Introduction
Surveys have remained useful and efficient tools for learning about the attitudes, opinions, and behaviors of the general public (Dillman, Smyth, & Christian, 2014; Salant & Dillman, 1994). Yet, what happens once a survey is distributed and the data has been collected? This fact sheet is one of three publications in the Savvy Survey Series that offer guidance on the steps that should be taken after implementing a survey.
As the Savvy Survey publications #10 (In-person-administered Surveys), #11 (Mail-based Surveys), #12 (Telephone Surveys), #13 (Online Surveys), #14 (Mixed-mode Surveys), and #18 (Group-administered Surveys) have stated, survey data can be collected using a variety of modes. The mode is chosen based on the best fit for the situation and purpose. Many modes (in-person, mail, mixed-mode) result in paper-based survey responses, while others (online, telephone, mixed-mode) may be designed to allow respondents to submit their answers directly into an electronic database.
This publication discusses the procedures for entering paper-based survey responses into an electronic database and preparing (or "cleaning") all data, regardless of mode, for analysis. Although scanning equipment is used by some organizations to record survey responses from specially formatted questionnaires into an electronic data file, this approach is not cost effective for the majority of small-scale surveys commonly used at the county or state level. Consequently, this fact sheet focuses on manual processes of data entry. This publication also addresses data entry considerations for closed-ended questions (with response choices), partially-closed questions, and open-ended questions, since the types of questions used in the survey will also impact the data entry process.
Dealing with Data
When working with paper-based survey responses, one process for data entry suggests following four steps (Groves et al., 2009):
- Collect data (e.g., retrieve surveys via mail, collection boxes, directly from survey participants)
- Code text-based data into a numeric format
- Enter the coded data into a computer program
- Review data for any mistakes made during the data entry step (see Figure 1)

Step 1. Collecting Data
When surveys were first used to capture responses from the public, the main modes for collecting the data were face-to- face interviewing and mail questionnaires (Groves, 2011). With mail questionnaires, respondents were asked to fill out the survey and mail it back to the organization delivering the survey. Mail-based surveys are still often used; however, this mode requires surveyors to wait until the question- naires are returned in the mail. Instead of waiting on the return of mail-based surveys, some situations (e.g., surveys at zoos, aquaria, amusement parks, demonstration gardens, public lands) use procedures asking visitors to complete the questionnaire in person. Often, these respondents are given the option to either return the completed questionnaires to the surveyors or to a centrally-located collection box.
With the onslaught of undesired phone solicitations and the emergence of non-geographically-connected cellular phone numbers, the use of telephone-based surveys has fallen out of favor (Dillman, Smyth, & Christian, 2014). However, when this mode proves to be the best option, data collection can vary in how the data is recorded. If the survey is being conducted by an interviewer, then that individual becomes responsible for recording the data, either on paper or directly into the computer, as well as by downloading an interview from an audio recording device. On the other hand, if the data is collected via interactive voice response (IVR) methods, the data is recorded along with the phone call solely by a computer.
Step 2. Code Text Data
Step 2.1 Code Quantitative Text Data
Even before the data has been collected, a savvy surveyor has often begun to consider what codes would be appropriate for responses to the questionnaire. Typically, questionnaires have response choices that are completely text-based. For example, responses to a closed-ended question may range from selected words in a scale (e.g., strongly disagree to strongly agree) or might be specific response words (e.g., male, female). Partially-closed questions give a list of specific items as well as an area to write in a response. For more information on constructing these various question types, refer to Savvy Survey Series #6c.
Coding questionnaire data means transforming responses into numeric values. Coding the data into numbers will help data analysis on a computer. Since usable data requires careful planning, coding decisions must be recorded and communicated to guarantee the procedure is consistent and reliable. If using a 5-point Likert scale, item choices such as "strongly disagree" would get a number value of "1" and would go up to "strongly agree" which would typically be assigned a "5" (as shown in Figure 2, Q1). Used for this response set, this 1-to-5 coding scheme has an associated value: the higher the score, the more strongly they agree. However, in the case of a categorical item, the number assigned has no "value" associated with it. Instead, the numeric code represents which category is being referred to (see Figure 2, Q2).
There are also situations in which a savvy surveyor may use a questionnaire with reverse coded items. This method is typically used to encourage respondents to think about each question carefully and select the proper response. Figure 3 provides an example of a survey item with reverse codes and a survey item with normal coding for comparison purposes. Question one in Figure 3 displays a negatively-worded item with a 5-point Likert scale response set where "strongly disagree" receives the number value of "5" and subsequently decreases to "strongly agree" which is assigned the value of "1" (which is the opposite of how most people think about the values of numbers and scales). Meanwhile, question two displays a positively-worded item with a 5-point Likert scale, where "strongly disagree" receives the number value of "1" and increases to "strongly agree" which is assigned the value of "5." When conducting analyses with negatively worded, reverse-coded items, it is important to confirm the appropriate codes are used prior to combining them with positively-worded items in a multi-item index. For more information on reverse scaling, see Savvy Survey #6d: Construction Indices for a Questionaire.
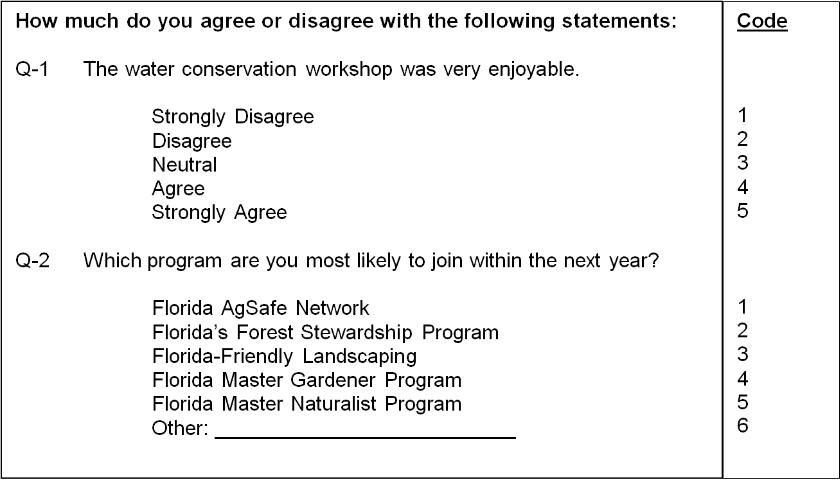
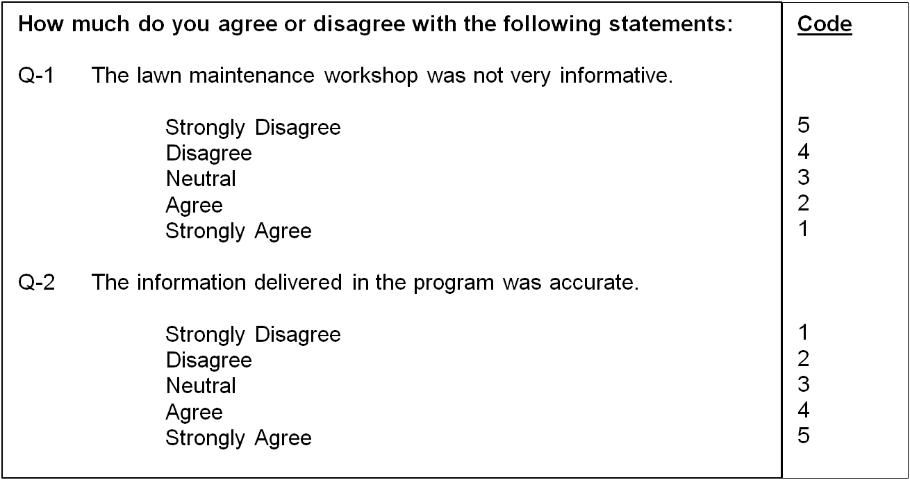
When coding surveys with quantitative responses, the following best practices should be used:
- Develop mutually exclusive and exhaustive categories for data entry. Mutually exclusive means that the categories do not overlap and a person's answer should fit into only one response category. Exhaustive means that all possible answers are covered by the response options.
- Match the number of variables in the data file to the number of items in the question. For example, a "check all that apply" question with five items should have a vari- able (or data entry field) for recording whether each item in the list is checked (this can be displayed as a horizontal cell for each person's response in a spreadsheet).
- It is also important to assign a unique ID number to each respondent. This allows each respondent to be identified in a study and is used when a study requires matching completed surveys for a specific person (e.g., a pre-test/ post-test research design or a longitudinal design). ID numbers are also helpful in matching paper surveys to responses in an electronic file to correct data entry errors (see below).
- When coding partially closed-ended questions, the set responses can be pre-coded. Coding numbers for "other" responses is also necessary for data analysis (see Figure 2, Q-2). However, additional codes can be created while cleaning the data using the responses provided in the open response area. This list can be used to see if there are enough answers to form additional meaningful categories for that item.
It may also be important to be able to tell the difference between responses such as "I don't know," "zero," "not applicable," and no response at all. Confusion about the meaning of answer categories can obscure the results. Users should assign code numbers to "I don't know" and "no reply" responses. In this instance, using codes such as 99 for "no response" and 88 for "don't know" or "no opinion" is a recommended practice (Salant & Dillman, 1994). However, it is important to remember to not include the 88s and 99s in the data analysis process since the high values can severely skew the results. This exclusion can be easily accomplished by indicating in the analysis program which values are missing from the data being analyzed.
Step 2.2 Coding Qualitative Text Data
Questionnaires can also include text-based responses in which a respondent writes out their entire response to a survey item. This type of question is referred to as an open-ended question. Open-ended questions, especially descriptive or explanatory types, have responses comprised of statements generated by the respondents rather than predetermined responses provided by the surveyor. The more open a response, the more creative the surveyor will have to be when entering the data and analyzing it.
There are several methods for coding qualitative data. One process that can be conducted by hand allows the research- er to peruse the text for important segments (Basit, 2003). When using this method for coding open-ended questions, the surveyor should read the relevant text to search for repeating ideas (same or similar words and phrases used to express the same idea across different participants) (Auerbach & Silverstein, 2003). In this setting, the surveyor is in search of the theme(s), or implicit topic(s), that organize(s) a group of repeating ideas.
Alternately, qualitative data analysis can be conducted via computer software, such as NVivo or MaxQDA. These applications assist users in organizing and analyzing non-numerical data. The software can be used to classify, sort, arrange, and explore relationships in the data (Basit, 2003). In-depth qualitative data, such as transcripts, can also be transferred to text-based computer software (i.e., Microsoft Word).
Once in the software, specific segments of the transcripts can be highlighted and separated into distinct folders for each theme (Basit, 2003).
Both the manual and electronic processes for coding qualitative data have pros and cons. For instance, manual coding requires a great deal of time to transcribe the data before any other process can be started. Furthermore, the longer it takes to code the data, the more costly the process becomes. Yet, it also allows the researchers to become familiar with the data and works well when using a small amount of qualitative data (e.g., 2–3 interviews in comparison to 15–20 interviews). It may take several weeks to become familiar with a software package to code qualitative data electronically (Basit, 2003), although computer software packages enable a researcher to code a great deal of qualitative data relatively smoothly, with the flexibility to code and un-code as well as highlight and un-highlight specific quotes. Ultimately, the choice of either manual or electronic coding is contingent upon the size of the project, the funds and time available, and the inclination and expertise of the researcher (Basit, 2003).
Step 3. Entering the Data
Step 3.1 Entering Quantitative Data
Once the design for coding the data is completed, it is time to enter the data into a computer for analysis. This task requires going through each questionnaire (one at a time) and typing the responses in order. For example, when entering data into Microsoft Excel, the spreadsheet has a series rows and columns. Each horizontal row contains responses from each questionnaire, including the ID number for each respondent. Each vertical column holds all of the responses to a specific question. As displayed in Figure 4, the first column contains the ID numbers (e.g., CCSID) for each respondent, the second column contains the code for the respondent's county, and columns 3-6 contain the responses for specific questionnaire items (e.g., Accurate, Deliver, Relevant, and Easy). In this instance, the first respondent is "20-151" where the county was "20" and this individual answered "5" for each of the questionnaire items.
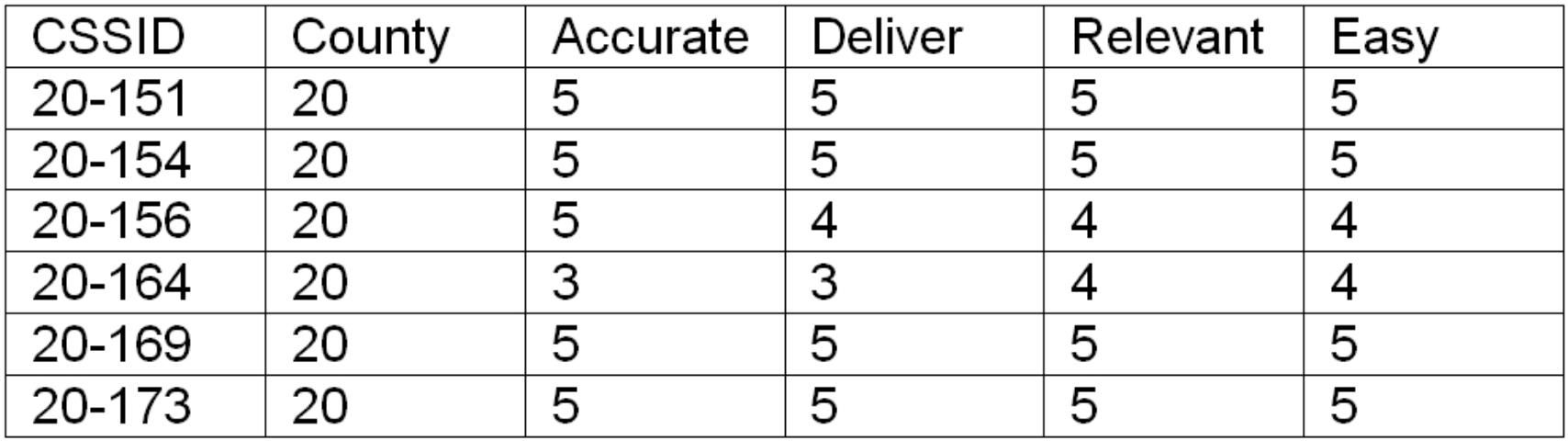
Step 3.2 Entering qualitative data
For qualitative data, the entry process will depend greatly on the coding process that was selected. For surveys with several open-ended questions, the answers can be typed verbatim into the appropriate data entry field (as in the case of an Excel file or Qualtrics survey). For more extensive responses from interviews, the data can be typed into separate text files (e.g., Microsoft Word document files) and saved for future use. The files can also be grouped into specific folders for further organization. For surveys that were conducted electronically, the transcripts can be either imported or typed into the software (e.g.,using NVivo or MaxQDA).
Another option to consider when a large number of questionnaires have been completed is the use of the Qualtrics survey software as a data entry tool. Although Qualtrics is typically used to create a survey, send it out to participants, and collect the responses, it can also be used to enter responses from a paper survey into an electronic file. The Qualtrics software then places the questionnaire data into a downloadable file format (e.g., Excel or SPSS file) for more in-depth data analysis. For instance, when using Excel for tabulating the data, a user can download an Excel file containing the survey responses from Qualtrics (with the data organized into a spreadsheet format). However, when using survey software for data entry, the program may assign default numeric codes during the initial survey construction. Therefore, a savvy surveyor should confirm that the codes are correct and consistent with the coding scheme previously created in Step 2 (e.g., 5 = strongly agree rather than 1 = strongly agree).
Step 4. Review Data for Mistakes
It is important to check the data for any mistakes before data analysis. Even the highly experienced surveyor can make data entry mistakes (e.g., enter responses twice or miskey the entry). Salant and Dillman (1994) suggest after entering data from all of questionnaires, one should re-enter the data into a second file and conduct basic calculations on both data sets. By comparing the results, inconsistencies can be helpful in identifying data entry errors. This method is called verifying or "cleaning" data, and is used frequently to avoid measurement error (when the data to be analyzed inaccurately reflects respondents' true attributes) due to incorrect data entry. When time and resources are insufficient for this double entry, the first step in data analysis should be to review the distribution of answers for each question to identify incorrect values (e.g., a 6 when the maximum code is 5) or unexpected answers.
In Summary
Data entry is an important step in preparing your data for analysis. Collecting data can be done using mail, telephone, and/or internet questionnaires. Coding data allows the collected information to be transformed into numeric values for data analysis (when using quantitative data) or to be broken down into smaller themes and grouped together (when using qualitative data). Entering data correctly either starts or continues the process of using the data on a computer. It also becomes critical to review the data to check for data entry errors and inconsistencies with the original responses.
References
Auerbach, C. F., & Silverstein, L. B. (2003). Qualitative data: An introduction to coding and analysis. New York, NY: New York University Press.
Basit, T. N. (2003). Manual or electronic? The role of coding in qualitative data analysis. Educational Research, 45(2), 143-154.
Dillman, D. A., Smyth, J. D., & Christian, L. M. (2014). Internet, phone, mail, and mixed-mode surveys: The tailored design method. (4th ed.). Hoboken, NJ: John Wiley and Sons.
Groves, R. M. (2011). Three eras of survey research. Public Opinion Quarterly, 75(5), 861-871.
Groves, R. M., Fowler Jr., F. J., Couper, M. P., Lepkowski, J. M., Singer, E., & Tourangeau, R. (2009). Survey methodology. (2nd ed.). Hoboken, NJ: John Wiley and Sons.
Salant, P., & Dillman, D. A. (1994). How to conduct your own survey: Leading professionals give you proven techniques for getting reliable results. New York, NY: John Wiley & Sons, Inc.