Strategically Selecting Behaviors That Impact the Problem: An Approach Drawn from Social Marketing
Abstract
Extension professionals and other practitioners address a wide variety of complex issues by providing education and encouraging behavior change using innovative strategies. The importance of prioritizing potential behaviors and selecting those with high expected impact cannot be overemphasized. However, behavior selection can be complicated because there are many solutions for any problem in a particular context. Using an approach drawn from social marketing to develop activities aimed at changing or maintaining people's behavior, Extension professionals and other practitioners can prioritize behaviors by mathematically calculating anticipated weights that will help focus efforts around key behaviors with the potential to make the greatest impact. This publication provides an overview of a process to collect and analyze the impact and the likelihood of adoption to help Extension professionals decide on behaviors for a campaign or intervention.
Introduction
Extension professionals and other practitioners have been using strategic interventions to promote behavior change, and social marketing is one promising approach (Costanzo et al., 1986; Lehman & Geller, 2004). Social marketing is an effective strategy for Extension and other practitioners because it focuses on identifying and addressing the real barriers to change among an audience (Monaghan & Monroe, 2013). Social marketing can be contrasted with strategies that make assumptions without involving the audience, such as in the information-deficit approach, where barriers are not strategically considered and lack of knowledge is assumed to be the reason for an audience's inaction or lack of support (Simis et al., 2016). Social marketing also provides one of the best ways to remain relevant to your Extension audience. To learn more about social marketing principles and tools, see other UF/IFAS EDIS publications on this topic, such as:
-
Improving Behavioral Outcomes in Extension Using the Tools of Community-Based Social Marketing (CBSM) (https://edis.ifas.ufl.edu/wc149),
-
Improving Extension Program Development Using Audience Segmentation (https://edis.ifas.ufl.edu/wc188),
-
Using Audience Commitment to Increase Behavior Changes in Sustainable Landscaping (https://edis.ifas.ufl.edu/wc154), and
-
Using Social Norms to Increase Behavior Change in Sustainable Landscaping (https://edis.ifas.ufl.edu/wc158).
Behavior selection is often the first step in developing an effective behavior change intervention when using a social marketing approach (McKenzie-Mohr & Schultz, 2014). Audience research specific to the benefits and barriers of discrete behaviors is needed to successfully change practices using social marketing. (For example, we need to know what farmers think about installing subirrigation in their fields in addition to how they feel about protecting water resources in general.)
This publication summarizes a process to weight and prioritize potential behaviors for an Extension or outreach behavior-change intervention. The process can be used to evaluate behaviors based on their potential impact, the level of adoption already in place, and the likelihood of adoption among those who are not currently doing the behavior (McKenzie-Mohr, 2011). Therefore, you will need three pieces of information: impact, current adoption levels, and likelihood (see Figure 1).
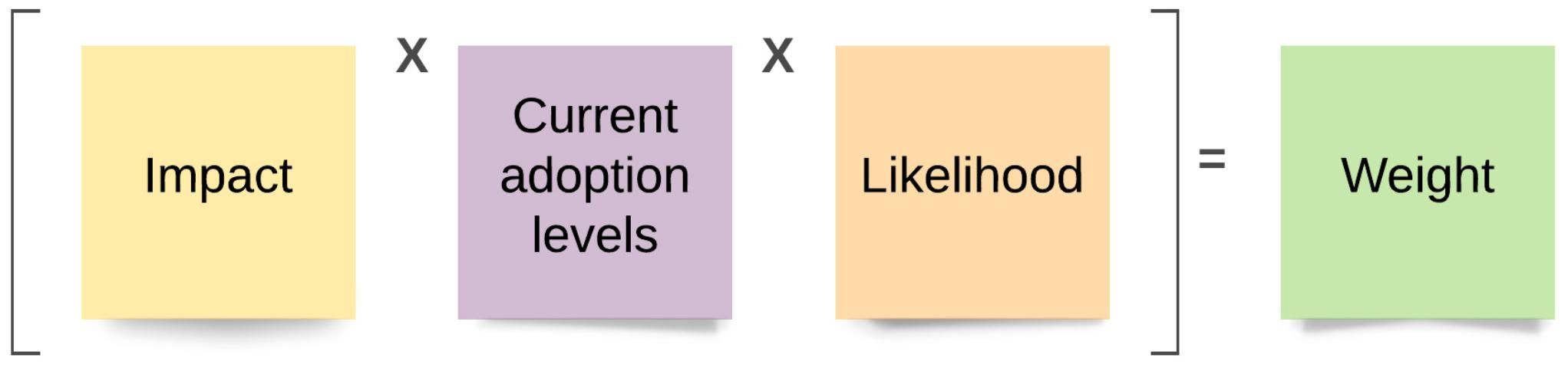
Behavior Selection in the Social Marketing Process
Potential behaviors should be selected based on their ability to address the problem you are working on. Your expertise comes into play here, as well as relevant research and others familiar with your area of focus. Some examples of potential behaviors include:
-
Preschools providing a piece of fruit each weekday (in place of less nutritious snacks) to preschool children in your county (Nutrition),
-
Homeowners installing weather stripping to gaps in windows (Sustainability), and
-
Farmers applying a more efficient fertilizer on tomato crops (Agriculture).
After you have defined a list of potential behaviors, you will want to identify those which warrant further consideration (McKenzie-Mohr, 2011). Are there any that fall outside your area of appointment or ability to address? Would any be unacceptable to your target audience? Would any be considered unreasonable requests due to financial, time, or other constraints?
We want to choose behaviors that are highly connected to the problem being addressed and will have a high impact on that problem, high likelihood of adoption, and low current adoption levels to have the greatest effect on the given problem (McKenzie-Mohr, 2011; McKenzie-Mohr & Schultz, 2014; Schultz, 2014).
-
Impact is the overall influence a behavior would make on the problem. Example: in the context of residential energy conservation, impact can be understood to mean the number of kilowatt-hours saved by each potential behavior.
-
Current adoption levels refer to how many people are already performing each potential behavior. There is data available on current adoption in some contexts. Example: in the context of residential water conservation, the proportion of households engaged in some of the most promising household-landscape water-conservation strategies has been reported at less than 20% at best in some locations in Florida (Warner, Momol et al., 2020), indicating substantial potential for further adoption. In cases where this type of data is not available, observation or audience surveys can be used to provide the needed information.
-
Likelihood is the probability that those who are not already performing a behavior will be willing to adopt it. Sometimes this information can be drawn from similar programs with the same or similar audience, although there is currently very limited research available on likelihood in many contexts. In cases where this type of data is not available, interviews or audience surveys can be used to provide the needed information.
Gathering the Required Information
Impact. The preferable option to establish impact, when possible, is to collect measurable data on each behavior's contribution to the problem (McKenzie-Mohr, 2011). For example, this could be the expected number of additional fruits and vegetables eaten per child, kilowatt-hours of electricity saved per household per year, or percent increase in profit per bushel of tomatoes sold, each respective to the particular behavior.
While this information is available as estimates for some behaviors (for example, residential landscape water conservation in Florida; Boyer & Dukes, 2015; Kumar Chaudhary et al., 2017), it is not available for all potential behaviors in every context. When the first option is infeasible, the second option is to independently survey 12–15 technical experts in the field of interest (McKenzie-Mohr et al., 2011). A simple survey administered to a panel of experts can be used. You might ask experts to rate behaviors with a question such as the following:
On a scale from 0 to 4, where 0 is no impact and 4 is the highest possible impact, please rate the potential impacts on water availability for the following behaviors.
Whether you measure impact as the percentage reduction in likelihood of financial savings per behavior, gallons of water saved per behavior, or a number from 0–4 indicating relative impact per behavior, what is important is that the units and scale are the same for all behaviors. Importantly, potential behaviors will not have the same impact across contexts (e.g., geographic location, specific audience, etc.). For this reason, it is ideal to obtain impact data from the context in which you are working or from experts familiar with the specific context.
Current adoption levels. Current adoption levels can be established by observing the target audience, when possible, or surveying the target audience to determine the current state of adoption (McKenzie-Mohr, 2011). If you choose to survey your audience, you might ask respondents to indicate their current adoption levels using yes and no responses. Or, you might ask them to indicate the frequency they engage in the behaviors of interest (on a scale from 5, never to 1, always), depending on your context. Depending on the approach, you can calculate either a percentage of people currently engaged in the behavior, or a mean frequency of engagement. Note: we want to code these data so lower current adoption levels (i.e., never or no) receive a greater value while higher current adoption levels (i.e., always or yes) receive a lower value, because lower levels of current adoption equate to higher potential effect on the problem.
Again, as with impact, it is critical to ensure the units and scale are the same for all behaviors.
Likelihood. Likelihood can be established by collecting case-study evaluation and behavior-change data from similar programs, when possible. Often this type of information is simply not available, in which case the alternative option is to interview or survey the target audience to determine their likelihood of adoption of each of the behaviors (McKenzie-Mohr, 2011). If you can access behavioral adoption data from similar programs, that is great. If you choose to survey your audience, you might ask people to tell you how unlikely or likely they are to engage in the specific behaviors in the future for each of the potential behaviors using response choices very unlikely, unlikely, neither unlikely nor likely, likely, and very likely.
As with impact and current adoption levels, it is critical to ensure the units and scale are the same for all behaviors.
Analyzing the Data
The three pieces of data for your list of behaviors can be analyzed using Microsoft Excel, a calculator, or paper and pencil.
Higher values of both impact and likelihood correspond to more desirable behaviors for targeted social marketing campaigns, so you can use the values of these measures as is. To calculate impact, take the mean of all responses. To calculate likelihood of adopting each of the behaviors you would assign a value to response (e.g., 1 for very unlikely to 5 for very likely) and then take the mean of all responses for each behavior.
As mentioned above, lower values of current adoption represent more desirable behaviors (indicating greater potential for adoption) so generally, the percentage of the audience not currently engaged (versus number of individuals engaged) will be used because fewer people engaged in the behavior indicates more potential people who could adopt as a result of your intervention. To calculate current adoption levels, be sure you have coded the people who have not adopted as a 1 and code those who have as a 0 or code frequency responses so lower frequency (e.g., never) receives the high values (e.g., 5).
To calculate the overall weight for each behavior, multiply impact by current adoption levels by likelihood. See Table 1 for a general representation of a table that could be used to calculate these values; a specific example follows.
For a ready-to-use behavior selection table with calculations prefilled, visit the Behavior Selection Calculator for Extension Professionals and Other Practitioners (Excel download).
Example
The following is a simple example of how three behaviors could be compared using impact, current adoption levels, and likelihood (see Table 2). The example was taken from a UF/IFAS project in a large residential community in northeast Florida (Warner, Martin et al., 2020). For this project, UF/IFAS worked with local government and university partners to conduct initial social marketing program planning activities. The goal was to identify promising behaviors for future social marketing campaigns to improve local water quality. Impact was collected using a panel of technical experts in both the local area and water quality who rated each behavior on a scale of 0 (no impact) to 4 (high impact). Current adoption levels were collected via a survey of neighborhood residents where respondents reported the frequency of engaging in each behavior on a scale of 1 (always) to 5 (never). Likelihood of engaging in the behaviors was collected via a survey of neighborhood residents where respondents reported their likelihood of engaging in the practice on a scale from 1 (very unlikely) to 5 (very likely). The project examined 16 unique behaviors, and for the purpose of simplicity we present three in the table below.
Notice that we might come to very different conclusions if we only considered one piece of information, such as likelihood. Looking at the overall weights, Behavior 1 would likely be the clear choice for our example program. Behavior 1 has lower likelihood of adoption among the residents, but this is offset by the experts' higher impact rating and lower current adoption levels. Notice Behaviors 2 and 3 have the same impact rating for addressing water quality as well as the same likelihood of adoption. However, fewer residents are engaged in Behavior 2, so it could potentially have a bigger impact on the problem. While people are somewhat less likely to adopt Behavior 1 (indicated by the low value under likelihood), the higher potential impact (indicated by the 3.42 under impact) and lower current adoption levels (denoted by the 2.91 under current adoption levels) led to a weight of 38.71, which would be worth considering as a priority behavior for our program.
How to Use This Information
Extension professionals and other practitioners are encouraged to utilize the concepts presented in this document in several ways:
-
Use the process described here to start a conversation about behavior selection during the program planning process.
-
Recognize that potential impacts, current adoption levels, and likelihood of adoption should be considered together in determining how effective a behavior could be.
-
Consider using the process described within to convey your decision-making process to stakeholders and support prioritization of a behavior.
-
Consider implementing this weighting process when developing logic models for program planning activities or funding requests.
-
Create program objectives that target change in the behaviors that have the greatest overall weight.
-
Design programs to focus on enhancing the adoption of the behaviors that have the greatest overall weight.
-
Refine evaluative efforts to measure adoption of the behaviors that have the greatest overall weight.
-
Consider expanding evaluative efforts beyond adoption to further assess the impact of the adoption under the adopter conditions. For example, does the program increase overall likelihood of adoption among others within the audience as they see their peers changing their behaviors?
-
When behaviors with high technical potential and low current adoption have low weights as a result of low likelihood of adoption, find out why the audience is unlikely or unwilling to adopt the behavior. Perceptions or barriers could be identified that could be addressed by a program.
-
Consider sharing new data on potential impact, current adoption levels, and likelihood of adoption. Some may experience challenges in applying the process described here in that there is a lack of existing data in many contexts, and there is an opportunity to help professionals and other practitioners by sharing this type of information.
Conclusion
We offer two notes of emphasis to the reader. First, low current adoption levels could indicate great potential for adoption or they could indicate something else, such as that there is overall resistance to the behavior, a lack of understanding of what the behavior involves, or even that the behavior could be unacceptable to target audience members. Additionally, when some of the behaviors being considered are similar, a person might need to give up a particular behavior before adopting something else, which would serve as a barrier to that behavior change. The approach described within this document does not factor these types of situations into the calculations, and more information may be needed. It is important to engage the audience as well as technical experts when planning programs so nuances such as these can be better understood.
Using an approach drawn from social marketing, Extension professionals and other practitioners can mathematically calculate anticipated weights of potential behaviors. This publication provided an overview of a process to collect and analyze potential impact, current adoption levels, and likelihood of adoption to make decisions about behavior selection for a campaign or intervention. Extension professionals and other practitioners are encouraged to use the process described in this document to make strategic programmatic decisions.
Acknowledgment
This work was supported by USDA National Institute of Food and Agriculture Hatch project 1018367.
References
Costanzo, M., Archer, D., Aronson, E., & Pettigrew, T. (1986). Energy conservation behavior: The difficult path from information to action. American Psychologist, 41(5), 521–528. https://doi.org/10.1037/0003-066X.41.5.521
Lehman, P. K., & Geller, E. S. (2004). Behavior analysis and environmental protection: Accomplishments and potential for more. Behavior and Social Issues, 13, 13–32. https://doi.org/10.5210/bsi.v13i1.33
McKenzie-Mohr, D. (2011). Fostering sustainable behavior (3rd ed.). Canada: New Society Publishers.
McKenzie-Mohr, D., & Schultz, P. W. (2014). Choosing effective behavior change tools. Social Marketing Quarterly, 20(1), 35-46. https://doi.org/10.1177/1524500413519257
Monaghan, P., & Monroe, M. (2013). Improving behavioral outcomes in extension using the tools of community-based social marketing (CBSM). AEC486. Gainesville: University of Florida Institute of Food and Agricultural Sciences. https://edis.ifas.ufl.edu/wc149
Monaghan, P., Warner, L., Telg, R., & Irani, T. (2014). Improving Extension program development using audience segmentation. AEC526. Gainesville: University of Florida Institute of Food and Agricultural Sciences. https://edis.ifas.ufl.edu/wc188
Simis, M. J., Madden, H., Cacciatore, M. A., & Yeo, S. K. (2016). The lure of rationality: Why does the deficit model persist in science communication? Public Understanding of Science, 25(4), 400–414. https://doi.org/10.1177/0963662516629749
Warner, L. A., Martin, M., Mitchell, J., & Reed, C. (2020, February). Selecting behaviors for your water outreach program: A case study from Volusia County, FL. Abstract presented at the 7th University of Florida Water Institute Symposium, Gainesville, FL.
Warner, L. A., Momol, E., Lewis, C., Wichman, T., Wilber, W., & Reisinger, A. J. (2020). Floridians' engagement in landscape best practices to protect water resources: Information from a 2018 survey. AEC682. Gainesville: University of Florida Institute of Food and Agricultural Sciences. https://edis.ifas.ufl.edu/wc345
Warner, L. A., & Monaghan, P. (2013). Using audience commitment to increase behavior changes in sustainable landscaping. AEC491. Gainesville: University of Florida Institute of Food and Agricultural Sciences. https://edis.ifas.ufl.edu/wc154
Warner, L., & Monaghan, P. (2014). Using social norms to increase behavior change in sustainable landscaping. AEC494. Gainesville: University of Florida Institute of Food and Agricultural Sciences. https://edis.ifas.ufl.edu/wc158