.org/10.3133/ofr20221011
Salmerón, M., J. Cavero, R. Isla, C. H. Porter, J. W. Jones, K. J. Boote. 2014. “DSSAT Nitrogen Cycle Simulation of Cover Crop-maize Rotations Under Irrigated Mediterranean Conditions.” Agronomy Journal 106 (4): 1283–1296. https://doi.org/10.2134/agronj13.0560
Santos, E. R. S., J. C. B. Dubeux Jr., C. Mackowiak, D. Wright, and G. Anguelov. 2023. “Integrated crop‐livestock systems result in less nitrate leaching than ungrazed crop systems in North Florida.” Journal of Environmental Quality 52 (4): 847–858. https://doi.org/10.1002/jeq2.20474
Song, J. H., Y. Her, X. Yu, Y. Li, A. Smyth, and W. Martens-Habbena. 2022. “Effect of Information-driven Irrigation Scheduling on Water Use Efficiency, Nutrient Leaching, Greenhouse Gas Emission, and Plant Growth in South Florida.” Agriculture, Ecosystems & Environment 333: 107954. https://doi.org/10.1016/j.agee.2022.107954
Tsuji, G. Y., G. Hoogenboom, and P. K. Thornton, eds. 1998. Understanding Options for Agricultural Production. Systems Approaches for Sustainable Agricultural Development, Vol. 7. Dordrecht, the Netherlands: Kluwer Academic Publishers.
USDA-NASS. 2024. Florida Agriculture by the Numbers 2022. Florida Department of Agriculture and Consumer Services. https://ccmedia.fdacs.gov/content/download/114369/file/Florida-Ag-by-the-Numbers-2022-Final.pdf
Woli, P., G. Hoogenboom, and A. Alva. 2016. “Simulation of Potato Yield, Nitrate Leaching, and Profit Margins as Influenced by Irrigation and Nitrogen Management in Different Soils and Production Regions.” Agricultural Water Management 171: 120–130. https://doi.org/10.1016/j.agwat.2016.04.003
Zamora-Re, M. I., S. Rath, M. D. Dukes, and W. D. Graham. 2020. “Water and Nitrogen Budget Dynamics for a Maize-peanut Rotation in Florida.” Transactions of the ASABE 63 (6): 2003–2020. https://dx.doi.org/10.13031/TRANS.13916