Introduction
The concept of networks has become more widely discussed in recent years (evidenced by discussion pertaining to social media as well as the six degrees of separation theory). Networks provide a valuable lens that can be used to explore various individual and organizational outcomes (Brass et al., 2004; Stevenson & Greenberg, 2000). An understanding of networks and social network research may benefit Extension professionals when conducting or interpreting research related to a practitioner's community or field of specialization. This publication discusses the major concepts and terms associated with social network research so that Extension professionals and others who utilize social science research may be better equipped to comprehend studies that have used this methodology. This document may be used as a reference for individuals who are new to this methodology yet want to understand research incorporating this approach.
Network, Nodes, and Relationships
Social network analysis is frequently confused with social media networking, but the two terms are very different. Brass et al. (2004) defined networks "as a set of nodes and the set of ties representing some relationship, or lack of relationship, between the nodes" (p. 795). The structure assumed by nodes and ties is defined as the Social Structure, where individual actors' behaviors within the network are influenced by attributes of an actor and by the net of relations in which the actor is embedded (Vera & Schupp, 2006). For example, an Extension client who wants to start an urban community garden in their area might make a decision to establish a garden based on views of other members in the community. In this example, the client represents one node and the relationships with others in the community represent ties to other nodes. The Extension client may be influenced by people they know who also have gardens or who are of a similar age (attributes) or by their central position within a group of gardeners (structure).
Nodes (or actors in the network) make up the unit of analysis and may be individuals, groups of individuals, the entire organization, or even countries (Zack, 2000). Ties (or relationships) define the connections between the nodes/actors (Wasserman & Faust, 1994), and these connections can be friendship, advice, social support, or common stakeholders for two programs (Kumar Chaudhary, 2014; Williams, 2005). For example, we could study the reach of Extension in the community by examining the networks of Extension professionals and their clientele. The ties between two nodes/actors can be either formal or informal (Provan et al., 2007). An example of an informal tie would be friendship, and an example of a formal tie would be a formal contract developed by two Extension clients starting a business together defining their work responsibilities and shared profit.
Not all nodes are connected to each other in a network, which means some networks are very dense (i.e., more connected) and some sparse (i.e., less connected). Based on how we collect data and analyze the networks, the networks can be approached as sociocentric (whole network) (Figure 1) or egocentric (personal network) (Figure 2). In the whole-network approach, connections (ties) among all existing pairs of actors in the network are collected. With data for whole network, we can extract the subgraph from the whole network representing the connections of an individual actor, which is also called an ego network. Personal-network data also can be collected by asking every single actor who they are connected to (such as through seeking advice, friendship, etc.) (Borgatti et al., 2013).

Credit: Adapted from McCarty and Vacca (2013)
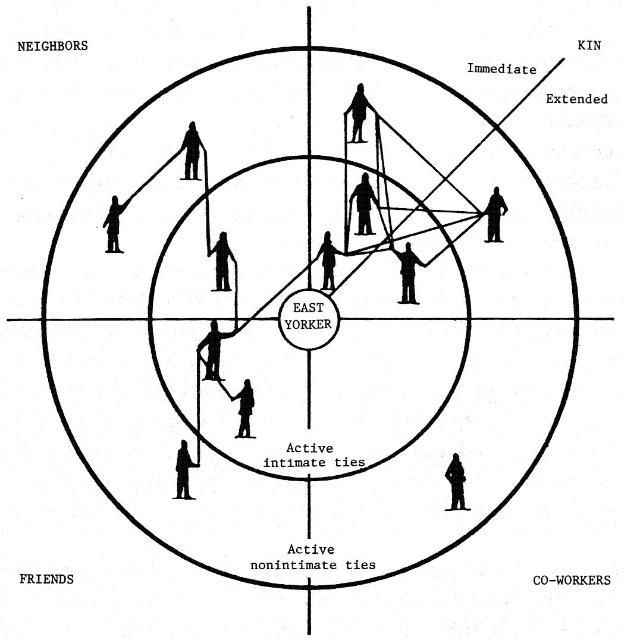
Credit: Adapted from Wellman and Berkowitz (1988, p. 27)
The following summarizes some key terms that are commonly used in social network research.
Network Variables
Network Centrality
Network centrality refers to how resourceful or influential an actor is in the network compared to other actors in the network (Brass & Burkhardt, 1992; Brass et al., 2004). A central position may provide various advantages to the central actor such as more access to information and resources (Brass & Burkhardt, 1992; Brass et al., 2004; Freeman, 1979; Stevenson & Greenberg, 2000; Tsai, 2001). For example, in the case of Extension stakeholders a local leader would be considered a central person in the network. Occupying a central position is not always beneficial, depending on the kind of network (what are the nodes and ties) and the kind of centrality. For example, in a network analysis of citrus growers, if we found that a very central farm is a source of citrus greening, then occupying this central position would not be considered beneficial. Based on different outcomes associated with centrality, it can be defined in a variety of ways. The most common classification of centrality was provided by Freeman (1979), where centrality was classified into Degree, Closeness, and Betweenness.
Degree Centrality
This measure defines the central actor by its direct connections to all other actors in the network and is measured by counting how many actors are directly connected to the central actor. A high degree of centrality of an actor means that a central actor has more options to access resources or information (Brass & Burkhardt, 1992; Freeman, 1979). The degree can be directed or undirected, and this differentiates degree centrality into in-degree and out-degree. For example, an Extension educator who provides advice to all other members in the county office has the highest in-degree centrality because all other members come to the educator for advice. This educator also has the lowest out-degree centrality because the educator is not seeking advice from others.
Closeness Centrality
This measure defines the reach or closeness of an actor to all other actors in the network; this reach can be through direct and indirect connections of an actor. Based on closeness, the central actor has an advantage in spreading the message to all other actors in the shortest amount of time due to their direct and indirect connections. (Brass & Burkhardt, 1992; Freeman, 1979). For example, Extension clients who have easy access to all other clients in the local community have the highest closeness centrality.
Betweenness Centrality
This centrality measure can be defined as how frequently an actor falls on the shortest path between two other actors in the network. This central position provides more control to the central actor by mediating the flow of information and resources between two other actors and also the ability to synthesize information from different parts of the network. (Brass & Burkhardt, 1992; Freeman, 1979). For example, an Extension client who connects two disconnected groups of people in a local community acts as a link between the two groups and has the highest betweenness centrality.
Other Centrality Measures
Eigenvector Centrality
This measure is the variation of degree centrality where direct connections of an actor are weighted by their degree centralities (Bonacich, 2007; Borgatti, 2005). For example, an Extension client who is connected to many other influential members in the community has a high eigenvector centrality.
Brokerage
Brokerage can be defined as occupancy of a position by an actor (black circle) in the network which connects two other actors (two white circles) who have no direct connection to each other (Figure 3) (Burt, 1992; Gould & Fernandez, 1989).

Credit: Adapted from Kumar Chaudhary (2014, p. 24)
Brokerage also can be characterized as "(a) … bridg[ing] a gap in social structure and (b) … help[ing] goods, information, opportunities, or knowledge flow across that gap" (Stovel & Shaw, 2013, p.141). The actor who occupies the broker position has the advantage of access to information and resources from both actors whom the broker is connecting (Burt, 1992; Yin et al., 2012). One example of brokerage occurs with the leader in a community who connects otherwise unconnected Extension professionals and Extension stakeholders. Further discussion on the concept of brokerage is presented in Introduction to Social Network Research: Brokerage Typology (https://edis.ifas.ufl.edu/publication/WC197).
Network Density
Network density is defined as the ratio of the number of existing ties in the network to the maximum possible number of ties if all the actors/ individuals were connected to all other individuals in the network (Scott, 1988). In Figure 4 there are six actors in the network and the network has seven ties. If all six actors were connected to all other actors in the network there would be a maximum of 15 possible ties in the network [n*(n–1)/2; n = number of actors in the network]. The density of the network is 7/15= 0.47, indicating that slightly less than half of the maximum number of ties that are utilized in the network and there is still a possibility to increase the number of ties to take full advantage of the network. In practical situations maintaining 100% of possible ties is not feasible due to various factors. One caution on network density is that that not all situations benefit from a completely connected network. For example, innovation in an organization typically may come from interaction between mostly isolated groups.
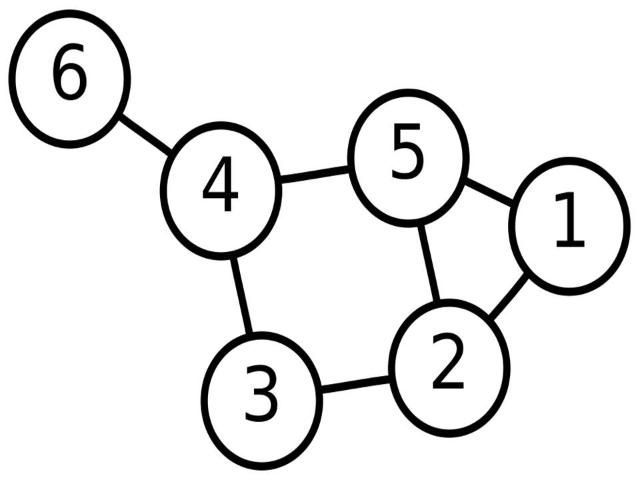
Credit:
Centralization of Network
A network is called a centralized network when the network is focused around one or a few actors. In the centralized network all individuals go to one or very few central actors for information and resources. For example, a community that has only one opinion leader would be considered a centralized network. Centralized networks are very vulnerable because the complete network would collapse if the central actor left (Kilduff & Tsai, 2003). In this case, a decentralized network, or one where there are multiple sources of information and resources are available, would be preferred as it would be more sustainable.
Clique
In social network research, a clique is a network in which all individuals have direct ties to all other individuals in the network with no existing external ties (Kilduff & Tsai, 2003). For example, in Figure 4, Actors 1, 2, and 5 form a clique where all three are connected to each other.
Structural Holes
A structural hole is a gap or lack of relationship between two individuals in the network who, if connected, would connect two different groups in the network. Individuals who are part of a network which is rich in structural holes can utilize their position in the network for their personal advantage by filling a gap as a broker (Burt, 1992). One example would be two Extension programs which were not connected in Florida Cooperative Extension. The lack of connection between these two programs is regarded as a structural hole, which represents an opportunity for collaboration and information sharing.
Social Capital
Within a network perspective, social capital can be classified into community social capital and network social capital. Community social capital "refers to features of social organization, such as trust, norms, and networks that can improve the efficiency of society by facilitating coordinated actions" (Putnam et al., 1993, p. 167). For example, a society which is rich in social capital has more resilience and the members of that society are more active toward the betterment of society. Network social capital refers to the existence of structural holes or brokerage opportunities where an individual actor in a network creates connections to improve their opportunities (Burt, 2000). See brokerage and structural holes above for examples.
Another perspective on social capital is that community social capital and social networks are interconnected, as a network which is dense, with many connections among actors in the network, also will have higher social capital. For example, in a community network of Extension stakeholders, all the stakeholders are well connected to each other, producing a dense network, and this density makes the community of Extension stakeholders rich in social capital. That means both social capital and social networks are interconnected concepts but still maintain their individual distinctions.
Strength of Ties
The strength of a tie can be defined as a "combination of the amount of time, the emotional intensity, the intimacy (mutual confiding), and the reciprocal services which characterize the tie" (Granovetter, 1973, p.1361). Strong ties are those which are sustainable and long lasting, such as ties of an individual to family members, whereas weak ties are distant and very infrequent (Granovetter, 1973), such as an Extension educator meeting an Extension specialist or another educator from a different state at a conference. Weak ties increase an actor's adaptive capacity to solve problems and his or her access to information and resources, and often are associated with actors who have higher brokerage capacities in a network.
Structural Equivalence
Structural equivalence refers to nodes occupying similar positions within a network—either a connection to similar nodes in the network, or nodes connected in the same pattern as other nodes in the network. For example, two Extension clients would be considered structurally equivalent when they occupy a similar position in the overall network, such as two clients who hold opinion leadership positions in the same community and are approached by the same number of community members seeking advice (Scott, 1988).
Homophily/Heterophily
Homophily is defined as the tendency for nodes to share attributes (for example ethnicity, gender, age, education) with other nodes. This concept is used to explain an actor's interaction, share of information, opinions, and behaviors with similar other actors in the network. Female Extension educators talking only to other female educators and male educators talking only to the other male educators are an example of homophily (Kilduff & Tsai, 2003). While homophily makes it easier for actors to communicate with each other, heterophily is characterized by weak ties, which increase access to different information sources and adaptive capacity.
Conclusion
Social networks play an important role in the functioning of society and have an important effect on the actions of an individual or organization. It is very important for Extension educators to understand the networks of their clientele in order to perform better and develop more impactful Extension programming for their target audiences. This document provides a brief description of network concepts and the terminology used in network studies. It is hoped that by understanding the network concepts discussed in this paper Extension educators may become comfortable utilizing the results of social network analysis research. This methodology uses visual elements (network maps) and statistical tools to describe and analyze the networks of Extension stakeholders. Further discussion about social network analysis and how to use it is presented in Introduction to Social Network Research: Application of Social Network Analysis in Extension (https://edis.ifas.ufl.edu/publication/WC196) and Introduction to Social Network Research: Brokerage Typology (https://edis.ifas.ufl.edu/publication/wc197). It is highly recommended that Extension professionals consider reading these publications to gain a deeper understanding of social networks and how they can utilize network analysis to improve the efficiency and productivity of Extension organizations.
References
Bonacich, P. (2007). Some unique properties of eigenvector centrality. Social Networks, 29, 555–564.
Borgatti, S. P. (2005). Centrality and network flow. Social Networks, 27, 55–71.
Borgatti, S. P., Everett, M. G., & Johnson, J. C. (2013). Analyzing social networks. London: Sage Publications.
Brass, D. J., & Burkhardt, M. E. (1992). Centrality and power in organizations. Networks and organizations: Structure, Form, and Action, 191, 215–230.
Brass, D. J., Galaskiewicz, J., Greve, H. R., & Tsai, W. (2004). Taking stock of networks and organizations: A multilevel perspective. Academy of management journal, 47(6), 795–817.
Burt, R. S. (1992). Structural Holes: The Social Structure of Competition. Cambridge, MA: Harvard University Press.
Burt, R. S. (2000). The network structure of social capital. Research in Organizational Behavior, 22, 345–423.
Freeman, L. C. (1979). Centrality in social networks conceptual clarification. Social Networks, 1(3), 215–239.
Gould, R. V., & Fernandez, R. M. (1989). Structures of mediation: A formal approach to brokerage in transaction networks. Sociological Methodology, 19, 89–126.
Granovetter, M. S. (1973). The strength of weak ties. American Journal of Sociology, 78(6), 1360–1380.
Kilduff, M., & Tsai, W. (2003). Social Networks and Organizations. Thousand Oaks, CA: Sage Publications.
Kumar Chaudhary, A. (2014). Diversity and reach of Penn State Extension programs and effect of brokerage and network position on extension program outcomes through Social Network Analysis (SNA). (Unpublished master's thesis.) The Pennsylvania State University, State College, PA.
McCarty, C. & Vacca, R. (2013). A social network analysis of scientific collaborations at UF. PowerPoint slides.
Provan, K. G., Fish, A., & Sydow, J. (2007). Interorganizational networks at the network level: A review of the empirical literature on whole networks. Journal of Management, 33(3), 479–516.
Putnam, R. D., Leonardi, R., & Nanetti, R.Y. (1993). Making Democracy Work: Civic Traditions in Modern Italy. Princeton, NJ: Princeton University Press.
Scott, J. (1988). Social network analysis. Sociology, 22(1), 109–127.
Stevenson, W. B., & Greenberg, D. (2000). Agency and social networks: Strategies of action in a social structure of position, opposition, and opportunity. Administrative Science Quarterly, 45(4), 651–678.
Stovel, K. & Shaw, L. (2013). Brokerage. Annual Review of Sociology, 38, 139–158.
Tsai, W. (2001). Knowledge transfer in intraorganizational networks: Effects of network position and absorptive capacity on business unit innovation and performance. Academy of Management Journal, 44(5), 996–1004.
Vera, E. R., & Schupp, T. (2006). Network analysis in comparative social sciences. Comparative Education, 42(3), 405–429.
Wasserman, S., & Faust, K. (1994). Social Network Analysis: Methods and applications. Cambridge: Cambridge University Press.
Wellman, B., & Berkowitz, S. D. (Eds.). (1988). Social structures: A network approach. Cambridge: Cambridge University Press.
Yin, X., Wu, J., & Tsai, W. (2012). When Unconnected Others Connect: Does Degree of Brokerage Persist After the Formation of a Multipartner Alliance? Organization Science, 23(6), 1682–1699.
Zack, M. H. (2000). Researching organizational systems using social network analysis. Proceedings of the 33rd Annual Hawaii International Conference on System Sciences. doi:10.1109/HICSS.2000.926933